Large-scale pancreatic cancer detection via non-contrast CT and deep learning
- PMID: 37985692
- PMCID: PMC10719100
- DOI: 10.1038/s41591-023-02640-w
Large-scale pancreatic cancer detection via non-contrast CT and deep learning
Abstract
Pancreatic ductal adenocarcinoma (PDAC), the most deadly solid malignancy, is typically detected late and at an inoperable stage. Early or incidental detection is associated with prolonged survival, but screening asymptomatic individuals for PDAC using a single test remains unfeasible due to the low prevalence and potential harms of false positives. Non-contrast computed tomography (CT), routinely performed for clinical indications, offers the potential for large-scale screening, however, identification of PDAC using non-contrast CT has long been considered impossible. Here, we develop a deep learning approach, pancreatic cancer detection with artificial intelligence (PANDA), that can detect and classify pancreatic lesions with high accuracy via non-contrast CT. PANDA is trained on a dataset of 3,208 patients from a single center. PANDA achieves an area under the receiver operating characteristic curve (AUC) of 0.986-0.996 for lesion detection in a multicenter validation involving 6,239 patients across 10 centers, outperforms the mean radiologist performance by 34.1% in sensitivity and 6.3% in specificity for PDAC identification, and achieves a sensitivity of 92.9% and specificity of 99.9% for lesion detection in a real-world multi-scenario validation consisting of 20,530 consecutive patients. Notably, PANDA utilized with non-contrast CT shows non-inferiority to radiology reports (using contrast-enhanced CT) in the differentiation of common pancreatic lesion subtypes. PANDA could potentially serve as a new tool for large-scale pancreatic cancer screening.
© 2023. The Author(s).
Conflict of interest statement
Alibaba group has filed for patent protection (application numbers: CN 202210575258.9, US 18046405) on behalf of Y.X., L.Z., J.Y., L. Lu and X. Hua for the work related to the methods of detection of pancreatic cancer in non-contrast CT. Y.X., J.Y., W.G., Y.W., W.F., M.Q., L. Lu and L.Z. are employees of Alibaba Group and own Alibaba stock as part of the standard compensation package. All other authors have no competing interests.
Figures
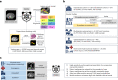
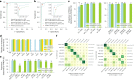
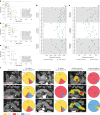
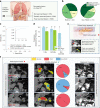
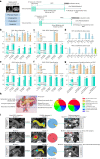
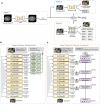
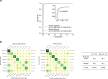
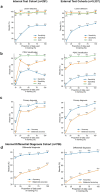
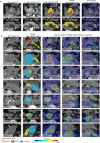
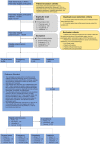
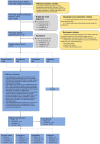
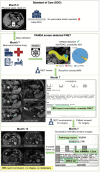
Similar articles
-
Radiomics-based Machine-learning Models Can Detect Pancreatic Cancer on Prediagnostic Computed Tomography Scans at a Substantial Lead Time Before Clinical Diagnosis.Gastroenterology. 2022 Nov;163(5):1435-1446.e3. doi: 10.1053/j.gastro.2022.06.066. Epub 2022 Jul 1. Gastroenterology. 2022. PMID: 35788343
-
CT Abnormalities of the Pancreas Associated With the Subsequent Diagnosis of Clinical Stage I Pancreatic Ductal Adenocarcinoma More Than 1 Year Later: A Case-Control Study.AJR Am J Roentgenol. 2021 Dec;217(6):1353-1364. doi: 10.2214/AJR.21.26014. Epub 2021 Jun 23. AJR Am J Roentgenol. 2021. PMID: 34161128
-
Utility of CT Radiomics Features in Differentiation of Pancreatic Ductal Adenocarcinoma From Normal Pancreatic Tissue.AJR Am J Roentgenol. 2019 Aug;213(2):349-357. doi: 10.2214/AJR.18.20901. Epub 2019 Apr 23. AJR Am J Roentgenol. 2019. PMID: 31012758
-
A review study on early detection of pancreatic ductal adenocarcinoma using artificial intelligence assisted diagnostic methods.Eur J Radiol. 2023 Sep;166:110972. doi: 10.1016/j.ejrad.2023.110972. Epub 2023 Jul 11. Eur J Radiol. 2023. PMID: 37454557 Review.
-
White paper on pancreatic ductal adenocarcinoma from society of abdominal radiology's disease-focused panel for pancreatic ductal adenocarcinoma: Part II, update on imaging techniques and screening of pancreatic cancer in high-risk individuals.Abdom Radiol (NY). 2020 Mar;45(3):729-742. doi: 10.1007/s00261-019-02290-y. Abdom Radiol (NY). 2020. PMID: 31768594 Review.
Cited by
-
Commentary on "Large-Scale Pancreatic Cancer Detection via Non-Contrast CT and Deep Learning".Biomed Eng Comput Biol. 2024 Oct 31;15:11795972241293521. doi: 10.1177/11795972241293521. eCollection 2024. Biomed Eng Comput Biol. 2024. PMID: 39494415 Free PMC article.
-
Risk Assessment and Radiomics Analysis in Magnetic Resonance Imaging of Pancreatic Intraductal Papillary Mucinous Neoplasms (IPMN).Cancer Control. 2024 Jan-Dec;31:10732748241263644. doi: 10.1177/10732748241263644. Cancer Control. 2024. PMID: 39293798 Free PMC article. Review.
-
Diagnosing Solid Lesions in the Pancreas With Multimodal Artificial Intelligence: A Randomized Crossover Trial.JAMA Netw Open. 2024 Jul 1;7(7):e2422454. doi: 10.1001/jamanetworkopen.2024.22454. JAMA Netw Open. 2024. PMID: 39028670 Free PMC article. Clinical Trial.
-
Esophageal cancer detection via non-contrast CT and deep learning.Front Med (Lausanne). 2024 Mar 6;11:1356752. doi: 10.3389/fmed.2024.1356752. eCollection 2024. Front Med (Lausanne). 2024. PMID: 38510455 Free PMC article.
-
Early detection of pancreatic cancer in the era of precision medicine.Abdom Radiol (NY). 2024 Oct;49(10):3559-3573. doi: 10.1007/s00261-024-04358-w. Epub 2024 May 18. Abdom Radiol (NY). 2024. PMID: 38761272 Review.
References
-
- Sung H, et al. Global cancer statistics 2020: GLOBOCAN estimates of incidence and mortality worldwide for 36 cancers in 185 countries. CA Cancer J. Clin. 2021;71:209–249. - PubMed
-
- Vasen H, et al. Benefit of surveillance for pancreatic cancer in high-risk individuals: outcome of long-term prospective follow-up studies from three European expert centers. J. Clin. Oncol. 2016;34:2010–2019. - PubMed
-
- Klatte DCF, et al. Pancreatic cancer surveillance in carriers of a germline CDKN2A pathogenic variant: yield and outcomes of a 20-year prospective follow-up. J. Clin. Oncol. 2022;40:3267–3277. - PubMed
Publication types
MeSH terms
Grants and funding
LinkOut - more resources
Full Text Sources
Medical