Long non-coding RNA signature for predicting gastric cancer survival based on genomic instability
- PMID: 38127056
- PMCID: PMC10781445
- DOI: 10.18632/aging.205336
Long non-coding RNA signature for predicting gastric cancer survival based on genomic instability
Abstract
Background: Gastric cancer is a prevalent type of tumor with a poor prognosis. Given the high occurrence of genomic instability in gastric cancer, it is essential to investigate the prognostic significance of genes associated with genomic instability in this disease.
Methods: We identified genomic instability-related lncRNAs (GInLncRNAs) by analyzing somatic mutation and transcriptome profiles. We evaluated co-expression and enrichment using various analyses, including univariate COX analysis and LASSO regression. Based on these findings, we established an lncRNA signature associated with genomic instability, which we subsequently assessed for prognostic value, immune cell and checkpoint analysis, drug sensitivity, and external validation. Finally, PCR assay was used to verify the expression of key lncRNAs.
Results: Our study resulted in the establishment of a seven-lncRNA prognostic signature, including PTENP1-AS, LINC00163, RP11-169F17.1, C8ORF87, RP11-389G6.3, LINCO1210, and RP11-115H13.1. This signature exhibited independent prognostic value and was associated with specific immune cells and checkpoints in gastric cancer. Additionally, the model was correlated with somatic mutation and several chemotherapeutic drugs. We further confirmed the prognostic value of LINC00163, which was included in our model, in an independent dataset. Our model demonstrated superior performance compared to other models. PCR showed that LINC00163 was significantly up-regulated in 4 adjacent normal tissues compared with the GC tissues.
Conclusions: Our study resulted in the establishment of a seven-lncRNA signature associated with genomic instability, which demonstrated robust prognostic value in predicting the prognosis of gastric cancer. The signature also identified potential chemotherapeutic drugs, making it a valuable tool for clinical decision-making and medication use.
Keywords: gastric cancer; genomic instability; immune checkpoint; lncRNA; prognosis.
Conflict of interest statement
Figures
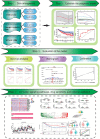
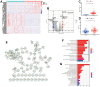
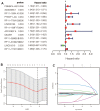
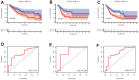
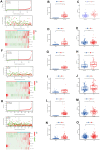
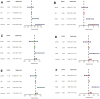
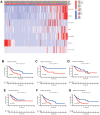
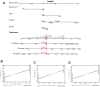
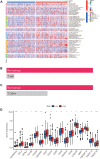
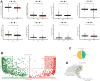
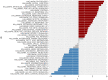
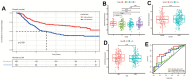
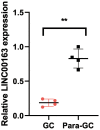
Similar articles
-
A somatic mutation-derived LncRNA signatures of genomic instability predicts the prognosis and tumor microenvironment immune characters in hepatocellular carcinoma.Hepatol Int. 2022 Oct;16(5):1220-1233. doi: 10.1007/s12072-022-10375-y. Epub 2022 Aug 10. Hepatol Int. 2022. PMID: 35947245
-
A genomic instability-related lncRNA model for predicting prognosis and immune checkpoint inhibitor efficacy in breast cancer.Front Immunol. 2022 Aug 5;13:929846. doi: 10.3389/fimmu.2022.929846. eCollection 2022. Front Immunol. 2022. PMID: 35990656 Free PMC article.
-
Identification and validation of a novel eight mutant-derived long non-coding RNAs signature as a prognostic biomarker for genome instability in low-grade glioma.Aging (Albany NY). 2021 Jun 3;13(11):15164-15192. doi: 10.18632/aging.203079. Epub 2021 Jun 3. Aging (Albany NY). 2021. PMID: 34081618 Free PMC article.
-
Prediction of bladder cancer outcome by identifying and validating a mutation-derived genomic instability-associated long noncoding RNA (lncRNA) signature.Bioengineered. 2021 Dec;12(1):1725-1738. doi: 10.1080/21655979.2021.1924555. Bioengineered. 2021. PMID: 33955803 Free PMC article.
-
A novel copper-induced cell death-related lncRNA prognostic signature associated with immune infiltration and clinical value in gastric cancer.J Cancer Res Clin Oncol. 2023 Sep;149(12):10543-10559. doi: 10.1007/s00432-023-04916-7. Epub 2023 Jun 8. J Cancer Res Clin Oncol. 2023. PMID: 37291405 Free PMC article.
Cited by
-
Screening the active ingredients of plants via molecular docking technology and evaluating their ability to reduce skin photoaging.Biogerontology. 2024 Nov;25(6):1115-1143. doi: 10.1007/s10522-024-10125-7. Epub 2024 Sep 23. Biogerontology. 2024. PMID: 39312047
-
Analysis of multiple factors influencing the survival of patients with advanced gastric cancer.Aging (Albany NY). 2024 May 13;16(10):8541-8551. doi: 10.18632/aging.205820. Epub 2024 May 13. Aging (Albany NY). 2024. PMID: 38742950 Free PMC article.
References
-
- Bailey P, Chang DK, Nones K, Johns AL, Patch AM, Gingras MC, Miller DK, Christ AN, Bruxner TJ, Quinn MC, Nourse C, Murtaugh LC, Harliwong I, et al., and Australian Pancreatic Cancer Genome Initiative. Genomic analyses identify molecular subtypes of pancreatic cancer. Nature. 2016; 531:47–52. 10.1038/nature16965 - DOI - PubMed
Publication types
MeSH terms
Substances
LinkOut - more resources
Full Text Sources
Medical
Research Materials
Miscellaneous