Brewpitopes: a pipeline to refine B-cell epitope predictions during public health emergencies
- PMID: 38124749
- PMCID: PMC10730938
- DOI: 10.3389/fimmu.2023.1278534
Brewpitopes: a pipeline to refine B-cell epitope predictions during public health emergencies
Abstract
The application of B-cell epitope identification to develop therapeutic antibodies and vaccine candidates is well established. However, the validation of epitopes is time-consuming and resource-intensive. To alleviate this, in recent years, multiple computational predictors have been developed in the immunoinformatics community. Brewpitopes is a pipeline that curates bioinformatic B-cell epitope predictions obtained by integrating different state-of-the-art tools. We used additional computational predictors to account for subcellular location, glycosylation status, and surface accessibility of the predicted epitopes. The implementation of these sets of rational filters optimizes in vivo antibody recognition properties of the candidate epitopes. To validate Brewpitopes, we performed a proteome-wide analysis of SARS-CoV-2 with a particular focus on S protein and its variants of concern. In the S protein, we obtained a fivefold enrichment in terms of predicted neutralization versus the epitopes identified by individual tools. We analyzed epitope landscape changes caused by mutations in the S protein of new viral variants that were linked to observed immune escape evidence in specific strains. In addition, we identified a set of epitopes with neutralizing potential in four SARS-CoV-2 proteins (R1AB, R1A, AP3A, and ORF9C). These epitopes and antigenic proteins are conserved targets for viral neutralization studies. In summary, Brewpitopes is a powerful pipeline that refines B-cell epitope bioinformatic predictions during public health emergencies in a high-throughput capacity to facilitate the optimization of experimental validation of therapeutic antibodies and candidate vaccines.
Keywords: antibody therapeutics; bioinformatics and computational biology; epitope prediction and antigenicity prediction; immunology and infectious diseases; vaccine development.
Copyright © 2023 Farriol-Duran, López-Aladid, Porta-Pardo, Torres and Fernández-Barat.
Conflict of interest statement
The authors declare that the research was conducted in the absence of any commercial or financial relationships that could be construed as a potential conflict of interest.
Figures
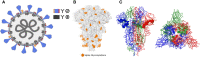
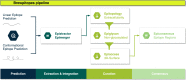
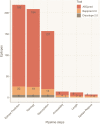
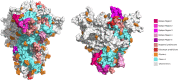
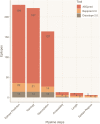
Similar articles
-
Bioinformatics-based SARS-CoV-2 epitopes design and the impact of spike protein mutants on epitope humoral immunities.Immunobiology. 2022 Nov;227(6):152287. doi: 10.1016/j.imbio.2022.152287. Epub 2022 Sep 28. Immunobiology. 2022. PMID: 36244092 Free PMC article.
-
Bioinformatics analysis of epitope-based vaccine design against the novel SARS-CoV-2.Infect Dis Poverty. 2020 Jul 10;9(1):88. doi: 10.1186/s40249-020-00713-3. Infect Dis Poverty. 2020. PMID: 32741372 Free PMC article.
-
Revelation of Potent Epitopes Present in Unannotated ORF Antigens of SARS-CoV-2 for Epitope-Based Polyvalent Vaccine Design Using Immunoinformatics Approach.Front Immunol. 2021 Aug 23;12:692937. doi: 10.3389/fimmu.2021.692937. eCollection 2021. Front Immunol. 2021. PMID: 34497604 Free PMC article.
-
Identification of B-Cell Epitopes for Eliciting Neutralizing Antibodies against the SARS-CoV-2 Spike Protein through Bioinformatics and Monoclonal Antibody Targeting.Int J Mol Sci. 2022 Apr 14;23(8):4341. doi: 10.3390/ijms23084341. Int J Mol Sci. 2022. PMID: 35457159 Free PMC article. Review.
-
Immunoinformatics Approach for the Identification and Characterization of T Cell and B Cell Epitopes towards the Peptide-Based Vaccine against SARS-CoV-2.Arch Med Res. 2021 May;52(4):362-370. doi: 10.1016/j.arcmed.2021.01.004. Epub 2021 Jan 29. Arch Med Res. 2021. PMID: 33546870 Free PMC article. Review.
References
-
- CoVariants. Available at: https://covariants.org/.
Publication types
MeSH terms
Substances
Grants and funding
LinkOut - more resources
Full Text Sources
Miscellaneous