Machine Learning-derived Multi-omics Prognostic Signature of Pyroptosis-related lncRNA with Regard to ZKSCAN2-DT and Tumor Immune Infiltration in Colorectal Cancer
- PMID: 37612868
- PMCID: PMC11327744
- DOI: 10.2174/1386207326666230823104952
Machine Learning-derived Multi-omics Prognostic Signature of Pyroptosis-related lncRNA with Regard to ZKSCAN2-DT and Tumor Immune Infiltration in Colorectal Cancer
Abstract
Background: Colorectal cancer (CRC) has become the most prevalent gastrointestinal malignant tumor, ranking third (10.2%) in incidence and second (9.2%) in death among all malignancies globally. The most common histological subtype of CRC is colon adenocarcinoma (COAD), although the cause of CRC remains unknown, as there are no valid biomarkers.
Methods: A thorough investigation was used to build a credible biomolecular risk model based on the pyroptosis-associated lncRNAs discovered for COAD prediction. Furthermore, Cibersort and Tumor Immune Dysfunction and Exclusion (TIDE), the methods of exploring tumor immune infiltration, were adopted in our paper to detect the effects of differential lncRNAs on the tumor microenvironment. Finally, quantitative real-time polymerase chain reaction (qPCR), as the approach of exploring expressions, was utilized on four different cell lines.
Results: Seven pyroptosis-related lncRNAs have been identified as COAD predictive risk factors. Cox analysis, both univariate and multivariate, revealed that the established signature might serve as a novel independent factor with prognostic meaning in COAD patients. ZKSCAN2-DT was shown to be considerably overexpressed in the COAD cell line when compared to normal human colonic epithelial cells. Furthermore, ssGSEA analysis results revealed that the immune infiltration percentage of most immune cells dropped considerably as ZKSCAN2-DT expression increased, implying that ZKSCAN2-DT may play an important role in COAD immunotherapy.
Conclusion: Our research is the first to identify pyroptosis-related lncRNAs connected with COAD patient prognosis and to construct a predictive prognosis signature, directing COAD patient prognosis in therapeutic interventions.
Keywords: Colon adenocarcinoma; biomarkers; colorectal cancer (CRC).; immune infiltration; prognosis; pyroptosis-related lncRNA.
Copyright© Bentham Science Publishers; For any queries, please email at epub@benthamscience.net.
Conflict of interest statement
The authors declared no conflict of interest, financial or otherwise.
Figures
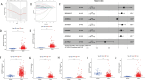
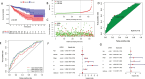
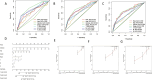
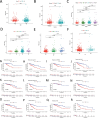
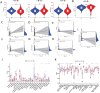
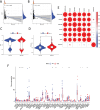
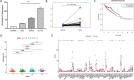
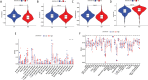
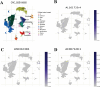
Similar articles
-
Research progress on the effect of pyroptosis on the occurrence, development, invasion and metastasis of colorectal cancer.World J Gastrointest Oncol. 2024 Aug 15;16(8):3410-3427. doi: 10.4251/wjgo.v16.i8.3410. World J Gastrointest Oncol. 2024. PMID: 39171180 Free PMC article. Review.
-
Construction and Validation of Pyroptosis-Related lncRNA Prediction Model for Colon Adenocarcinoma and Immune Infiltration Analysis.Dis Markers. 2022 Sep 17;2022:4492608. doi: 10.1155/2022/4492608. eCollection 2022. Dis Markers. 2022. PMID: 36168326 Free PMC article.
-
Developing a RiskScore Model based on Angiogenesis-related lncRNAs for Colon Adenocarcinoma Prognostic Prediction.Curr Med Chem. 2024;31(17):2449-2466. doi: 10.2174/0109298673277243231108071620. Curr Med Chem. 2024. PMID: 37961859
-
Identification of a pyroptosis-related lncRNA risk model for predicting prognosis and immune response in colon adenocarcinoma.World J Surg Oncol. 2022 Apr 12;20(1):118. doi: 10.1186/s12957-022-02572-8. World J Surg Oncol. 2022. PMID: 35413978 Free PMC article.
-
The Role and Therapeutic Potential of Pyroptosis in Colorectal Cancer: A Review.Biomolecules. 2024 Jul 20;14(7):874. doi: 10.3390/biom14070874. Biomolecules. 2024. PMID: 39062587 Free PMC article. Review.
Cited by
-
Research progress on the effect of pyroptosis on the occurrence, development, invasion and metastasis of colorectal cancer.World J Gastrointest Oncol. 2024 Aug 15;16(8):3410-3427. doi: 10.4251/wjgo.v16.i8.3410. World J Gastrointest Oncol. 2024. PMID: 39171180 Free PMC article. Review.
References
-
- Villariba-Tolentino C., Cariño A.M., Notarte K.I., Macaranas I., Fellizar A., Tomas R.C., Angeles L.M., Abanilla L., Lim A., Aguilar M.K.C., Albano P.M. pks+ Escherichia coli more prevalent in benign than malignant colorectal tumors. Mol. Biol. Rep. 2021;48(7):5451–5458. doi: 10.1007/s11033-021-06552-1. - DOI - PubMed
MeSH terms
Substances
Grants and funding
LinkOut - more resources
Full Text Sources
Medical