Dominance vs epistasis: the biophysical origins and plasticity of genetic interactions within and between alleles
- PMID: 37689712
- PMCID: PMC10492795
- DOI: 10.1038/s41467-023-41188-8
Dominance vs epistasis: the biophysical origins and plasticity of genetic interactions within and between alleles
Abstract
An important challenge in genetics, evolution and biotechnology is to understand and predict how mutations combine to alter phenotypes, including molecular activities, fitness and disease. In diploids, mutations in a gene can combine on the same chromosome or on different chromosomes as a "heteroallelic combination". However, a direct comparison of the extent, sign, and stability of the genetic interactions between variants within and between alleles is lacking. Here we use thermodynamic models of protein folding and ligand-binding to show that interactions between mutations within and between alleles are expected in even very simple biophysical systems. Protein folding alone generates within-allele interactions and a single molecular interaction is sufficient to cause between-allele interactions and dominance. These interactions change differently, quantitatively and qualitatively as a system becomes more complex. Altering the concentration of a ligand can, for example, switch alleles from dominant to recessive. Our results show that intra-molecular epistasis and dominance should be widely expected in even the simplest biological systems but also reinforce the view that they are plastic system properties and so a formidable challenge to predict. Accurate prediction of both intra-molecular epistasis and dominance will require either detailed mechanistic understanding and experimental parameterization or brute-force measurement and learning.
© 2023. Springer Nature Limited.
Conflict of interest statement
The authors declare no competing interests.
Figures
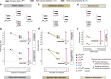
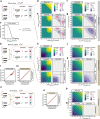
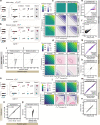
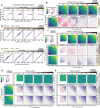
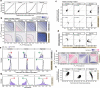
Similar articles
-
Effects of genetic drift on variance components under a general model of epistasis.Evolution. 2004 Oct;58(10):2111-32. doi: 10.1111/j.0014-3820.2004.tb01591.x. Evolution. 2004. PMID: 15562679
-
Distributions of epistasis in microbes fit predictions from a fitness landscape model.Nat Genet. 2007 Apr;39(4):555-60. doi: 10.1038/ng1998. Epub 2007 Mar 18. Nat Genet. 2007. PMID: 17369829
-
Estimating the contribution of folding stability to nonspecific epistasis in protein evolution.Proteins. 2018 Dec;86(12):1242-1250. doi: 10.1002/prot.25588. Epub 2018 Nov 9. Proteins. 2018. PMID: 30039542
-
REVIEW: Epistasis and dominance in the emergence of catalytic function as exemplified by the evolution of plant terpene synthases.Plant Sci. 2017 Feb;255:29-38. doi: 10.1016/j.plantsci.2016.11.006. Epub 2016 Nov 14. Plant Sci. 2017. PMID: 28131339 Review.
-
The Causes and Consequences of Genetic Interactions (Epistasis).Annu Rev Genomics Hum Genet. 2019 Aug 31;20:433-460. doi: 10.1146/annurev-genom-083118-014857. Epub 2019 May 13. Annu Rev Genomics Hum Genet. 2019. PMID: 31082279 Review.
Cited by
-
Higher-order epistasis within Pol II trigger loop haplotypes.bioRxiv [Preprint]. 2024 Sep 25:2024.01.20.576280. doi: 10.1101/2024.01.20.576280. bioRxiv. 2024. Update in: Genetics. 2024 Oct 24:iyae172. doi: 10.1093/genetics/iyae172 PMID: 38293233 Free PMC article. Updated. Preprint.
References
Publication types
MeSH terms
Substances
LinkOut - more resources
Full Text Sources