Machine Learning in Predicting Printable Biomaterial Formulations for Direct Ink Writing
- PMID: 37469394
- PMCID: PMC10353544
- DOI: 10.34133/research.0197
Machine Learning in Predicting Printable Biomaterial Formulations for Direct Ink Writing
Abstract
Three-dimensional (3D) printing is emerging as a transformative technology for biomedical engineering. The 3D printed product can be patient-specific by allowing customizability and direct control of the architecture. The trial-and-error approach currently used for developing the composition of printable inks is time- and resource-consuming due to the increasing number of variables requiring expert knowledge. Artificial intelligence has the potential to reshape the ink development process by forming a predictive model for printability from experimental data. In this paper, we constructed machine learning (ML) algorithms including decision tree, random forest (RF), and deep learning (DL) to predict the printability of biomaterials. A total of 210 formulations including 16 different bioactive and smart materials and 4 solvents were 3D printed, and their printability was assessed. All ML methods were able to learn and predict the printability of a variety of inks based on their biomaterial formulations. In particular, the RF algorithm has achieved the highest accuracy (88.1%), precision (90.6%), and F1 score (87.0%), indicating the best overall performance out of the 3 algorithms, while DL has the highest recall (87.3%). Furthermore, the ML algorithms have predicted the printability window of biomaterials to guide the ink development. The printability map generated with DL has finer granularity than other algorithms. ML has proven to be an effective and novel strategy for developing biomaterial formulations with desired 3D printability for biomedical engineering applications.
Copyright © 2023 Hongyi Chen et al.
Figures
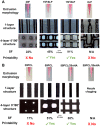
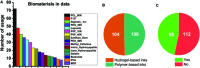
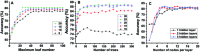
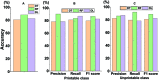
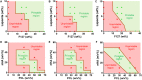
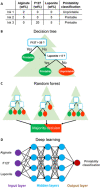
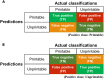
Similar articles
-
Image-based assessment and machine learning-enabled prediction of printability of polysaccharides-based food ink for 3D printing.Food Res Int. 2023 Nov;173(Pt 2):113384. doi: 10.1016/j.foodres.2023.113384. Epub 2023 Aug 18. Food Res Int. 2023. PMID: 37803721
-
Machine Learning Reveals a General Understanding of Printability in Formulations Based on Rheology Additives.Adv Sci (Weinh). 2022 Oct;9(29):e2202638. doi: 10.1002/advs.202202638. Epub 2022 Aug 25. Adv Sci (Weinh). 2022. PMID: 36008135 Free PMC article.
-
Composite Inks for Extrusion Printing of Biological and Biomedical Constructs.ACS Biomater Sci Eng. 2021 Sep 13;7(9):4009-4026. doi: 10.1021/acsbiomaterials.0c01158. Epub 2020 Nov 10. ACS Biomater Sci Eng. 2021. PMID: 34510905 Review.
-
M3DISEEN: A novel machine learning approach for predicting the 3D printability of medicines.Int J Pharm. 2020 Nov 30;590:119837. doi: 10.1016/j.ijpharm.2020.119837. Epub 2020 Sep 20. Int J Pharm. 2020. PMID: 32961295
-
The current state of the art in gellan-based printing inks in tissue engineering.Carbohydr Polym. 2023 Jun 1;309:120676. doi: 10.1016/j.carbpol.2023.120676. Epub 2023 Feb 8. Carbohydr Polym. 2023. PMID: 36906360 Review.
Cited by
-
Recent advances and applications of artificial intelligence in 3D bioprinting.Biophys Rev (Melville). 2024 Jul 19;5(3):031301. doi: 10.1063/5.0190208. eCollection 2024 Sep. Biophys Rev (Melville). 2024. PMID: 39036708 Review.
-
Let's Print an Ecology in 3D (and 4D).Materials (Basel). 2024 May 7;17(10):2194. doi: 10.3390/ma17102194. Materials (Basel). 2024. PMID: 38793260 Free PMC article. Review.
-
Programmable biomaterials for bone regeneration.Mater Today Bio. 2024 Oct 9;29:101296. doi: 10.1016/j.mtbio.2024.101296. eCollection 2024 Dec. Mater Today Bio. 2024. PMID: 39469314 Free PMC article. Review.
-
Recent Development of Photochromic Polymer Systems: Mechanism, Materials, and Applications.Research (Wash D C). 2024 Jun 17;7:0392. doi: 10.34133/research.0392. eCollection 2024. Research (Wash D C). 2024. PMID: 38894714 Free PMC article.
-
Bridging the Gap: Integrating 3D Bioprinting and Microfluidics for Advanced Multi-Organ Models in Biomedical Research.Bioengineering (Basel). 2024 Jun 28;11(7):664. doi: 10.3390/bioengineering11070664. Bioengineering (Basel). 2024. PMID: 39061746 Free PMC article.
References
-
- Lai J, Wang C, Wang M. 3D printing in biomedical engineering: Processes, materials, and applications. Appl Phys Rev. 2021;8(2): Article 021322.
-
- Murphy SV, Atala A. 3D bioprinting of tissues and organs. Nat Biotechnol. 2014;32(8):773–785. - PubMed
LinkOut - more resources
Full Text Sources