Identifying the personalized driver gene sets maximally contributing to abnormality of transcriptome phenotype in glioblastoma multiforme individuals
- PMID: 37491836
- PMCID: PMC10620122
- DOI: 10.1002/1878-0261.13499
Identifying the personalized driver gene sets maximally contributing to abnormality of transcriptome phenotype in glioblastoma multiforme individuals
Abstract
High heterogeneity in genome and phenotype of cancer populations made it difficult to apply population-based common driver genes to the diagnosis and treatment of cancer individuals. Characterizing and identifying the personalized driver mechanism for glioblastoma multiforme (GBM) individuals were pivotal for the realization of precision medicine. We proposed an integrative method to identify the personalized driver gene sets by integrating the profiles of gene expression and genetic alterations in cancer individuals. This method coupled genetic algorithm and random walk to identify the optimal gene sets that could explain abnormality of transcriptome phenotype to the maximum extent. The personalized driver gene sets were identified for 99 GBM individuals using our method. We found that genomic alterations in between one and seven driver genes could maximally and cumulatively explain the dysfunction of cancer hallmarks across GBM individuals. The driver gene sets were distinct even in GBM individuals with significantly similar transcriptomic phenotypes. Our method identified MCM4 with rare genetic alterations as previously unknown oncogenic genes, the high expression of which were significantly associated with poor GBM prognosis. The functional experiments confirmed that knockdown of MCM4 could significantly inhibit proliferation, invasion, migration, and clone formation of the GBM cell lines U251 and U118MG, and overexpression of MCM4 significantly promoted the proliferation, invasion, migration, and clone formation of the GBM cell line U87MG. Our method could dissect the personalized driver genetic alteration sets that are pivotal for developing targeted therapy strategies and precision medicine. Our method could be extended to identify key drivers from other levels and could be applied to more cancer types.
Keywords: cancer heterogeneity; driver gene sets; genetic algorithm; integrative analysis; personalization; random walk.
© 2023 The Authors. Molecular Oncology published by John Wiley & Sons Ltd on behalf of Federation of European Biochemical Societies.
Conflict of interest statement
The authors declare no conflict of interest.
Figures
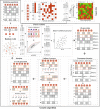
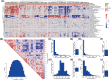
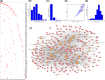
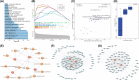
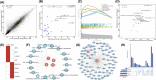
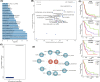
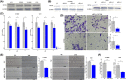
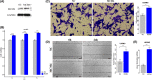
Similar articles
-
Network analysis of genomic alteration profiles reveals co-altered functional modules and driver genes for glioblastoma.Mol Biosyst. 2013 Mar;9(3):467-77. doi: 10.1039/c2mb25528f. Epub 2013 Jan 23. Mol Biosyst. 2013. PMID: 23344900
-
Identifying core gene modules in glioblastoma based on multilayer factor-mediated dysfunctional regulatory networks through integrating multi-dimensional genomic data.Nucleic Acids Res. 2015 Feb 27;43(4):1997-2007. doi: 10.1093/nar/gkv074. Epub 2015 Feb 4. Nucleic Acids Res. 2015. PMID: 25653168 Free PMC article.
-
An integrative characterization of recurrent molecular aberrations in glioblastoma genomes.Nucleic Acids Res. 2013 Oct;41(19):8803-21. doi: 10.1093/nar/gkt656. Epub 2013 Jul 31. Nucleic Acids Res. 2013. PMID: 23907387 Free PMC article.
-
Genomic, epigenomic and transcriptomic landscape of glioblastoma.Metab Brain Dis. 2024 Dec;39(8):1591-1611. doi: 10.1007/s11011-024-01414-8. Epub 2024 Aug 24. Metab Brain Dis. 2024. PMID: 39180605 Review.
-
Systems Biology Approaches to Decipher the Underlying Molecular Mechanisms of Glioblastoma Multiforme.Int J Mol Sci. 2021 Dec 8;22(24):13213. doi: 10.3390/ijms222413213. Int J Mol Sci. 2021. PMID: 34948010 Free PMC article. Review.
References
-
- Pon JR, Marra MA. Driver and passenger mutations in cancer. Annu Rev Pathol. 2015;10:25–50. - PubMed
MeSH terms
Grants and funding
- 32170675/National Natural Science Foundation of China
- 32000459/National Natural Science Foundation of China
- 82173321/National Natural Science Foundation of China
- UNPYSCT-2018068/Special Funds for the Construction of Higher Education in Heilongjiang Province
- LBH-Q20147/Heilongjiang Postdoctoral Scientific Research Developmental Fund
LinkOut - more resources
Full Text Sources
Medical