The PANoptosis-related signature indicates the prognosis and tumor immune infiltration features of gliomas
- PMID: 37501725
- PMCID: PMC10369193
- DOI: 10.3389/fnmol.2023.1198713
The PANoptosis-related signature indicates the prognosis and tumor immune infiltration features of gliomas
Abstract
Background: Gliomas are the most common primary tumors of the central nervous system, with high heterogeneity and highly variable survival rates. Accurate classification and prognostic assessment are key to the selection of treatment strategies. One hallmark of the tumor is resistance to cell death. PANoptosis, a novel mode of programmed cell death, has been frequently reported to be involved in the innate immunity associated with pathogen infection and played an important role in cancers. However, the intrinsic association of PANoptosis with glioma requires deeper investigation.
Methods: The genetics and expression of the 17 reported PANoptosome-related genes were analyzed in glioma. Based on these genes, patients were divided into two subtypes by consensus clustering analysis. After obtaining the differentially expressed genes between clusters, a prognostic model called PANopotic score was constructed after univariate Cox regression, LASSO regression, and multivariate Cox regression. The expression of the 5 genes included in the PANopotic score was also examined by qPCR in our cohort. The prognostic differences, clinical features, TME infiltration status, and immune characteristics between PANoptotic clusters and score groups were compared, some of which even extended to pan-cancer levels.
Results: Gene mutations, CNVs and altered gene expression of PANoptosome-related genes exist in gliomas. Two PANoptotic clusters were significantly different in prognosis, clinical features, immune characteristics, and mutation landscapes. The 5 genes included in the PANopotic score had significantly altered expression in glioma samples in our cohort. The high PANoptotic score group was inclined to show an unfavorable prognosis, lower tumor purity, worse molecular genetic signature, and distinct immune characteristics related to immunotherapy. The PANoptotic score was considered as an independent prognostic factor for glioma and showed superior prognostic assessment efficacy over several reported models. PANopotic score was included in the nomogram constructed for the potential clinical prognostic application. The associations of PANoptotic score with prognostic assessment and tumor immune characteristics were also reflected at the pan-cancer level.
Conclusion: Molecular subtypes of glioma based on PANoptosome-related genes were proposed and PANoptotic score was constructed with different clinical characteristics of anti-tumor immunity. The potential intrinsic association between PANoptosis and glioma subtypes, prognosis, and immunotherapy was revealed.
Keywords: PANoptosis; PANoptosome; glioma; immune cell infiltration; prognosis.
Copyright © 2023 Song, Xu, Fan, Sun and Lin.
Conflict of interest statement
The authors declare that the research was conducted in the absence of any commercial or financial relationships that could be construed as a potential conflict of interest.
Figures
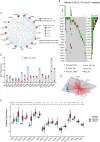
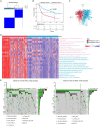
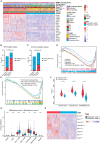
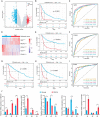
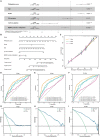
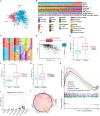
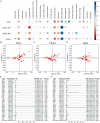
Similar articles
-
PANoptosis subtypes predict prognosis and immune efficacy in gastric cancer.Apoptosis. 2024 Jun;29(5-6):799-815. doi: 10.1007/s10495-023-01931-4. Epub 2024 Feb 12. Apoptosis. 2024. PMID: 38347337
-
The Landscape of the Tumor Microenvironment in Skin Cutaneous Melanoma Reveals a Prognostic and Immunotherapeutically Relevant Gene Signature.Front Cell Dev Biol. 2021 Oct 1;9:739594. doi: 10.3389/fcell.2021.739594. eCollection 2021. Front Cell Dev Biol. 2021. PMID: 34660598 Free PMC article.
-
PANoptosis-related molecular clustering and prognostic signature associated with the immune landscape and therapy response in breast cancer.Medicine (Baltimore). 2024 Sep 13;103(37):e39511. doi: 10.1097/MD.0000000000039511. Medicine (Baltimore). 2024. PMID: 39287311 Free PMC article.
-
Identification of a novel cuproptosis-related gene signature and integrative analyses in patients with lower-grade gliomas.Front Immunol. 2022 Aug 15;13:933973. doi: 10.3389/fimmu.2022.933973. eCollection 2022. Front Immunol. 2022. PMID: 36045691 Free PMC article. Review.
-
Molecular subtypes based on PANoptosis-related genes and tumor microenvironment infiltration characteristics in lower-grade glioma.Funct Integr Genomics. 2023 Mar 17;23(2):84. doi: 10.1007/s10142-023-01003-5. Funct Integr Genomics. 2023. Retraction in: Funct Integr Genomics. 2024 May 11;24(3):88. doi: 10.1007/s10142-024-01374-3. PMID: 36930242 Retracted. Review.
Cited by
-
PANoptosis: bridging apoptosis, pyroptosis, and necroptosis in cancer progression and treatment.Cancer Gene Ther. 2024 Jul;31(7):970-983. doi: 10.1038/s41417-024-00765-9. Epub 2024 Mar 29. Cancer Gene Ther. 2024. PMID: 38553639 Free PMC article. Review.
-
Characterizing PANoptosis gene signature in prognosis and chemosensitivity of colorectal cancer.J Gastrointest Oncol. 2024 Oct 31;15(5):2129-2144. doi: 10.21037/jgo-24-245. Epub 2024 Oct 29. J Gastrointest Oncol. 2024. PMID: 39554569 Free PMC article.
-
Current evidence and therapeutic implication of PANoptosis in cancer.Theranostics. 2024 Jan 1;14(2):640-661. doi: 10.7150/thno.91814. eCollection 2024. Theranostics. 2024. PMID: 38169587 Free PMC article. Review.
References
-
- Charrad M., Ghazzali N., Boiteau V., Niknafs A. (2014). NbClust: an R package for determining the relevant number of clusters in a data set. J. Stat. Softw. 61, 1–36. doi: 10.18637/jss.v061.i06 - DOI
-
- Christgen S., Zheng M., Kesavardhana S., Karki R., Malireddi R. K. S., Banoth B., et al. . (2020). Identification of the PANoptosome: a molecular platform triggering Pyroptosis, apoptosis, and necroptosis (PANoptosis). Front. Cell. Infect. Microbiol. 10:237. doi: 10.3389/fcimb.2020.00237, PMID: - DOI - PMC - PubMed
LinkOut - more resources
Full Text Sources