scFed: federated learning for cell type classification with scRNA-seq
- PMID: 38221903
- PMCID: PMC10788680
- DOI: 10.1093/bib/bbad507
scFed: federated learning for cell type classification with scRNA-seq
Abstract
The advent of single-cell RNA sequencing (scRNA-seq) has revolutionized our understanding of cellular heterogeneity and complexity in biological tissues. However, the nature of large, sparse scRNA-seq datasets and privacy regulations present challenges for efficient cell identification. Federated learning provides a solution, allowing efficient and private data use. Here, we introduce scFed, a unified federated learning framework that allows for benchmarking of four classification algorithms without violating data privacy, including single-cell-specific and general-purpose classifiers. We evaluated scFed using eight publicly available scRNA-seq datasets with diverse sizes, species and technologies, assessing its performance via intra-dataset and inter-dataset experimental setups. We find that scFed performs well on a variety of datasets with competitive accuracy to centralized models. Though Transformer-based model excels in centralized training, its performance slightly lags behind single-cell-specific model within the scFed framework, coupled with a notable time complexity concern. Our study not only helps select suitable cell identification methods but also highlights federated learning's potential for privacy-preserving, collaborative biomedical research.
Keywords: cell type; classification; federated learning; scRNA-seq.
© The Author(s) 2024. Published by Oxford University Press.
Figures
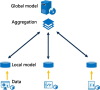
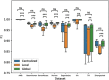
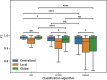
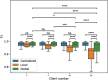
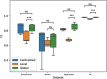
Similar articles
-
scEVOLVE: cell-type incremental annotation without forgetting for single-cell RNA-seq data.Brief Bioinform. 2024 Jan 22;25(2):bbae039. doi: 10.1093/bib/bbae039. Brief Bioinform. 2024. PMID: 38366803 Free PMC article.
-
Depressing time: Waiting, melancholia, and the psychoanalytic practice of care.In: Kirtsoglou E, Simpson B, editors. The Time of Anthropology: Studies of Contemporary Chronopolitics. Abingdon: Routledge; 2020. Chapter 5. In: Kirtsoglou E, Simpson B, editors. The Time of Anthropology: Studies of Contemporary Chronopolitics. Abingdon: Routledge; 2020. Chapter 5. PMID: 36137063 Free Books & Documents. Review.
-
scGCC: Graph Contrastive Clustering With Neighborhood Augmentations for scRNA-Seq Data Analysis.IEEE J Biomed Health Inform. 2023 Dec;27(12):6133-6143. doi: 10.1109/JBHI.2023.3319551. Epub 2023 Dec 5. IEEE J Biomed Health Inform. 2023. PMID: 37751336
-
scRGCL: a cell type annotation method for single-cell RNA-seq data using residual graph convolutional neural network with contrastive learning.Brief Bioinform. 2024 Nov 22;26(1):bbae662. doi: 10.1093/bib/bbae662. Brief Bioinform. 2024. PMID: 39708840 Free PMC article.
-
Interventions for providers to promote a patient-centred approach in clinical consultations.Cochrane Database Syst Rev. 2012 Dec 12;12(12):CD003267. doi: 10.1002/14651858.CD003267.pub2. Cochrane Database Syst Rev. 2012. PMID: 23235595 Free PMC article. Review.
Cited by
-
Knowledge-based inductive bias and domain adaptation for cell type annotation.Commun Biol. 2024 Nov 5;7(1):1440. doi: 10.1038/s42003-024-07171-9. Commun Biol. 2024. PMID: 39501016 Free PMC article.
-
AI Prediction of Structural Stability of Nanoproteins Based on Structures and Residue Properties by Mean Pooled Dual Graph Convolutional Network.Interdiscip Sci. 2024 Oct 5. doi: 10.1007/s12539-024-00662-7. Online ahead of print. Interdiscip Sci. 2024. PMID: 39367992
References
-
- Kiselev VY, Yiu A, Hemberg M. Scmap: projection of single-cell rna-seq data across data sets. Nat Methods 2018;15(5):359–62. - PubMed
-
- Ferguson D. A privacy concern: Bioinformatics and storing biodata. In The ADMI 2021 Symposium, 2021.