Exploring the cellular and molecular differences between ovarian clear cell carcinoma and high-grade serous carcinoma using single-cell RNA sequencing and GEO gene expression signatures
- PMID: 37525249
- PMCID: PMC10391916
- DOI: 10.1186/s13578-023-01087-3
Exploring the cellular and molecular differences between ovarian clear cell carcinoma and high-grade serous carcinoma using single-cell RNA sequencing and GEO gene expression signatures
Abstract
The two most prevalent subtypes of epithelial ovarian carcinoma (EOC) are ovarian clear cell carcinoma (OCCC) and high-grade serous ovarian carcinoma (HGSC). Patients with OCCC have a poor prognosis than those with HGSC due to chemoresistance, implying the need for novel treatment target. In this study, we applied single-cell RNA sequencing (scRNA-seq) together with bulk RNA-seq data from the GEO (Gene Expression Omnibus) database (the GSE189553 dataset) to characterize and compare tumor heterogeneity and cell-level evolution between OCCC and HGSC samples. To begin, we found that the smaller proportion of an epithelial OCCC cell subset in the G2/M phase might explain OCCC chemoresistance. Second, we identified a possible pathogenic OCCC epithelial cell subcluster that overexpresses LEFTY1. Third, novel biomarkers separating OCCC from HGSC were discovered and subsequently validated on a wide scale using immunohistochemistry. Amine oxidase copper containing 1 (AOC1) was preferentially expressed in OCCC over HGSC, while S100 calcium-binding protein A2 (S100A2) was detected less frequently in OCCC than in HGSC. In addition, we discovered that metabolic pathways were enriched in the epithelial compartment of the OCCC samples. In vitro experiments verified that inhibition of oxidative phosphorylation or glycolysis pathways exerted direct antitumor effects on both OCCC and HGSC cells, while targeting glutamine metabolism or ferroptosis greatly attenuated chemosensitivity only in OCCC cells. Finally, to determine whether there were any variations in immune cell subsets between OCCC and HGSC, data from scRNA-seq and mass cytometry were pooled for analysis. In summary, our work provides the first holistic insights into the cellular and molecular distinctions between OCCC and HGSC and is a valuable source for discovering new targets to leverage in clinical treatments to improve the poor prognosis of patients with OCCC.
Keywords: Bulk RNA-seq; HGSC; OCCC; Single-cell RNA-seq.
© 2023. The Author(s).
Conflict of interest statement
The authors declare that they have no competing interests.
Figures
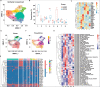
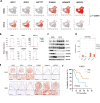
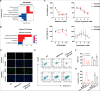
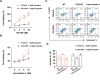
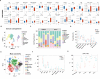
Similar articles
-
HNF-1β in ovarian carcinomas with serous and clear cell change.Int J Gynecol Pathol. 2013 Nov;32(6):541-6. doi: 10.1097/PGP.0b013e318273fd07. Int J Gynecol Pathol. 2013. PMID: 24071869 Free PMC article.
-
Single-cell RNA-sequencing analysis reveals divergent transcriptome events between platinum-sensitive and platinum-resistant high-grade serous ovarian carcinoma.J Gene Med. 2023 Oct;25(10):e3504. doi: 10.1002/jgm.3504. Epub 2023 Apr 26. J Gene Med. 2023. PMID: 36994597
-
Genomic scar signatures associated with homologous recombination deficiency predict adverse clinical outcomes in patients with ovarian clear cell carcinoma.J Mol Med (Berl). 2018 Jun;96(6):527-536. doi: 10.1007/s00109-018-1643-8. Epub 2018 May 3. J Mol Med (Berl). 2018. PMID: 29725737
-
Current and future strategies for treatment of ovarian clear cell carcinoma.J Obstet Gynaecol Res. 2020 Sep;46(9):1678-1689. doi: 10.1111/jog.14350. Epub 2020 Jun 23. J Obstet Gynaecol Res. 2020. PMID: 32578333 Review.
-
Cell Origins of High-Grade Serous Ovarian Cancer.Cancers (Basel). 2018 Nov 12;10(11):433. doi: 10.3390/cancers10110433. Cancers (Basel). 2018. PMID: 30424539 Free PMC article. Review.
Cited by
-
Redox-Regulated Iron Metabolism and Ferroptosis in Ovarian Cancer: Molecular Insights and Therapeutic Opportunities.Antioxidants (Basel). 2024 Jun 28;13(7):791. doi: 10.3390/antiox13070791. Antioxidants (Basel). 2024. PMID: 39061859 Free PMC article. Review.
References
Grants and funding
- 2023-PUMCH-F-004/the National High Level Hospital Clinical Research Funding
- 2022-PUMCH-B-062/the National High Level Hospital Clinical Research Funding
- 2022-PUMCH-D-002/the National High Level Hospital Clinical Research Funding
- 2021-I2M-1-053/Chinese Academy of Medical Sciences Initiative for Innovative Medicine
- No. 8197544, PB-QY012108/the National Natural Science Foundation of China
LinkOut - more resources
Full Text Sources
Research Materials
Miscellaneous