Roastgsa: a comparison of rotation-based scores for gene set enrichment analysis
- PMID: 37904108
- PMCID: PMC10617084
- DOI: 10.1186/s12859-023-05510-x
Roastgsa: a comparison of rotation-based scores for gene set enrichment analysis
Abstract
Background: Gene-wise differential expression is usually the first major step in the statistical analysis of high-throughput data obtained from techniques such as microarrays or RNA-sequencing. The analysis at gene level is often complemented by interrogating the data in a broader biological context that considers as unit of measure groups of genes that may have a common function or biological trait. Among the vast number of publications about gene set analysis (GSA), the rotation test for gene set analysis, also referred to as roast, is a general sample randomization approach that maintains the integrity of the intra-gene set correlation structure in defining the null distribution of the test.
Results: We present roastgsa, an R package that contains several enrichment score functions that feed the roast algorithm for hypothesis testing. These implemented methods are evaluated using both simulated and benchmarking data in microarray and RNA-seq datasets. We find that computationally intensive measures based on Kolmogorov-Smirnov (KS) statistics fail to improve the rates of simpler measures of GSA like mean and maxmean scores. We also show the importance of accounting for the gene linear dependence structure of the testing set, which is linked to the loss of effective signature size. Complete graphical representation of the results, including an approximation for the effective signature size, can be obtained as part of the roastgsa output.
Conclusions: We encourage the usage of the absmean (non-directional), mean (directional) and maxmean (directional) scores for roast GSA analysis as these are simple measures of enrichment that have presented dominant results in all provided analyses in comparison to the more complex KS measures.
Keywords: Competitive test; Correlation; Gene set analysis; Rotation test.
© 2023. The Author(s).
Conflict of interest statement
The authors declare that they have no competing interests.
Figures
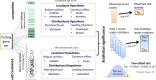
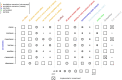
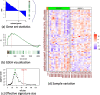
Similar articles
-
ROAST: rotation gene set tests for complex microarray experiments.Bioinformatics. 2010 Sep 1;26(17):2176-82. doi: 10.1093/bioinformatics/btq401. Epub 2010 Jul 7. Bioinformatics. 2010. PMID: 20610611 Free PMC article.
-
Linear combination test for gene set analysis of a continuous phenotype.BMC Bioinformatics. 2013 Jul 1;14:212. doi: 10.1186/1471-2105-14-212. BMC Bioinformatics. 2013. PMID: 23815123 Free PMC article.
-
Gene set analysis controlling for length bias in RNA-seq experiments.BioData Min. 2017 Feb 6;10:5. doi: 10.1186/s13040-017-0125-9. eCollection 2017. BioData Min. 2017. PMID: 28184252 Free PMC article.
-
GAGE: generally applicable gene set enrichment for pathway analysis.BMC Bioinformatics. 2009 May 27;10:161. doi: 10.1186/1471-2105-10-161. BMC Bioinformatics. 2009. PMID: 19473525 Free PMC article.
-
Gene-set analysis and reduction.Brief Bioinform. 2009 Jan;10(1):24-34. doi: 10.1093/bib/bbn042. Epub 2008 Oct 4. Brief Bioinform. 2009. PMID: 18836208 Free PMC article. Review.
Cited by
-
Release of mitochondrial dsRNA into the cytosol is a key driver of the inflammatory phenotype of senescent cells.Nat Commun. 2024 Aug 27;15(1):7378. doi: 10.1038/s41467-024-51363-0. Nat Commun. 2024. PMID: 39191740 Free PMC article.
References
MeSH terms
LinkOut - more resources
Full Text Sources