Aging Fly Cell Atlas identifies exhaustive aging features at cellular resolution
- PMID: 37319212
- PMCID: PMC10829769
- DOI: 10.1126/science.adg0934
Aging Fly Cell Atlas identifies exhaustive aging features at cellular resolution
Abstract
Aging is characterized by a decline in tissue function, but the underlying changes at cellular resolution across the organism remain unclear. Here, we present the Aging Fly Cell Atlas, a single-nucleus transcriptomic map of the whole aging Drosophila. We characterized 163 distinct cell types and performed an in-depth analysis of changes in tissue cell composition, gene expression, and cell identities. We further developed aging clock models to predict fly age and show that ribosomal gene expression is a conserved predictive factor for age. Combining all aging features, we find distinctive cell type-specific aging patterns. This atlas provides a valuable resource for studying fundamental principles of aging in complex organisms.
Conflict of interest statement
Figures
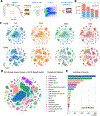
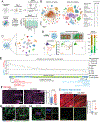
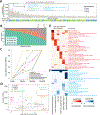
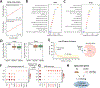
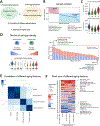
Similar articles
-
Fly Cell Atlas: A single-nucleus transcriptomic atlas of the adult fruit fly.Science. 2022 Mar 4;375(6584):eabk2432. doi: 10.1126/science.abk2432. Epub 2022 Mar 4. Science. 2022. PMID: 35239393 Free PMC article.
-
Transcriptome profiling of aging Drosophila photoreceptors reveals gene expression trends that correlate with visual senescence.BMC Genomics. 2017 Nov 21;18(1):894. doi: 10.1186/s12864-017-4304-3. BMC Genomics. 2017. PMID: 29162050 Free PMC article.
-
A Single-Cell Transcriptome Atlas of the Aging Drosophila Brain.Cell. 2018 Aug 9;174(4):982-998.e20. doi: 10.1016/j.cell.2018.05.057. Epub 2018 Jun 18. Cell. 2018. PMID: 29909982 Free PMC article.
-
Functional senescence in Drosophila melanogaster.Ageing Res Rev. 2005 Aug;4(3):372-97. doi: 10.1016/j.arr.2005.04.001. Ageing Res Rev. 2005. PMID: 16024299 Review.
-
As time flies by: Investigating cardiac aging in the short-lived Drosophila model.Biochim Biophys Acta Mol Basis Dis. 2019 Jul 1;1865(7):1831-1844. doi: 10.1016/j.bbadis.2018.11.010. Epub 2018 Nov 27. Biochim Biophys Acta Mol Basis Dis. 2019. PMID: 30496794 Free PMC article. Review.
Cited by
-
How to Build the Virtual Cell with Artificial Intelligence: Priorities and Opportunities.ArXiv [Preprint]. 2024 Oct 14:arXiv:2409.11654v2. ArXiv. 2024. PMID: 39398201 Free PMC article. Preprint.
-
Targeted single cell expression profiling identifies integrators of sleep and metabolic state.bioRxiv [Preprint]. 2024 Sep 27:2024.09.25.614841. doi: 10.1101/2024.09.25.614841. bioRxiv. 2024. PMID: 39386468 Free PMC article. Preprint.
-
Asynchronous changes of hydrogen sulfide and its generating enzymes in most tissues with the aging process.Biosci Rep. 2024 Oct 30;44(10):BSR20240320. doi: 10.1042/BSR20240320. Biosci Rep. 2024. PMID: 39312181 Free PMC article.
-
3D chromatin structures associated with ncRNA roX2 for hyperactivation and coactivation across the entire X chromosome.Sci Adv. 2024 Jul 26;10(30):eado5716. doi: 10.1126/sciadv.ado5716. Epub 2024 Jul 26. Sci Adv. 2024. PMID: 39058769 Free PMC article.
-
Improved whole-mount immunofluorescence protocol for consistent and robust labeling of adult Drosophila melanogaster adipose tissue.Biol Open. 2024 Aug 15;13(8):bio060491. doi: 10.1242/bio.060491. Epub 2024 Aug 1. Biol Open. 2024. PMID: 39041865 Free PMC article.
References
MeSH terms
Grants and funding
LinkOut - more resources
Full Text Sources
Medical
Molecular Biology Databases