Comparative Analysis of Cell Mixtures Deconvolution and Gene Signatures Generated for Blood, Immune and Cancer Cells
- PMID: 37445946
- PMCID: PMC10341895
- DOI: 10.3390/ijms241310765
Comparative Analysis of Cell Mixtures Deconvolution and Gene Signatures Generated for Blood, Immune and Cancer Cells
Abstract
In the last two decades, many detailed full transcriptomic studies on complex biological samples have been published and included in large gene expression repositories. These studies primarily provide a bulk expression signal for each sample, including multiple cell-types mixed within the global signal. The cellular heterogeneity in these mixtures does not allow the activity of specific genes in specific cell types to be identified. Therefore, inferring relative cellular composition is a very powerful tool to achieve a more accurate molecular profiling of complex biological samples. In recent decades, computational techniques have been developed to solve this problem by applying deconvolution methods, designed to decompose cell mixtures into their cellular components and calculate the relative proportions of these elements. Some of them only calculate the cell proportions (supervised methods), while other deconvolution algorithms can also identify the gene signatures specific for each cell type (unsupervised methods). In these work, five deconvolution methods (CIBERSORT, FARDEEP, DECONICA, LINSEED and ABIS) were implemented and used to analyze blood and immune cells, and also cancer cells, in complex mixture samples (using three bulk expression datasets). Our study provides three analytical tools (corrplots, cell-signature plots and bar-mixture plots) that allow a thorough comparative analysis of the cell mixture data. The work indicates that CIBERSORT is a robust method optimized for the identification of immune cell-types, but not as efficient in the identification of cancer cells. We also found that LINSEED is a very powerful unsupervised method that provides precise and specific gene signatures for each of the main immune cell types tested: neutrophils and monocytes (of the myeloid lineage), B-cells, NK cells and T-cells (of the lymphoid lineage), and also for cancer cells.
Keywords: bioinformatics; blood cells; cell mixture; deconvolution; gene signature; immune cells.
Conflict of interest statement
The authors declare no conflict of interest.
Figures
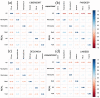
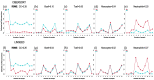
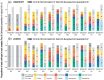
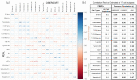
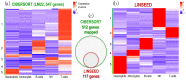
Similar articles
-
Assessing transcriptomic heterogeneity of single-cell RNASeq data by bulk-level gene expression data.BMC Bioinformatics. 2024 Jun 12;25(1):209. doi: 10.1186/s12859-024-05825-3. BMC Bioinformatics. 2024. PMID: 38867193 Free PMC article.
-
Semi-CAM: A semi-supervised deconvolution method for bulk transcriptomic data with partial marker gene information.Sci Rep. 2020 Mar 25;10(1):5434. doi: 10.1038/s41598-020-62330-2. Sci Rep. 2020. PMID: 32214192 Free PMC article.
-
Improved cell composition deconvolution method of bulk gene expression profiles to quantify subsets of immune cells.BMC Med Genomics. 2019 Dec 20;12(Suppl 8):169. doi: 10.1186/s12920-019-0613-5. BMC Med Genomics. 2019. PMID: 31856824 Free PMC article.
-
A review of digital cytometry methods: estimating the relative abundance of cell types in a bulk of cells.Brief Bioinform. 2021 Jul 20;22(4):bbaa219. doi: 10.1093/bib/bbaa219. Brief Bioinform. 2021. PMID: 33003193 Free PMC article. Review.
-
Computational deconvolution of transcriptomics data from mixed cell populations.Bioinformatics. 2018 Jun 1;34(11):1969-1979. doi: 10.1093/bioinformatics/bty019. Bioinformatics. 2018. PMID: 29351586 Review.
Cited by
-
High tumor glucocorticoid receptor expression in early-stage, triple-negative breast cancer is associated with increased T-regulatory cell infiltration.Breast Cancer Res Treat. 2024 Nov 23. doi: 10.1007/s10549-024-07515-3. Online ahead of print. Breast Cancer Res Treat. 2024. PMID: 39579248
-
Deconvolution analysis identified altered hepatic cell landscape in primary sclerosing cholangitis and primary biliary cholangitis.Front Med (Lausanne). 2024 May 15;11:1327973. doi: 10.3389/fmed.2024.1327973. eCollection 2024. Front Med (Lausanne). 2024. PMID: 38818402 Free PMC article.
-
Blood cell indices and inflammation-related markers with kidney cancer risk: a large-population prospective analysis in UK Biobank.Front Oncol. 2024 May 23;14:1366449. doi: 10.3389/fonc.2024.1366449. eCollection 2024. Front Oncol. 2024. PMID: 38846978 Free PMC article.
-
RNA-sequencing of Human Kidney Allografts and Delineation of T-Cell Genes, Gene Sets, and Pathways Associated With Acute T Cell-mediated Rejection.Transplantation. 2024 Apr 1;108(4):911-922. doi: 10.1097/TP.0000000000004896. Epub 2024 Mar 23. Transplantation. 2024. PMID: 38291584
-
CATD: a reproducible pipeline for selecting cell-type deconvolution methods across tissues.Bioinform Adv. 2024 Mar 23;4(1):vbae048. doi: 10.1093/bioadv/vbae048. eCollection 2024. Bioinform Adv. 2024. PMID: 38638280 Free PMC article.
References
-
- Wong P.F., Wei W., Smithy J.W., Acs B., Toki M.I., Blenman K.R.M., Zelterman D., Kluger H.M., Rimm D.L. Multiplex Quantitative Analysis of Tumor-Infiltrating Lymphocytes and Immunotherapy Outcome in Metastatic Melanoma. Clin. Cancer Res. 2019;25:2442–2449. doi: 10.1158/1078-0432.CCR-18-2652. - DOI - PMC - PubMed
-
- Uryvaev A., Passhak M., Hershkovits D., Sabo E., Bar-Sela G. The role of tumor-infiltrating lymphocytes (TILs) as a predictive biomarker of response to anti-PD1 therapy in patients with metastatic non-small cell lung cancer or metastatic melanoma. Med. Oncol. 2018;35:25. doi: 10.1007/s12032-018-1080-0. - DOI - PubMed
MeSH terms
LinkOut - more resources
Full Text Sources