Transcription factor-binding k-mer analysis clarifies the cell type dependency of binding specificities and cis-regulatory SNPs in humans
- PMID: 37805453
- PMCID: PMC10560430
- DOI: 10.1186/s12864-023-09692-9
Transcription factor-binding k-mer analysis clarifies the cell type dependency of binding specificities and cis-regulatory SNPs in humans
Abstract
Background: Transcription factors (TFs) exhibit heterogeneous DNA-binding specificities in individual cells and whole organisms under natural conditions, and de novo motif discovery usually provides multiple motifs, even from a single chromatin immunoprecipitation-sequencing (ChIP-seq) sample. Despite the accumulation of ChIP-seq data and ChIP-seq-derived motifs, the diversity of DNA-binding specificities across different TFs and cell types remains largely unexplored.
Results: Here, we applied MOCCS2, our k-mer-based motif discovery method, to a collection of human TF ChIP-seq samples across diverse TFs and cell types, and systematically computed profiles of TF-binding specificity scores for all k-mers. After quality control, we compiled a set of TF-binding specificity score profiles for 2,976 high-quality ChIP-seq samples, comprising 473 TFs and 398 cell types. Using these high-quality samples, we confirmed that the k-mer-based TF-binding specificity profiles reflected TF- or TF-family dependent DNA-binding specificities. We then compared the binding specificity scores of ChIP-seq samples with the same TFs but with different cell type classes and found that half of the analyzed TFs exhibited differences in DNA-binding specificities across cell type classes. Additionally, we devised a method to detect differentially bound k-mers between two ChIP-seq samples and detected k-mers exhibiting statistically significant differences in binding specificity scores. Moreover, we demonstrated that differences in the binding specificity scores between k-mers on the reference and alternative alleles could be used to predict the effect of variants on TF binding, as validated by in vitro and in vivo assay datasets. Finally, we demonstrated that binding specificity score differences can be used to interpret disease-associated non-coding single-nucleotide polymorphisms (SNPs) as TF-affecting SNPs and provide candidates responsible for TFs and cell types.
Conclusions: Our study provides a basis for investigating the regulation of gene expression in a TF-, TF family-, or cell-type-dependent manner. Furthermore, our differential analysis of binding-specificity scores highlights noncoding disease-associated variants in humans.
Keywords: Cell type dependency; ChIP-seq; DNA-binding motif; Differential k-mer analysis; Functional genomics; GWAS-SNP; Regulatory SNP; Transcription factor; k-mer-based analysis.
© 2023. BioMed Central Ltd., part of Springer Nature.
Conflict of interest statement
The authors declare no competing interests.
Figures
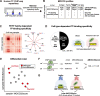
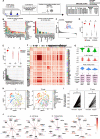
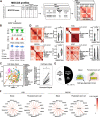
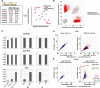
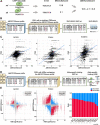
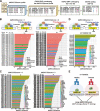
Similar articles
-
Cell-type specificity of ChIP-predicted transcription factor binding sites.BMC Genomics. 2012 Aug 3;13:372. doi: 10.1186/1471-2164-13-372. BMC Genomics. 2012. PMID: 22863112 Free PMC article.
-
A novel k-mer set memory (KSM) motif representation improves regulatory variant prediction.Genome Res. 2018 Jun;28(6):891-900. doi: 10.1101/gr.226852.117. Epub 2018 Apr 13. Genome Res. 2018. PMID: 29654070 Free PMC article.
-
Benchmarking and building DNA binding affinity models using allele-specific and allele-agnostic transcription factor binding data.Genome Biol. 2024 Oct 31;25(1):284. doi: 10.1186/s13059-024-03424-2. Genome Biol. 2024. PMID: 39482734 Free PMC article.
-
Role of ChIP-seq in the discovery of transcription factor binding sites, differential gene regulation mechanism, epigenetic marks and beyond.Cell Cycle. 2014;13(18):2847-52. doi: 10.4161/15384101.2014.949201. Cell Cycle. 2014. PMID: 25486472 Free PMC article. Review.
-
A conserved role for transcription factor sumoylation in binding-site selection.Curr Genet. 2019 Dec;65(6):1307-1312. doi: 10.1007/s00294-019-00992-w. Epub 2019 May 15. Curr Genet. 2019. PMID: 31093693 Review.
References
MeSH terms
Substances
Grants and funding
LinkOut - more resources
Full Text Sources
Miscellaneous