Prognostic hub gene CBX2 drives a cancer stem cell-like phenotype in HCC revealed by multi-omics and multi-cohorts
- PMID: 37980163
- PMCID: PMC10713423
- DOI: 10.18632/aging.205173
Prognostic hub gene CBX2 drives a cancer stem cell-like phenotype in HCC revealed by multi-omics and multi-cohorts
Abstract
Hepatocellular carcinoma (HCC) is a malignant tumor with a high prevalence and fatality rate. CBX2 has been demonstrated to impact the development and advancement of various cancers, albeit it has received limited attention in relation to HCC. In this study, CBX2 and CEP55 were screened out with the refined triple regulatory networks constructed by total RNA-seq datasets (TCGA-LIHC, GSE140845) and a robust prognostic model. Aberrantly higher expression levels of CBX2 and CEP55 in HCC may be caused by CNV alterations, promoter hypo-methylation, open chromatin accessibility, and greater active marks such as H3K4me3, H3K4me1, and H3K27ac. Functionally, CBX2, which was highly correlated with CD44, shaped a cancer stem cell-like phenotype by positively regulating cell-cycle progression, proliferation, invasion, metastasis, wound healing, and radiation resistance, revealed by combining bulk RNA-seq and scRNA-seq datasets. CBX2 knockdown validated its role in affecting the cell cycle. Importantly, we revealed CBX2 could activate gene by cooperating with co-regulators or not rather than a recognizer of the repressive mark H3K27me3. For instance, we uncovered CBX2 bound to promoter of CTNNB1 and CEP55 to augment their expressions. CBX2 showed a highly positive correlation with CEP55 at pan-cancer level. In addition, CBX2 and CEP55 may enhance extracellular matrix reprograming via cancer-associated fibroblast. Surprisingly, patients with high expression of CBX2 or CEP55 exhibited a higher response to immunotherapy, indicating that CBX2 and CEP55 may be promising therapeutic targets for HCC patients.
Keywords: CBX2; CEP55; HCC; cell cycle; regulatory network.
Conflict of interest statement
Figures
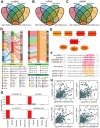
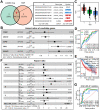
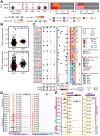
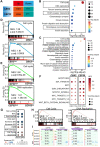
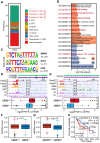
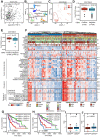
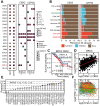
Similar articles
-
CBX2-mediated suppression of SIAH2 triggers WNK1 accumulations to promote glycolysis in hepatocellular carcinoma.Exp Cell Res. 2023 May 1;426(1):113513. doi: 10.1016/j.yexcr.2023.113513. Epub 2023 Feb 11. Exp Cell Res. 2023. PMID: 36780970
-
SPAG5 interacts with CEP55 and exerts oncogenic activities via PI3K/AKT pathway in hepatocellular carcinoma.Mol Cancer. 2018 Aug 8;17(1):117. doi: 10.1186/s12943-018-0872-3. Mol Cancer. 2018. PMID: 30089483 Free PMC article.
-
Identification of hepatocellular carcinoma subtypes based on PcG-related genes and biological relevance with cancer cells.Clin Epigenetics. 2022 Dec 24;14(1):184. doi: 10.1186/s13148-022-01393-6. Clin Epigenetics. 2022. PMID: 36566204 Free PMC article.
-
Upregulation of CEP55 Predicts Dismal Prognosis in Patients with Liver Cancer.Biomed Res Int. 2020 Apr 12;2020:4139320. doi: 10.1155/2020/4139320. eCollection 2020. Biomed Res Int. 2020. PMID: 32337246 Free PMC article.
-
Beyond EZH2: is the polycomb protein CBX2 an emerging target for anti-cancer therapy?Expert Opin Ther Targets. 2019 Jul;23(7):565-578. doi: 10.1080/14728222.2019.1627329. Epub 2019 Jun 10. Expert Opin Ther Targets. 2019. PMID: 31177918 Review.
Cited by
-
A novel super-enhancer-related risk model for predicting prognosis and guiding personalized treatment in hepatocellular carcinoma.BMC Cancer. 2024 Sep 2;24(1):1087. doi: 10.1186/s12885-024-12874-7. BMC Cancer. 2024. PMID: 39223584 Free PMC article.
-
Pyroptosis-related genes features on prediction of the prognosis in liver cancer: An integrated analysis of bulk and single-cell RNA sequencing.Heliyon. 2024 Sep 25;10(19):e38438. doi: 10.1016/j.heliyon.2024.e38438. eCollection 2024 Oct 15. Heliyon. 2024. PMID: 39416843 Free PMC article.
References
Publication types
MeSH terms
Substances
LinkOut - more resources
Full Text Sources
Medical
Miscellaneous