Tissue-adjusted pathway analysis of cancer (TPAC): A novel approach for quantifying tumor-specific gene set dysregulation relative to normal tissue
- PMID: 38206988
- PMCID: PMC10807770
- DOI: 10.1371/journal.pcbi.1011717
Tissue-adjusted pathway analysis of cancer (TPAC): A novel approach for quantifying tumor-specific gene set dysregulation relative to normal tissue
Abstract
We describe a novel single sample gene set testing method for cancer transcriptomics data named tissue-adjusted pathway analysis of cancer (TPAC). The TPAC method leverages information about the normal tissue-specificity of human genes to compute a robust multivariate distance score that quantifies gene set dysregulation in each profiled tumor. Because the null distribution of the TPAC scores has an accurate gamma approximation, both population and sample-level inference is supported. As we demonstrate through an analysis of gene expression data for 21 solid human cancers from The Cancer Genome Atlas (TCGA) and associated normal tissue expression data from the Human Protein Atlas (HPA), TPAC gene set scores are more strongly associated with patient prognosis than the scores generated by existing single sample gene set testing methods.
Copyright: © 2024 H. Robert Frost. This is an open access article distributed under the terms of the Creative Commons Attribution License, which permits unrestricted use, distribution, and reproduction in any medium, provided the original author and source are credited.
Conflict of interest statement
The authors have declared that no competing interests exist.
Figures
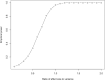
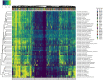
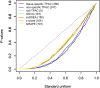
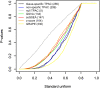
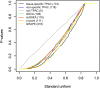
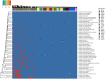
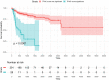
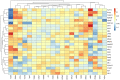
Similar articles
-
Variance-adjusted Mahalanobis (VAM): a fast and accurate method for cell-specific gene set scoring.Nucleic Acids Res. 2020 Sep 18;48(16):e94. doi: 10.1093/nar/gkaa582. Nucleic Acids Res. 2020. PMID: 32633778 Free PMC article.
-
GRAPE: a pathway template method to characterize tissue-specific functionality from gene expression profiles.BMC Bioinformatics. 2017 Jun 26;18(1):317. doi: 10.1186/s12859-017-1711-z. BMC Bioinformatics. 2017. PMID: 28651562 Free PMC article.
-
Analyzing cancer gene expression data through the lens of normal tissue-specificity.PLoS Comput Biol. 2021 Jun 18;17(6):e1009085. doi: 10.1371/journal.pcbi.1009085. eCollection 2021 Jun. PLoS Comput Biol. 2021. PMID: 34143767 Free PMC article.
-
Prognostic value of γ-aminobutyric acidergic synapse-associated signature for lower-grade gliomas.Front Immunol. 2022 Nov 3;13:983569. doi: 10.3389/fimmu.2022.983569. eCollection 2022. Front Immunol. 2022. PMID: 36405708 Free PMC article.
-
GS-TCGA: Gene Set-Based Analysis of The Cancer Genome Atlas.J Comput Biol. 2024 Mar;31(3):229-240. doi: 10.1089/cmb.2023.0278. Epub 2024 Mar 4. J Comput Biol. 2024. PMID: 38436570
References
MeSH terms
Grants and funding
LinkOut - more resources
Full Text Sources
Research Materials