Explainable deep learning in plant phenotyping
- PMID: 37795496
- PMCID: PMC10546035
- DOI: 10.3389/frai.2023.1203546
Explainable deep learning in plant phenotyping
Abstract
The increasing human population and variable weather conditions, due to climate change, pose a threat to the world's food security. To improve global food security, we need to provide breeders with tools to develop crop cultivars that are more resilient to extreme weather conditions and provide growers with tools to more effectively manage biotic and abiotic stresses in their crops. Plant phenotyping, the measurement of a plant's structural and functional characteristics, has the potential to inform, improve and accelerate both breeders' selections and growers' management decisions. To improve the speed, reliability and scale of plant phenotyping procedures, many researchers have adopted deep learning methods to estimate phenotypic information from images of plants and crops. Despite the successful results of these image-based phenotyping studies, the representations learned by deep learning models remain difficult to interpret, understand, and explain. For this reason, deep learning models are still considered to be black boxes. Explainable AI (XAI) is a promising approach for opening the deep learning model's black box and providing plant scientists with image-based phenotypic information that is interpretable and trustworthy. Although various fields of study have adopted XAI to advance their understanding of deep learning models, it has yet to be well-studied in the context of plant phenotyping research. In this review article, we reviewed existing XAI studies in plant shoot phenotyping, as well as related domains, to help plant researchers understand the benefits of XAI and make it easier for them to integrate XAI into their future studies. An elucidation of the representations within a deep learning model can help researchers explain the model's decisions, relate the features detected by the model to the underlying plant physiology, and enhance the trustworthiness of image-based phenotypic information used in food production systems.
Keywords: agriculture; data bias; deep learning; explainable AI; plant phenotyping.
Copyright © 2023 Mostafa, Mondal, Panjvani, Kochian and Stavness.
Conflict of interest statement
The authors declare that the research was conducted in the absence of any commercial or financial relationships that could be construed as a potential conflict of interest.
Figures
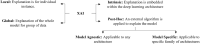
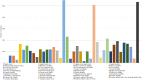
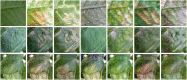
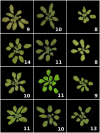
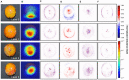
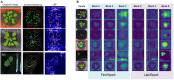
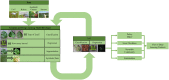
Similar articles
-
Explainable AI for Bioinformatics: Methods, Tools and Applications.Brief Bioinform. 2023 Sep 20;24(5):bbad236. doi: 10.1093/bib/bbad236. Brief Bioinform. 2023. PMID: 37478371 Review.
-
Applications of Explainable Artificial Intelligence in Diagnosis and Surgery.Diagnostics (Basel). 2022 Jan 19;12(2):237. doi: 10.3390/diagnostics12020237. Diagnostics (Basel). 2022. PMID: 35204328 Free PMC article. Review.
-
Understanding the black-box: towards interpretable and reliable deep learning models.PeerJ Comput Sci. 2023 Nov 29;9:e1629. doi: 10.7717/peerj-cs.1629. eCollection 2023. PeerJ Comput Sci. 2023. PMID: 38077598 Free PMC article.
-
Recent Advances in Explainable Artificial Intelligence for Magnetic Resonance Imaging.Diagnostics (Basel). 2023 Apr 27;13(9):1571. doi: 10.3390/diagnostics13091571. Diagnostics (Basel). 2023. PMID: 37174962 Free PMC article. Review.
-
Enhancement of Plant Productivity in the Post-Genomics Era.Curr Genomics. 2016 Aug;17(4):295-6. doi: 10.2174/138920291704160607182507. Curr Genomics. 2016. PMID: 27499678 Free PMC article.
Cited by
-
Investigating the contribution of image time series observations to cauliflower harvest-readiness prediction.Front Artif Intell. 2024 Sep 18;7:1416323. doi: 10.3389/frai.2024.1416323. eCollection 2024. Front Artif Intell. 2024. PMID: 39359647 Free PMC article.
-
Explainable artificial intelligence for genotype-to-phenotype prediction in plant breeding: a case study with a dataset from an almond germplasm collection.Front Plant Sci. 2024 Sep 9;15:1434229. doi: 10.3389/fpls.2024.1434229. eCollection 2024. Front Plant Sci. 2024. PMID: 39319003 Free PMC article.
-
Advancing plant biology through deep learning-powered natural language processing.Plant Cell Rep. 2024 Aug 5;43(8):208. doi: 10.1007/s00299-024-03294-9. Plant Cell Rep. 2024. PMID: 39102077 Review.
-
Fast and Efficient Root Phenotyping via Pose Estimation.Plant Phenomics. 2024 Apr 12;6:0175. doi: 10.34133/plantphenomics.0175. eCollection 2024. Plant Phenomics. 2024. PMID: 38629082 Free PMC article.
References
-
- Adadi A., Berrada M. (2018). Peeking inside the black-box: a survey on explainable artificial intelligence (XAI). IEEE Access 6, 52138–52160. 10.1109/ACCESS.2018.2870052 - DOI
-
- Adebayo J., Gilmer J., Goodfellow I., Kim B. (2018a). Local explanation methods for deep neural networks lack sensitivity to parameter values. arXiv:1810.03307v1.
-
- Adebayo J., Gilmer J., Muelly M., Goodfellow I., Hardt M., Kim B. (2018b). Sanity checks for saliency maps. Adv. Neural Inf. Process. Syst. 31.
-
- Agarwal R., Melnick L., Frosst N., Zhang X., Lengerich B., Caruana R., et al. . (2021). Neural additive models: interpretable machine learning with neural nets. Adv. Neural Inf. Process. Syst. 34, 4699–4711. Available online at: https://proceedings.neurips.cc/paper_files/paper/2021/file/251bd0442dfcc... - PubMed
-
- Aich S., Stavness I. (2017). “Leaf counting with deep convolutional and deconvolutional networks,” in Proceedings of the IEEE International Conference on Computer Vision Workshops (Italy: ), 2080–2089.
Publication types
Grants and funding
LinkOut - more resources
Full Text Sources