High-Throughput Transcriptomics Differentiates Toxic versus Non-Toxic Chemical Exposures Using a Rat Liver Model
- PMID: 38139254
- PMCID: PMC10743995
- DOI: 10.3390/ijms242417425
High-Throughput Transcriptomics Differentiates Toxic versus Non-Toxic Chemical Exposures Using a Rat Liver Model
Erratum in
-
Correction: Pannala et al. High-Throughput Transcriptomics Differentiates Toxic versus Non-Toxic Chemical Exposures Using a Rat Liver Model. Int. J. Mol. Sci. 2023, 24, 17425.Int J Mol Sci. 2024 Jun 28;25(13):7108. doi: 10.3390/ijms25137108. Int J Mol Sci. 2024. PMID: 39000612 Free PMC article.
Abstract
To address the challenge of limited throughput with traditional toxicity testing, a newly developed high-throughput transcriptomics (HTT) platform, together with a 5-day in vivo rat model, offers an alternative approach to estimate chemical exposures and provide reasonable estimates of toxicological endpoints. This study contains an HTT analysis of 18 environmental chemicals with known liver toxicity. They were evaluated using male Sprague Dawley rats exposed to various concentrations daily for five consecutive days via oral gavage, with data collected on the sixth day. Here, we further explored the 5-day rat model to identify potential gene signatures that can differentiate between toxic and non-toxic liver responses and provide us with a potential histopathological endpoint of chemical exposure. We identified a distinct gene expression pattern that differentiated non-hepatotoxic compounds from hepatotoxic compounds in a dose-dependent manner, and an analysis of the significantly altered common genes indicated that toxic chemicals predominantly upregulated most of the genes and several pathways in amino acid and lipid metabolism. Finally, our liver injury module analysis revealed that several liver-toxic compounds showed similarities in the key injury phenotypes of cellular inflammation and proliferation, indicating potential molecular initiating processes that may lead to a specific end-stage liver disease.
Keywords: dose response; gene expression; high-throughput transcriptomics; histopathology; liver toxicity.
Conflict of interest statement
V.R.P. is employed by The Henry M. Jackson Foundation for the Advancement of Military Medicine, Inc. The remaining author declares that the research was conducted in the absence of any commercial or financial relationships that could be construed as potential conflicts of interest.
Figures
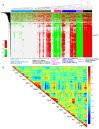
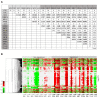
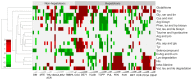
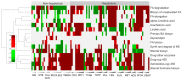
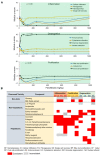
Similar articles
-
Evaluation of 5-day In Vivo Rat Liver and Kidney With High-throughput Transcriptomics for Estimating Benchmark Doses of Apical Outcomes.Toxicol Sci. 2020 Aug 1;176(2):343-354. doi: 10.1093/toxsci/kfaa081. Toxicol Sci. 2020. PMID: 32492150 Free PMC article.
-
NTP Research Report on the CLARITY-BPA Core Study: A Perinatal and Chronic Extended-Dose-Range Study of Bisphenol A in Rats: Research Report 9 [Internet].Research Triangle Park (NC): National Toxicology Program; 2018 Sep. Research Triangle Park (NC): National Toxicology Program; 2018 Sep. PMID: 31305969 Free Books & Documents. Review.
-
NTP technical report on the toxicology and carcinogenesis studies of 2,2',4,4',5,5'-hexachlorobiphenyl (PCB 153) (CAS No. 35065-27-1) in female Harlan Sprague-Dawley rats (Gavage studies).Natl Toxicol Program Tech Rep Ser. 2006 May;(529):4-168. Natl Toxicol Program Tech Rep Ser. 2006. PMID: 16835634
-
Safety and nutritional assessment of GM plants and derived food and feed: the role of animal feeding trials.Food Chem Toxicol. 2008 Mar;46 Suppl 1:S2-70. doi: 10.1016/j.fct.2008.02.008. Epub 2008 Feb 13. Food Chem Toxicol. 2008. PMID: 18328408 Review.
-
NTP toxicology and carcinogenesis studies of 3,3',4,4',5-pentachlorobiphenyl (PCB 126) (CAS No. 57465-28-8) in female Harlan Sprague-Dawley rats (Gavage Studies).Natl Toxicol Program Tech Rep Ser. 2006 Jan;(520):4-246. Natl Toxicol Program Tech Rep Ser. 2006. PMID: 16628245
Cited by
-
Correction: Pannala et al. High-Throughput Transcriptomics Differentiates Toxic versus Non-Toxic Chemical Exposures Using a Rat Liver Model. Int. J. Mol. Sci. 2023, 24, 17425.Int J Mol Sci. 2024 Jun 28;25(13):7108. doi: 10.3390/ijms25137108. Int J Mol Sci. 2024. PMID: 39000612 Free PMC article.
References
-
- Ramaiahgari S.C., Auerbach S.S., Saddler T.O., Rice J.R., Dunlap P.E., Sipes N.S., DeVito M.J., Shah R.R., Bushel P.R., Merrick B.A., et al. The Power of Resolution: Contextualized Understanding of Biological Responses to Liver Injury Chemicals Using High-throughput Transcriptomics and Benchmark Concentration Modeling. Toxicol. Sci. 2019;169:553–566. doi: 10.1093/toxsci/kfz065. - DOI - PMC - PubMed
-
- Spellman P.T., Sherlock G., Zhang M.Q., Iyer V.R., Anders K., Eisen M.B., Brown P.O., Botstein D., Futcher B. Comprehensive identification of cell cycle-regulated genes of the yeast Saccharomyces cerevisiae by microarray hybridization. Mol. Biol. Cell. 1998;9:3273–3297. doi: 10.1091/mbc.9.12.3273. - DOI - PMC - PubMed
MeSH terms
Grants and funding
LinkOut - more resources
Full Text Sources