Polygenic risk scores: the future of cancer risk prediction, screening, and precision prevention
- PMID: 37724297
- PMCID: PMC10471106
- DOI: 10.1515/mr-2021-0025
Polygenic risk scores: the future of cancer risk prediction, screening, and precision prevention
Abstract
Genome-wide association studies (GWASs) have shown that the genetic architecture of cancers are highly polygenic and enabled researchers to identify genetic risk loci for cancers. The genetic variants associated with a cancer can be combined into a polygenic risk score (PRS), which captures part of an individual's genetic susceptibility to cancer. Recently, PRSs have been widely used in cancer risk prediction and are shown to be capable of identifying groups of individuals who could benefit from the knowledge of their probabilistic susceptibility to cancer, which leads to an increased interest in understanding the potential utility of PRSs that might further refine the assessment and management of cancer risk. In this context, we provide an overview of the major discoveries from cancer GWASs. We then review the methodologies used for PRS construction, and describe steps for the development and evaluation of risk prediction models that include PRS and/or conventional risk factors. Potential utility of PRSs in cancer risk prediction, screening, and precision prevention are illustrated. Challenges and practical considerations relevant to the implementation of PRSs in health care settings are discussed.
Keywords: cancer screening; genome-wide association study (GWAS); polygenic risk score (PRS); precision prevention; risk prediction model.
© 2021 Yuzhuo Wang et al., published by De Gruyter, Berlin/Boston.
Conflict of interest statement
Competing interests: All the authors declare that there is no conflict of interest.
Figures
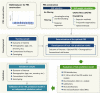
Similar articles
-
Polygenic risk scores for the prediction of common cancers in East Asians: A population-based prospective cohort study.Elife. 2023 Mar 27;12:e82608. doi: 10.7554/eLife.82608. Elife. 2023. PMID: 36971353 Free PMC article.
-
Comparison of Methods Utilizing Sex-Specific PRSs Derived From GWAS Summary Statistics.Front Genet. 2022 Jul 8;13:892950. doi: 10.3389/fgene.2022.892950. eCollection 2022. Front Genet. 2022. PMID: 35873490 Free PMC article.
-
Polygenic risk scores: An overview from bench to bedside for personalised medicine.Front Genet. 2022 Nov 11;13:1000667. doi: 10.3389/fgene.2022.1000667. eCollection 2022. Front Genet. 2022. PMID: 36437929 Free PMC article. Review.
-
Polygenic risk scores: from research tools to clinical instruments.Genome Med. 2020 May 18;12(1):44. doi: 10.1186/s13073-020-00742-5. Genome Med. 2020. PMID: 32423490 Free PMC article. Review.
-
Genome-wide association studies and polygenic risk scores for skin cancer: clinically useful yet?Br J Dermatol. 2019 Dec;181(6):1146-1155. doi: 10.1111/bjd.17917. Epub 2019 Jul 7. Br J Dermatol. 2019. PMID: 30908599 Free PMC article. Review.
Cited by
-
Recent advances in polygenic scores: translation, equitability, methods and FAIR tools.Genome Med. 2024 Feb 19;16(1):33. doi: 10.1186/s13073-024-01304-9. Genome Med. 2024. PMID: 38373998 Free PMC article. Review.
-
Polygenic Risk Score (PRS) Combined with NGS Panel Testing Increases Accuracy in Hereditary Breast Cancer Risk Estimation.Diagnostics (Basel). 2024 Aug 21;14(16):1826. doi: 10.3390/diagnostics14161826. Diagnostics (Basel). 2024. PMID: 39202314 Free PMC article.
-
Associations of metabolic changes and polygenic risk scores with cardiovascular outcomes and all-cause mortality across BMI categories: a prospective cohort study.Cardiovasc Diabetol. 2024 Jul 4;23(1):231. doi: 10.1186/s12933-024-02332-w. Cardiovasc Diabetol. 2024. PMID: 38965592 Free PMC article.
-
Implementation of Whole-Genome and Transcriptome Sequencing Into Clinical Cancer Care.JCO Precis Oncol. 2022 Dec;6:e2200245. doi: 10.1200/PO.22.00245. JCO Precis Oncol. 2022. PMID: 36480778 Free PMC article. Review.
-
Understanding metabolic reprogramming in tumor microenvironment.Med Rev (2021). 2022 Feb 14;1(2):111-113. doi: 10.1515/mr-2021-0037. eCollection 2021 Dec. Med Rev (2021). 2022. PMID: 37724298 Free PMC article. No abstract available.
References
-
- Global Health Estimates 2020 . Deaths by cause, age, sex, by country and by region, 2000-2019. Geneva: World Health Organization; 2020.
Publication types
LinkOut - more resources
Full Text Sources