A Predictive Rule for COVID-19 Pneumonia Among COVID-19 Patients: A Classification and Regression Tree (CART) Analysis Model
- PMID: 37720137
- PMCID: PMC10500617
- DOI: 10.7759/cureus.45199
A Predictive Rule for COVID-19 Pneumonia Among COVID-19 Patients: A Classification and Regression Tree (CART) Analysis Model
Abstract
Background: In this study, we aimed to identify predictive factors for coronavirus disease 2019 (COVID-19) patients with complicated pneumonia and determine which COVID-19 patients should undergo computed tomography (CT) using classification and regression tree (CART) analysis.
Methods: This retrospective cross-sectional survey was conducted at a university hospital. We recruited patients diagnosed with COVID-19 between January 1 and December 31, 2020. We extracted clinical information (e.g., vital signs, symptoms, laboratory results, and CT findings) from patient records. Factors potentially predicting COVID-19 pneumonia were analyzed using Student's t-test, the chi-square test, and a CART analysis model.
Results: Among 221 patients (119 men (53.8%); mean age, 54.59±18.61 years), 160 (72.4%) had pneumonia. The CART analysis revealed that patients were at high risk of pneumonia if they had C-reactive protein (CRP) levels of >1.60 mg/dL (incidence of pneumonia: 95.7%); CRP levels of ≤1.60 mg/dL + age >35.5 years + lactate dehydrogenase (LDH)>225.5 IU/L (incidence of pneumonia: 95.5%); and CRP levels of ≤1.60 mg/dL + age >35.5 years + LDH≤225.5 IU/L + hemoglobin ≤14.65 g/dL (incidence of pneumonia: 69.6%). The area of the curve of the receiver operating characteristic of the model was 0.860 (95% CI: 0.804-0.915), indicating sufficient explanatory power.
Conclusions: The present results are useful for deciding whether to perform CT in COVID-19 patients. High-risk patients such as those mentioned above should undergo CT.
Keywords: blood testing; clinical feature; computed tomography; prevalent infection; severe acute respiratory syndrome coronavirus 2.
Copyright © 2023, Fukui et al.
Conflict of interest statement
The authors have declared that no competing interests exist.
Figures
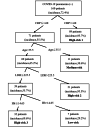
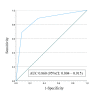
Similar articles
-
Prediction models for respiratory outcomes in patients with COVID-19: integration of quantitative computed tomography parameters, demographics, and laboratory features.J Thorac Dis. 2023 Mar 31;15(3):1506-1516. doi: 10.21037/jtd-22-1076. Epub 2023 Mar 9. J Thorac Dis. 2023. PMID: 37065603 Free PMC article.
-
A deep learning-based quantitative computed tomography model for predicting the severity of COVID-19: a retrospective study of 196 patients.Ann Transl Med. 2021 Feb;9(3):216. doi: 10.21037/atm-20-2464. Ann Transl Med. 2021. PMID: 33708843 Free PMC article.
-
Determining extent of COVID-19 pneumonia on CT based on biological variables.Respir Med. 2020 Dec;175:106206. doi: 10.1016/j.rmed.2020.106206. Epub 2020 Nov 5. Respir Med. 2020. PMID: 33166904 Free PMC article.
-
Visual and Quantitative Assessment of COVID-19 Pneumonia on Chest CT: The Relationship with Disease Severity and Clinical Findings.Curr Med Imaging. 2021;17(9):1142-1150. doi: 10.2174/1573405617666210215142528. Curr Med Imaging. 2021. PMID: 33588737
-
Clinical Features of 154 COVID-19 Patients and the Parameters for the Effective Detection of Pneumonia at the Time of the Initial Diagnosis in Japan.Intern Med. 2021 Jan 1;60(1):31-37. doi: 10.2169/internalmedicine.5528-20. Epub 2020 Nov 2. Intern Med. 2021. PMID: 33132330 Free PMC article.
References
-
- The epidemiological characteristics of an outbreak of 2019 novel coronavirus diseases (COVID-19) in China (Article in Chinese) Zhonghua Liu Xing Bing Xue Za Zhi. 2020;41:145–151. - PubMed
-
- World Health Organization: Coronavirus disease (COVID-19) pandemic. [ Oct; 2020 ]. 2020. https://www.who.int/europe/emergencies/situations/covid-19 https://www.who.int/europe/emergencies/situations/covid-19
LinkOut - more resources
Full Text Sources
Research Materials
Miscellaneous