Evaluating the ability of end-point methods to predict the binding affinity tendency of protein kinase inhibitors
- PMID: 37614784
- PMCID: PMC10443623
- DOI: 10.1039/d3ra04916g
Evaluating the ability of end-point methods to predict the binding affinity tendency of protein kinase inhibitors
Abstract
Because of the high economic cost of exploring the experimental impact of mutations occurring in kinase proteins, computational approaches have been employed as alternative methods for evaluating the structural and energetic aspects of kinase mutations. Among the main computational methods used to explore the affinity linked to kinase mutations are docking procedures and molecular dynamics (MD) simulations combined with end-point methods or alchemical methods. Although it is known that end-point methods are not able to reproduce experimental binding free energy (ΔG) values, it is also true that they are able to discriminate between a better or a worse ligand through the estimation of ΔG. In this contribution, we selected ten wild-type and mutant cocrystallized EGFR-inhibitor complexes containing experimental binding affinities to evaluate whether MMGBSA or MMPBSA approaches can predict the differences in affinity between the wild type and mutants forming a complex with a similar inhibitor. Our results show that a long MD simulation (the last 50 ns of a 100 ns-long MD simulation) using the MMGBSA method without considering the entropic components reproduced the experimental affinity tendency with a Pearson correlation coefficient of 0.779 and an R2 value of 0.606. On the other hand, the correlation between theoretical and experimental ΔΔG values indicates that the MMGBSA and MMPBSA methods are helpful for obtaining a good correlation using a short rather than a long simulation period.
This journal is © The Royal Society of Chemistry.
Conflict of interest statement
The authors declare they have no conflict of interest in terms of the content of this manuscript.
Figures
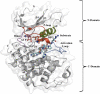
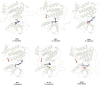
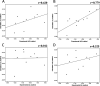
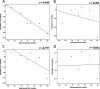
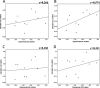
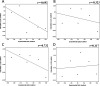
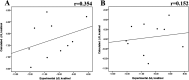
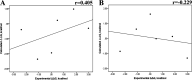
Similar articles
-
Statistical Analysis on the Performance of Molecular Mechanics Poisson-Boltzmann Surface Area versus Absolute Binding Free Energy Calculations: Bromodomains as a Case Study.J Chem Inf Model. 2017 Sep 25;57(9):2203-2221. doi: 10.1021/acs.jcim.7b00347. Epub 2017 Aug 24. J Chem Inf Model. 2017. PMID: 28786670 Free PMC article.
-
Macromolecular crowding: chemistry and physics meet biology (Ascona, Switzerland, 10-14 June 2012).Phys Biol. 2013 Aug;10(4):040301. doi: 10.1088/1478-3975/10/4/040301. Epub 2013 Aug 2. Phys Biol. 2013. PMID: 23912807
-
The impact of simulation time in predicting binding free energies using end-point approaches.J Bioinform Comput Biol. 2022 Oct;20(5):2250024. doi: 10.1142/S021972002250024X. Epub 2022 Nov 9. J Bioinform Comput Biol. 2022. PMID: 36350600
-
Computing Clinically Relevant Binding Free Energies of HIV-1 Protease Inhibitors.J Chem Theory Comput. 2014 Mar 11;10(3):1228-1241. doi: 10.1021/ct4007037. Epub 2014 Jan 27. J Chem Theory Comput. 2014. PMID: 24683369 Free PMC article.
-
Current Tools and Methods in Molecular Dynamics (MD) Simulations for Drug Design.Curr Med Chem. 2016;23(34):3909-3924. doi: 10.2174/0929867323666160530144742. Curr Med Chem. 2016. PMID: 27237821 Review.
Cited by
-
Understanding the impact of binding free energy and kinetics calculations in modern drug discovery.Expert Opin Drug Discov. 2024 Jun;19(6):671-682. doi: 10.1080/17460441.2024.2349149. Epub 2024 May 9. Expert Opin Drug Discov. 2024. PMID: 38722032 Review.
References
-
- Manning G. and Hunter T., Eukaryotic Kinomes: Genomics and Evolution of Protein Kinases, in Handb. Cell Signaling, Academic Press, California, 2nd edn, 2010, pp. 393–397
-
- Bossemeyer D. Protein kinases—structure and function. FEBS Lett. 1995;369(1):57–61. - PubMed
-
- Weber T. J. and Qian W., Protein Kinases, in Compr. Toxicol, Elsevier Ltd, AL, USA, 3rd edn, 2018, pp. 264–285
-
- Cruzalegui F. Protein Kinases: From Targets to Anti-Cancer Drugs. Ann. Pharm. Fr. 2010;68(4):254–259. - PubMed
LinkOut - more resources
Full Text Sources
Research Materials
Miscellaneous