A novel efficient drug repurposing framework through drug-disease association data integration using convolutional neural networks
- PMID: 37993777
- PMCID: PMC10664633
- DOI: 10.1186/s12859-023-05572-x
A novel efficient drug repurposing framework through drug-disease association data integration using convolutional neural networks
Abstract
Drug repurposing is an exciting field of research toward recognizing a new FDA-approved drug target for the treatment of a specific disease. It has received extensive attention regarding the tedious, time-consuming, and highly expensive procedure with a high risk of failure of new drug discovery. Data-driven approaches are an important class of methods that have been introduced for identifying a candidate drug against a target disease. In the present study, a model is proposed illustrating the integration of drug-disease association data for drug repurposing using a deep neural network. The model, so-called IDDI-DNN, primarily constructs similarity matrices for drug-related properties (three matrices), disease-related properties (two matrices), and drug-disease associations (one matrix). Then, these matrices are integrated into a unique matrix through a two-step procedure benefiting from the similarity network fusion method. The model uses a constructed matrix for the prediction of novel and unknown drug-disease associations through a convolutional neural network. The proposed model was evaluated comparatively using two different datasets including the gold standard dataset and DNdataset. Comparing the results of evaluations indicates that IDDI-DNN outperforms other state-of-the-art methods concerning prediction accuracy.
Keywords: Data integration; Deep learning; Drug repurposing; Machine learning.
© 2023. The Author(s).
Conflict of interest statement
The authors declare that they have no competing interests.
Figures
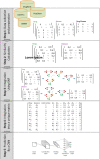
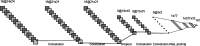
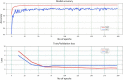
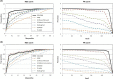
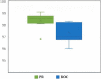
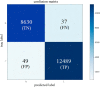
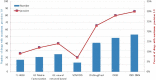
Similar articles
-
SNF-NN: computational method to predict drug-disease interactions using similarity network fusion and neural networks.BMC Bioinformatics. 2021 Jan 22;22(1):28. doi: 10.1186/s12859-020-03950-3. BMC Bioinformatics. 2021. PMID: 33482713 Free PMC article.
-
Predicting drug-target interaction network using deep learning model.Comput Biol Chem. 2019 Jun;80:90-101. doi: 10.1016/j.compbiolchem.2019.03.016. Epub 2019 Mar 25. Comput Biol Chem. 2019. PMID: 30939415
-
A comparative analysis of computational drug repurposing approaches: proposing a novel tensor-matrix-tensor factorization method.Mol Divers. 2024 Aug;28(4):2177-2196. doi: 10.1007/s11030-024-10851-7. Epub 2024 Apr 29. Mol Divers. 2024. PMID: 38683487
-
Data Integration Using Advances in Machine Learning in Drug Discovery and Molecular Biology.Methods Mol Biol. 2021;2190:167-184. doi: 10.1007/978-1-0716-0826-5_7. Methods Mol Biol. 2021. PMID: 32804365 Review.
-
A comprehensive review of artificial intelligence and network based approaches to drug repurposing in Covid-19.Biomed Pharmacother. 2022 Sep;153:113350. doi: 10.1016/j.biopha.2022.113350. Epub 2022 Jun 28. Biomed Pharmacother. 2022. PMID: 35777222 Free PMC article. Review.
References
-
- Parvizpour S et al. In silico targeting breast cancer biomarkers by applying rambutan (Nepheliumlappaceum) phytocompounds. J Biomol Struct Dyn. 2022;1–14 - PubMed
MeSH terms
LinkOut - more resources
Full Text Sources