Deep learning for plant bioinformatics: an explainable gradient-based approach for disease detection
- PMID: 37900739
- PMCID: PMC10612337
- DOI: 10.3389/fpls.2023.1283235
Deep learning for plant bioinformatics: an explainable gradient-based approach for disease detection
Abstract
Emerging in the realm of bioinformatics, plant bioinformatics integrates computational and statistical methods to study plant genomes, transcriptomes, and proteomes. With the introduction of high-throughput sequencing technologies and other omics data, the demand for automated methods to analyze and interpret these data has increased. We propose a novel explainable gradient-based approach EG-CNN model for both omics data and hyperspectral images to predict the type of attack on plants in this study. We gathered gene expression, metabolite, and hyperspectral image data from plants afflicted with four prevalent diseases: powdery mildew, rust, leaf spot, and blight. Our proposed EG-CNN model employs a combination of these omics data to learn crucial plant disease detection characteristics. We trained our model with multiple hyperparameters, such as the learning rate, number of hidden layers, and dropout rate, and attained a test set accuracy of 95.5%. We also conducted a sensitivity analysis to determine the model's resistance to hyperparameter variations. Our analysis revealed that our model exhibited a notable degree of resilience in the face of these variations, resulting in only marginal changes in performance. Furthermore, we conducted a comparative examination of the time efficiency of our EG-CNN model in relation to baseline models, including SVM, Random Forest, and Logistic Regression. Although our model necessitates additional time for training and validation due to its intricate architecture, it demonstrates a faster testing time per sample, offering potential advantages in real-world scenarios where speed is paramount. To gain insights into the internal representations of our EG-CNN model, we employed saliency maps for a qualitative analysis. This visualization approach allowed us to ascertain that our model effectively captures crucial aspects of plant disease, encompassing alterations in gene expression, metabolite levels, and spectral discrepancies within plant tissues. Leveraging omics data and hyperspectral images, this study underscores the potential of deep learning methods in the realm of plant disease detection. The proposed EG-CNN model exhibited impressive accuracy and displayed a remarkable degree of insensitivity to hyperparameter variations, which holds promise for future plant bioinformatics applications.
Keywords: Omics data; deep learning; hyperspectral imaging; plant bioinformatics; plant disease detection.
Copyright © 2023 Shoaib, Shah, Sayed, Ali, Ullah and Hussain.
Conflict of interest statement
The authors declare that the research was conducted in the absence of any commercial or financial relationships that could be construed as a potential conflict of interest. The author(s) declared that they were an editorial board member of Frontiers, at the time of submission. This had no impact on the peer review process and the final decision.
Figures
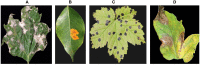
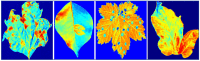
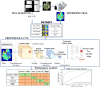
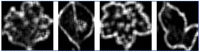
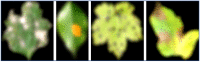
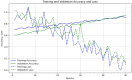
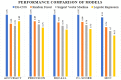
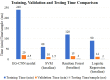
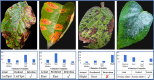
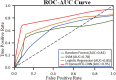
Similar articles
-
An effective approach for plant leaf diseases classification based on a novel DeepPlantNet deep learning model.Front Plant Sci. 2023 Oct 11;14:1212747. doi: 10.3389/fpls.2023.1212747. eCollection 2023. Front Plant Sci. 2023. PMID: 37900756 Free PMC article.
-
Improved classification accuracy of powdery mildew infection levels of wine grapes by spatial-spectral analysis of hyperspectral images.Plant Methods. 2017 Jun 15;13:47. doi: 10.1186/s13007-017-0198-y. eCollection 2017. Plant Methods. 2017. PMID: 28630643 Free PMC article.
-
Deep Learning Classification of Systemic Sclerosis Skin Using the MobileNetV2 Model.IEEE Open J Eng Med Biol. 2021 Mar 17;2:104-110. doi: 10.1109/OJEMB.2021.3066097. eCollection 2021. IEEE Open J Eng Med Biol. 2021. PMID: 35402975 Free PMC article.
-
Translational Metabolomics of Head Injury: Exploring Dysfunctional Cerebral Metabolism with Ex Vivo NMR Spectroscopy-Based Metabolite Quantification.In: Kobeissy FH, editor. Brain Neurotrauma: Molecular, Neuropsychological, and Rehabilitation Aspects. Boca Raton (FL): CRC Press/Taylor & Francis; 2015. Chapter 25. In: Kobeissy FH, editor. Brain Neurotrauma: Molecular, Neuropsychological, and Rehabilitation Aspects. Boca Raton (FL): CRC Press/Taylor & Francis; 2015. Chapter 25. PMID: 26269925 Free Books & Documents. Review.
-
Explainable deep learning in plant phenotyping.Front Artif Intell. 2023 Sep 19;6:1203546. doi: 10.3389/frai.2023.1203546. eCollection 2023. Front Artif Intell. 2023. PMID: 37795496 Free PMC article. Review.
Cited by
-
CSXAI: a lightweight 2D CNN-SVM model for detection and classification of various crop diseases with explainable AI visualization.Front Plant Sci. 2024 Jul 5;15:1412988. doi: 10.3389/fpls.2024.1412988. eCollection 2024. Front Plant Sci. 2024. PMID: 39036360 Free PMC article.
References
-
- Alzubaidi A., Tepper J. (2022). “Deep mining from omics dataOmics data,” in Data mining techniques for the life sciences. Eds. Carugo O., Eisenhaber F. (US: Springer; ), 349–386. doi: 10.1007/978-1-0716-2095-3_15 - DOI
Grants and funding
LinkOut - more resources
Full Text Sources