GENERALIST: A latent space based generative model for protein sequence families
- PMID: 38011273
- PMCID: PMC10703406
- DOI: 10.1371/journal.pcbi.1011655
GENERALIST: A latent space based generative model for protein sequence families
Abstract
Generative models of protein sequence families are an important tool in the repertoire of protein scientists and engineers alike. However, state-of-the-art generative approaches face inference, accuracy, and overfitting- related obstacles when modeling moderately sized to large proteins and/or protein families with low sequence coverage. Here, we present a simple to learn, tunable, and accurate generative model, GENERALIST: GENERAtive nonLInear tenSor-factorizaTion for protein sequences. GENERALIST accurately captures several high order summary statistics of amino acid covariation. GENERALIST also predicts conservative local optimal sequences which are likely to fold in stable 3D structure. Importantly, unlike current methods, the density of sequences in GENERALIST-modeled sequence ensembles closely resembles the corresponding natural ensembles. Finally, GENERALIST embeds protein sequences in an informative latent space. GENERALIST will be an important tool to study protein sequence variability.
Copyright: © 2023 Akl et al. This is an open access article distributed under the terms of the Creative Commons Attribution License, which permits unrestricted use, distribution, and reproduction in any medium, provided the original author and source are credited.
Conflict of interest statement
The authors have declared that no competing interests exist.
Figures
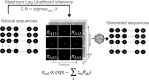
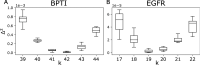
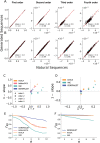
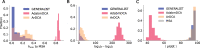
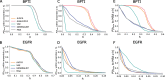
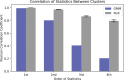
Similar articles
-
Accurate prediction for atomic-level protein design and its application in diversifying the near-optimal sequence space.Proteins. 2009 May 15;75(3):682-705. doi: 10.1002/prot.22280. Proteins. 2009. PMID: 19003998
-
Generative power of a protein language model trained on multiple sequence alignments.Elife. 2023 Feb 3;12:e79854. doi: 10.7554/eLife.79854. Elife. 2023. PMID: 36734516 Free PMC article.
-
Navigating the amino acid sequence space between functional proteins using a deep learning framework.PeerJ Comput Sci. 2021 Sep 17;7:e684. doi: 10.7717/peerj-cs.684. eCollection 2021. PeerJ Comput Sci. 2021. PMID: 34616884 Free PMC article.
-
General strategies for using amino acid sequence data to guide biochemical investigation of protein function.Biochem Soc Trans. 2022 Dec 16;50(6):1847-1858. doi: 10.1042/BST20220849. Biochem Soc Trans. 2022. PMID: 36416676 Free PMC article. Review.
-
Deep generative modeling for protein design.Curr Opin Struct Biol. 2022 Feb;72:226-236. doi: 10.1016/j.sbi.2021.11.008. Epub 2021 Dec 25. Curr Opin Struct Biol. 2022. PMID: 34963082 Review.
Cited by
-
Efficient Hi-C inversion facilitates chromatin folding mechanism discovery and structure prediction.bioRxiv [Preprint]. 2023 Jul 21:2023.03.17.533194. doi: 10.1101/2023.03.17.533194. bioRxiv. 2023. Update in: Biophys J. 2023 Sep 5;122(17):3425-3438. doi: 10.1016/j.bpj.2023.07.017 PMID: 36993500 Free PMC article. Updated. Preprint.
-
Efficient Hi-C inversion facilitates chromatin folding mechanism discovery and structure prediction.Biophys J. 2023 Sep 5;122(17):3425-3438. doi: 10.1016/j.bpj.2023.07.017. Epub 2023 Jul 26. Biophys J. 2023. PMID: 37496267 Free PMC article.
References
MeSH terms
Substances
Grants and funding
LinkOut - more resources
Full Text Sources