Estimation of Absolute and Relative Body Fat Content Using Noninvasive Surrogates: Can DXA Be Bypassed?
- PMID: 37942909
- PMCID: PMC10651165
- DOI: 10.1002/jcph.2306
Estimation of Absolute and Relative Body Fat Content Using Noninvasive Surrogates: Can DXA Be Bypassed?
Abstract
Dual-energy x-ray absorptiometry (DXA) scanning is used for objective determination of body composition, but instrumentation is expensive and not generally available in customary clinical practice. Anthropometric surrogates are often substituted as anticipated correlates of absolute and relative body fat content in the clinical management of obesity and its associated medical risks. DXA and anthropometric data from a cohort of 9230 randomly selected American subjects, available through the ongoing National Health and Nutrition Examination Survey, was used to evaluate combinations of surrogates (age, height, total weight, waist circumference) as predictors of DXA-determined absolute and relative body fat content. Multiple regression analysis yielded linear combinations of the 4 surrogates that were closely predictive of DXA-determined absolute fat content (R2 = 0.93 and 0.96 for male and female subjects). Accuracy of the new algorithm was improved over customary surrogate-based predictors such as body mass index. However prediction of relative body fat was less robust (R2 less than 0.75), probably due to the nonlinear relation between degree of obesity (based on body mass index) and relative body fat. The paradigm was validated using an independent cohort from the National Health and Nutrition Examination Survey, as well as two independent external subject groups. The described regression-based algorithm is likely to be a sufficiently accurate predictor of absolute body fat (but not relative body fat) to substitute for DXA scanning in many clinical situations. Further work is needed to assess algorithm validity for subgroups of individuals with "atypical" body construction.
Keywords: National Health and Nutrition Examination Survey (NHANES); anthropometric surrogates; body fat content; dual-energy x-ray absorptiometry (DXA); epidemiology; obesity.
© 2023, The American College of Clinical Pharmacology.
Conflict of interest statement
Conflicts of Interest
CDB and CRC are employees of Emerald Lake Safety, LLC. Other authors do not have conflicts of interest.
Figures
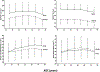
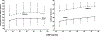
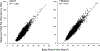
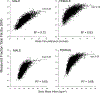
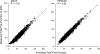
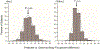
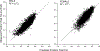
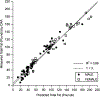
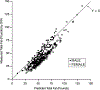
Similar articles
-
Development and validation of anthropometric prediction equations for lean body mass, fat mass and percent fat in adults using the National Health and Nutrition Examination Survey (NHANES) 1999-2006.Br J Nutr. 2017 Nov;118(10):858-866. doi: 10.1017/S0007114517002665. Epub 2017 Nov 7. Br J Nutr. 2017. PMID: 29110742
-
Relationship between indices of obesity obtained by anthropometry and dual-energy X-ray absorptiometry: The Fourth and Fifth Korea National Health and Nutrition Examination Survey (KNHANES IV and V, 2008-2011).Obes Res Clin Pract. 2015 Sep-Oct;9(5):487-98. doi: 10.1016/j.orcp.2014.11.002. Epub 2014 Dec 4. Obes Res Clin Pract. 2015. PMID: 25484303
-
Optimal scaling of weight and waist circumference to height for maximal association with DXA-measured total body fat mass by sex, age and race/ethnicity.Int J Obes (Lond). 2013 Aug;37(8):1154-60. doi: 10.1038/ijo.2012.201. Epub 2012 Dec 4. Int J Obes (Lond). 2013. PMID: 23207404
-
The prediction of visceral fat by dual-energy X-ray absorptiometry in the elderly: a comparison with computed tomography and anthropometry.Int J Obes Relat Metab Disord. 2002 Jul;26(7):984-93. doi: 10.1038/sj.ijo.0801968. Int J Obes Relat Metab Disord. 2002. PMID: 12080454
-
Validation of dual energy X-ray absorptiometry (DXA) by comparison with chemical analysis of dogs and cats.Int J Obes Relat Metab Disord. 2001 Mar;25(3):439-47. doi: 10.1038/sj.ijo.0801544. Int J Obes Relat Metab Disord. 2001. PMID: 11319644
Cited by
-
Incomplete Data and Potential Risks of Drugs in People with Obesity.Curr Obes Rep. 2023 Dec;12(4):429-438. doi: 10.1007/s13679-023-00532-1. Epub 2023 Nov 18. Curr Obes Rep. 2023. PMID: 37980304 Free PMC article. Review.
References
Publication types
MeSH terms
Grants and funding
LinkOut - more resources
Full Text Sources
Medical