DNA Hypomethylation in the TNF-Alpha Gene Predicts Rheumatoid Arthritis Classification in Patients with Early Inflammatory Symptoms
- PMID: 37830590
- PMCID: PMC10571942
- DOI: 10.3390/cells12192376
DNA Hypomethylation in the TNF-Alpha Gene Predicts Rheumatoid Arthritis Classification in Patients with Early Inflammatory Symptoms
Abstract
Biomarkers for the classification of rheumatoid arthritis (RA), and particularly for anti-citrullinated peptide antibody (ACPA)-negative patients, remain an important hurdle for the early initiation of treatment. Taking advantage of DNA-methylation patterns specific to early RA, quantitative methylation-specific qPCR (qMSP) offers a robust technology for the development of biomarkers. We developed assays and established their value as RA classification biomarkers.
Methods: DNA-methylation data were screened to select candidate CpGs to design qMSP assays. Eight assays were developed and tested on two early inflammatory arthritis cohorts. Logistic regression and bootstrapping were used to demonstrate the added value of the qMSP assays.
Result: Differentially methylated CpG data were screened for candidate CpG, thereby meeting the qMSP assay requirements. The top CpG candidate was in the TNF gene, for which we successfully developed a qMSP assay. Significantly lower DNA-methylation levels were observed in RA (p < 4 × 10-9), with a high predictive value (OR < 0.54/AUC < 0.198) in both cohorts (n = 127/n = 157). Regression using both datasets showed improved accuracy = 87.7% and AUC = 0.944 over the model using only clinical variables (accuracy = 85.2%, AUC = 0.917). Similar data were obtained in ACPA-negative patients (n = 167, accuracy = 82.6%, AUC = 0.930) compared to the clinical variable model (accuracy = 79.5%, AUC = 0.892). Bootstrapping using 2000 datasets confirmed that the AUCs for the clinical+TNF-qMSP model had significant added value in both analyses.
Conclusion: The qMSP technology is robust and can successfully be developed with a high specificity of the TNF qMSP assay for RA in patients with early inflammatory arthritis. It should assist classification in ACPA-negative patients, providing a means of reducing time to diagnosis and treatment.
Keywords: DNA-methylation; qMSP biomarker; rheumatoid arthritis.
Conflict of interest statement
The authors declare no conflict of interest.
Figures
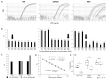
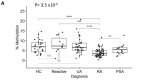
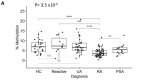
Similar articles
-
Rheumatoid arthritis-relevant DNA methylation changes identified in ACPA-positive asymptomatic individuals using methylome capture sequencing.Clin Epigenetics. 2019 Jul 31;11(1):110. doi: 10.1186/s13148-019-0699-9. Clin Epigenetics. 2019. PMID: 31366403 Free PMC article.
-
DNA methylation change of HIPK3 in Chinese rheumatoid arthritis and its effect on inflammation.Front Immunol. 2023 Jan 10;13:1087279. doi: 10.3389/fimmu.2022.1087279. eCollection 2022. Front Immunol. 2023. PMID: 36703984 Free PMC article.
-
DNA methylation mediates genotype and smoking interaction in the development of anti-citrullinated peptide antibody-positive rheumatoid arthritis.Arthritis Res Ther. 2017 Mar 29;19(1):71. doi: 10.1186/s13075-017-1276-2. Arthritis Res Ther. 2017. PMID: 28356135 Free PMC article.
-
Autoantibodies in Rheumatoid Arthritis - Laboratory and Clinical Perspectives.Front Immunol. 2021 May 14;12:685312. doi: 10.3389/fimmu.2021.685312. eCollection 2021. Front Immunol. 2021. PMID: 34054878 Free PMC article. Review.
-
[The pathogenic role of ACPA in rheumatoid arthritis].Nihon Rinsho Meneki Gakkai Kaishi. 2017;40(6):391-395. doi: 10.2177/jsci.40.391. Nihon Rinsho Meneki Gakkai Kaishi. 2017. PMID: 29367523 Review. Japanese.
References
-
- Quinn M.A., Emery P. Window of opportunity in early rheumatoid arthritis: Possibility of altering the disease process with early intervention. Clin. Exp. Rheumatol. 2003;21((Suppl. 31)):S154–S157. - PubMed
Publication types
MeSH terms
Substances
Grants and funding
LinkOut - more resources
Full Text Sources
Medical