Characterization of cancer-associated fibroblasts (CAFs) and development of a CAF-based risk model for triple-negative breast cancer
- PMID: 38007443
- PMCID: PMC10676599
- DOI: 10.1186/s12935-023-03152-w
Characterization of cancer-associated fibroblasts (CAFs) and development of a CAF-based risk model for triple-negative breast cancer
Abstract
Triple-negative breast Cancer (TNBC) is a highly malignant cancer with unclear pathogenesis. Within the tumor microenvironment (TME), cancer-associated fibroblasts (CAFs) vitally influence tumor onset and progression. Thus, this research aimed to identify distinct subgroups of CAF using single-cell and TNBC-related information from the GEO and TCGA databases, respectively. The primary aim was to establish a novel predictive model based on the CAF features and their clinical relevance. Moreover, the CAFs were analyzed for their immune characteristics, response to immunotherapy, and sensitivity to different drugs. The developed predictive model demonstrated significant effectiveness in determining the prognosis of patients with TNBC, TME, and the immune landscape of the tumor. Of note, the expression of GPR34 was significantly higher in TNBC tissues compared to that in other breast cancer (non-TNBC) tissues, indicating that GPR34 plays a crucial role in the onset and progression of TNBC. In summary, this research has yielded a novel predictive model for TNBC that holds promise for the accurate prediction of prognosis and response to immunotherapy in patients with TNBC.
Keywords: Cancer-associated fibroblast; GPR34; Prognosis; Triple-negative breast cancer; Tumor microenvironment.
© 2023. The Author(s).
Conflict of interest statement
The authors declare that they have no competing interests.
Figures
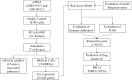
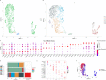
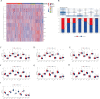
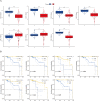
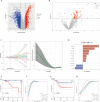
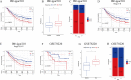
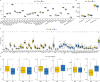
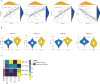
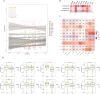
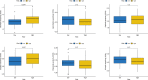
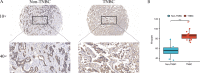
Similar articles
-
Landscape of cancer-associated fibroblasts identifies the secreted biglycan as a protumor and immunosuppressive factor in triple-negative breast cancer.Oncoimmunology. 2022 Jan 3;11(1):2020984. doi: 10.1080/2162402X.2021.2020984. eCollection 2022. Oncoimmunology. 2022. PMID: 35003899 Free PMC article.
-
Turning up a new pattern: Identification of cancer-associated fibroblast-related clusters in TNBC.Front Immunol. 2022 Oct 6;13:1022147. doi: 10.3389/fimmu.2022.1022147. eCollection 2022. Front Immunol. 2022. PMID: 36275659 Free PMC article.
-
Establishment of a tumor-associated fibroblast associated gene score based on scRNA-seq to predict prognosis in patients with triple-negative breast cancer.PLoS One. 2024 Oct 17;19(10):e0311801. doi: 10.1371/journal.pone.0311801. eCollection 2024. PLoS One. 2024. PMID: 39418248 Free PMC article.
-
CAF-Associated Genes in Breast Cancer for Novel Therapeutic Strategies.Biomedicines. 2024 Aug 29;12(9):1964. doi: 10.3390/biomedicines12091964. Biomedicines. 2024. PMID: 39335478 Free PMC article. Review.
-
Cancer-associated fibroblasts: The chief architect in the tumor microenvironment.Front Cell Dev Biol. 2023 Jan 30;11:1089068. doi: 10.3389/fcell.2023.1089068. eCollection 2023. Front Cell Dev Biol. 2023. PMID: 36793444 Free PMC article. Review.
Cited by
-
Breast Cancer Stem Cells Upregulate IRF6 in Stromal Fibroblasts to Induce Stromagenesis.Cells. 2024 Aug 31;13(17):1466. doi: 10.3390/cells13171466. Cells. 2024. PMID: 39273037 Free PMC article.
-
Identification of cuproptosis-related miRNAs in triple-negative breast cancer and analysis of the miRNA-mRNA regulatory network.Heliyon. 2024 Mar 27;10(7):e28242. doi: 10.1016/j.heliyon.2024.e28242. eCollection 2024 Apr 15. Heliyon. 2024. PMID: 38601669 Free PMC article.
References
-
- Zuo C, Baer JM, Knolhoff BL, Belle JI, Liu X, Alarcon De La Lastra A, Fu C, Hogg GD, Kingston NL, Breden MA, et al. Stromal and therapy-induced macrophage proliferation promotes PDAC progression and susceptibility to innate immunotherapy. J Exp Med. 2023 doi: 10.1084/jem.20212062. - DOI - PMC - PubMed
LinkOut - more resources
Full Text Sources