Integrative evaluation and experimental validation of the immune-modulating potential of dysregulated extracellular matrix genes in high-grade serous ovarian cancer prognosis
- PMID: 37777759
- PMCID: PMC10543838
- DOI: 10.1186/s12935-023-03061-y
Integrative evaluation and experimental validation of the immune-modulating potential of dysregulated extracellular matrix genes in high-grade serous ovarian cancer prognosis
Abstract
Background: High-grade serous ovarian cancer (HGSOC) is a challenging malignancy characterized by complex interactions between tumor cells and the surrounding microenvironment. Understanding the immune landscape of HGSOC, particularly the role of the extracellular matrix (ECM), is crucial for improving prognosis and guiding therapeutic interventions.
Methods and results: Using univariate Cox regression analysis, we identified 71 ECM genes associated with prognosis in seven HGSOC populations. The ECMscore signature, consisting of 14 genes, was validated using Cox proportional hazards regression with a lasso penalty. Cox regression analyses demonstrated that ECMscore is an excellent indicator for prognostic classification in prevalent malignancies, including HGSOC. Moreover, patients with higher ECMscores exhibited more active stromal and carcinogenic activation pathways, including apical surface signaling, Notch signaling, apical junctions, Wnt signaling, epithelial-mesenchymal transition, TGF-beta signaling, and angiogenesis. In contrast, patients with relatively low ECMscores showed more active immune-related pathways, such as interferon alpha response, interferon-gamma response, and inflammatory response. The relationship between the ECMscore and genomic anomalies was further examined. Additionally, the correlation between ECMscore and immune microenvironment components and signals in HGSOC was examined in greater detail. Moreover, the expression of MGP, COL8A2, and PAPPA and its correlation with FAP were validated using qRT-PCR on samples from HGSOC. The utility of ECMscore in predicting the prospective clinical success of immunotherapy and its potential in guiding the selection of chemotherapeutic agents were also explored. Similar results were obtained from pan-cancer research.
Conclusion: The comprehensive evaluation of the ECM may help identify immune activation and assist patients in HGSOC and even pan-cancer in receiving proper therapy.
Keywords: Extracellular matrix; Immunity; Ovarian carcinoma; Prognosis; Treatment.
© 2023. BioMed Central Ltd., part of Springer Nature.
Conflict of interest statement
The authors declare no competing interests.
Figures
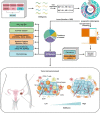
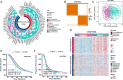
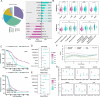
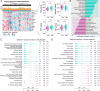
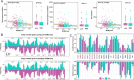
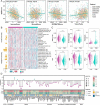
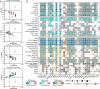
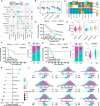
Similar articles
-
Deciphering the immune landscape dominated by cancer-associated fibroblasts to investigate their potential in indicating prognosis and guiding therapeutic regimens in high grade serous ovarian carcinoma.Front Immunol. 2022 Sep 2;13:940801. doi: 10.3389/fimmu.2022.940801. eCollection 2022. Front Immunol. 2022. PMID: 36119108 Free PMC article.
-
Machine learning-based integration develops an immune-related risk model for predicting prognosis of high-grade serous ovarian cancer and providing therapeutic strategies.Front Immunol. 2023 Apr 5;14:1164408. doi: 10.3389/fimmu.2023.1164408. eCollection 2023. Front Immunol. 2023. PMID: 37090728 Free PMC article.
-
Development and validation of an individualized gene expression-based signature to predict overall survival of patients with high-grade serous ovarian carcinoma.Eur J Med Res. 2023 Oct 27;28(1):465. doi: 10.1186/s40001-023-01376-0. Eur J Med Res. 2023. PMID: 37884970 Free PMC article.
-
Extracellular matrix in high-grade serous ovarian cancer: Advances in understanding of carcinogenesis and cancer biology.Matrix Biol. 2023 Apr;118:16-46. doi: 10.1016/j.matbio.2023.02.004. Epub 2023 Feb 11. Matrix Biol. 2023. PMID: 36781087 Review.
-
Improving PARP inhibitor efficacy in high-grade serous ovarian carcinoma: A focus on the immune system.Front Genet. 2022 Sep 9;13:886170. doi: 10.3389/fgene.2022.886170. eCollection 2022. Front Genet. 2022. PMID: 36159999 Free PMC article. Review.
Cited by
-
Fibroblast activation protein (FAP) as a prognostic biomarker in multiple tumors and its therapeutic potential in head and neck squamous cell carcinoma.Oncol Res. 2024 Jul 17;32(8):1323-1334. doi: 10.32604/or.2024.046965. eCollection 2024. Oncol Res. 2024. PMID: 39055892 Free PMC article.
References
-
- Sung H, Ferlay J, Siegel RL, Laversanne M, Soerjomataram I, Jemal A, Bray F. Global Cancer Statistics 2020: GLOBOCAN estimates of incidence and Mortality Worldwide for 36 cancers in 185 countries. CA Cancer J Clin. 2021;71(3):209–49. - PubMed
-
- Siegel RL, Miller KD, Fuchs HE, Jemal A. Cancer statistics, 2022. CA Cancer J Clin. 2022;72(1):7–33. - PubMed
Grants and funding
LinkOut - more resources
Full Text Sources
Miscellaneous