AMSF: attention-based multi-view slice fusion for early diagnosis of Alzheimer's disease
- PMID: 38077590
- PMCID: PMC10703093
- DOI: 10.7717/peerj-cs.1706
AMSF: attention-based multi-view slice fusion for early diagnosis of Alzheimer's disease
Abstract
Alzheimer's disease (AD) is an irreversible neurodegenerative disease with a high prevalence in the elderly population over 65 years of age. Intervention in the early stages of AD is of great significance to alleviate the symptoms. Recent advances in deep learning have shown extreme advantages in computer-aided diagnosis of AD. However, most studies only focus on extracting features from slices in specific directions or whole brain images, ignoring the complementarity between features from different angles. To overcome the above problem, attention-based multi-view slice fusion (AMSF) is proposed for accurate early diagnosis of AD. It adopts the fusion of three-dimensional (3D) global features with multi-view 2D slice features by using an attention mechanism to guide the fusion of slice features for each view, to generate a comprehensive representation of the MRI images for classification. The experiments on the public dataset demonstrate that AMSF achieves 94.3% accuracy with 1.6-7.1% higher than other previous promising methods. It indicates that the better solution for AD early diagnosis depends not only on the large scale of the dataset but also on the organic combination of feature construction strategy and deep neural networks.
Keywords: Alzheimer’s disease; Attention mechanism; Magnetic resonance imaging; Multi-view slice fusion.
© 2023 Zhang et al.
Conflict of interest statement
The authors declare that they have no competing interests.
Figures
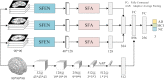
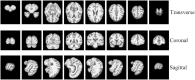
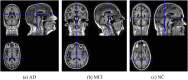
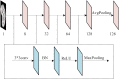
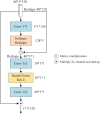
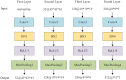
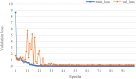
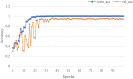
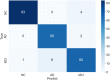
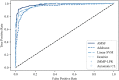
Similar articles
-
A multi-slice attention fusion and multi-view personalized fusion lightweight network for Alzheimer's disease diagnosis.BMC Med Imaging. 2024 Sep 27;24(1):258. doi: 10.1186/s12880-024-01429-8. BMC Med Imaging. 2024. PMID: 39333903 Free PMC article.
-
Alzheimer's Disease Diagnosis With Brain Structural MRI Using Multiview-Slice Attention and 3D Convolution Neural Network.Front Aging Neurosci. 2022 Apr 26;14:871706. doi: 10.3389/fnagi.2022.871706. eCollection 2022. Front Aging Neurosci. 2022. PMID: 35557839 Free PMC article.
-
A Fusion of Multi-view 2D and 3D Convolution Neural Network based MRI for Alzheimer's Disease Diagnosis.Annu Int Conf IEEE Eng Med Biol Soc. 2021 Nov;2021:3317-3321. doi: 10.1109/EMBC46164.2021.9629923. Annu Int Conf IEEE Eng Med Biol Soc. 2021. PMID: 34891950
-
Diagnosis of Alzheimer's disease based on regional attention with sMRI gray matter slices.J Neurosci Methods. 2022 Jan 1;365:109376. doi: 10.1016/j.jneumeth.2021.109376. Epub 2021 Oct 8. J Neurosci Methods. 2022. PMID: 34627926
-
Attention-guided neural network for early dementia detection using MRS data.Comput Med Imaging Graph. 2022 Jul;99:102074. doi: 10.1016/j.compmedimag.2022.102074. Epub 2022 May 27. Comput Med Imaging Graph. 2022. PMID: 35728368 Review.
References
-
- Barthelemy NR, Li Y, Joseph-Mathurin N, Gordon BA, Hassenstab J, Benzinger TLS, Buckles V, Fagan AM, Perrin RJ, Goate AM, Morris JC, Karch CM, Xiong C, Allegri R, Mendez PC, Berman SB, Ikeuchi T, Mori H, Shimada H, Shoji M, Suzuki K, Noble J, Farlow M, Chhatwal J, Graff-Radford NR, Salloway S, Schofield PR, Masters CL, Martins RN, O’Connor A, Fox NC, Levin J, Jucker M, Gabelle A, Lehmann S, Sato C, Bateman RJ, McDade E, Dominantly Inherited Alzheimer Network A soluble phosphorylated tau signature links tau, amyloid and the evolution of stages of dominantly inherited Alzheimer’s disease. Nature Medicine. 2020;26(3):398–407. doi: 10.1038/s41591-020-0781-z. - DOI - PMC - PubMed
-
- Billones CD, Demetria OJLD, Hostallero DED, Naval PC. DemNet: a convolutional neural network for the detection of Alzheimer’s disease and mild cognitive impairment. 2016 IEEE Region 10 Conference (TENCON); Piscataway: IEEE; 2016. pp. 3724–3727.
-
- Buyrukoğlu S. Improvement of machine learning models’ performances based on ensemble learning for the detection of Alzheimer disease. 2021 6th International Conference on Computer Science and Engineering (UBMK); Piscataway: IEEE; 2021. pp. 102–106.
-
- Cheng D, Liu M, Fu J, Wang Y. Classification of MR brain images by combination of multi-CNNs for AD diagnosis. Ninth international Conference on Digital Image Processing (ICDIP 2017): SPIE; Piscataway: IEEE; 2017. pp. 875–879.
Grants and funding
LinkOut - more resources
Full Text Sources