Identification and verification of aging-related lncRNAs for prognosis prediction and immune microenvironment in patients with head and neck squamous carcinoma
- PMID: 37303739
- PMCID: PMC10208083
- DOI: 10.32604/or.2022.028193
Identification and verification of aging-related lncRNAs for prognosis prediction and immune microenvironment in patients with head and neck squamous carcinoma
Abstract
Aging is highly associated with tumor formation and progression. However, little research has explored the association of aging-related lncRNAs (ARLs) with the prognosis and tumor immune microenvironment (TIME) of head and neck squamous cell carcinoma (HNSCC). RNA sequences and clinicopathological data of HNSCC patients and normal subjects were downloaded from The Cancer Genome Atlas. In the training group, we used Pearson correlation, univariate Cox regression, least absolute shrinkage/selection operator regression analyses, and multivariate Cox regression to build a prognostic model. In the test group, we evaluated the model. Multivariate Cox regression was done to screen out independent prognostic factors, with which we constructed a nomogram. Afterward, we demonstrated the predictive value of the risk scores based on the model and the nomogram using time-dependent receiver operating characteristics. Gene set enrichment analysis, immune correlation analysis, and half-maximal inhibitory concentration were also performed to reveal the different landscapes of TIME between risk groups and to predict immuno- and chemo-therapeutic responses. The most important LINC00861 in the model was examined in HNE1, CNE1, and CNE2 nasopharyngeal carcinoma cell lines and transfected into the cell lines CNE1 and CNE2 using the LINC00861-pcDNA3.1 construct plasmid. In addition, CCK-8, Edu, and SA-β-gal staining assays were conducted to test the biofunction of LINC00861 in the CNE1 and CNE2 cells. The signature based on nine ARLs has a good predictive value in survival time, immune infiltration, immune checkpoint expression, and sensitivity to multiple drugs. LINC00861 expression in CNE2 was significantly lower than in the HNE1 and CNE1 cells, and LINC00861 overexpression significantly inhibited the proliferation and increased the senescence of nasopharyngeal carcinoma cell lines. This work built and verified a new prognostic model for HNSCC based on ARLs and mapped the immune landscape in HNSCC. LINC00861 is a protective factor for the development of HNSCC.
Keywords: Aging; Bioinformatics; HNSCC; Prognosis; Tumor immune microenvironment; lncRNA.
© 2023 Gao et al.
Conflict of interest statement
The authors declare that they have no conflicts of interest to report regarding the present study.
Figures
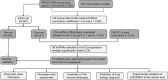
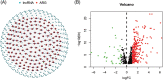
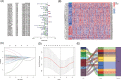
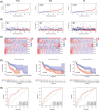
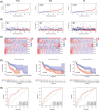
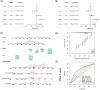

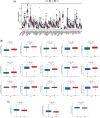
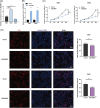
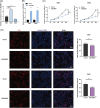
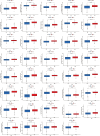
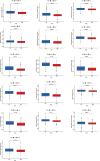
Similar articles
-
A pyroptosis-related lncRNA signature predicts prognosis and immune microenvironment in head and neck squamous cell carcinoma.Int Immunopharmacol. 2021 Dec;101(Pt B):108268. doi: 10.1016/j.intimp.2021.108268. Epub 2021 Oct 20. Int Immunopharmacol. 2021. PMID: 34688154
-
Predicting the Immune Microenvironment and Prognosis with a NETosis-Related lncRNA Signature in Head and Neck Squamous Cell Carcinoma.Biomed Res Int. 2022 Sep 12;2022:3191474. doi: 10.1155/2022/3191474. eCollection 2022. Biomed Res Int. 2022. PMID: 36147630 Free PMC article.
-
Necrotic related-lncRNAs: Prediction of prognosis and differentiation between cold and hot tumors in head and neck squamous cell carcinoma.Medicine (Baltimore). 2023 Jun 9;102(23):e33994. doi: 10.1097/MD.0000000000033994. Medicine (Baltimore). 2023. PMID: 37335630 Free PMC article.
-
Development and experimental verification of a prognosis model for disulfidptosis-associated genes in HNSCC.Medicine (Baltimore). 2024 Mar 22;103(12):e37308. doi: 10.1097/MD.0000000000037308. Medicine (Baltimore). 2024. PMID: 38518012 Free PMC article. Review.
-
Genetically engineered mouse models of head and neck cancers.Oncogene. 2023 Aug;42(35):2593-2609. doi: 10.1038/s41388-023-02783-7. Epub 2023 Jul 20. Oncogene. 2023. PMID: 37474617 Free PMC article. Review.
Cited by
-
Developing risk models and subtypes of autophagy-associated LncRNAs for enhanced prognostic prediction and precision in therapeutic approaches for liver cancer patients.Oncol Res. 2024 Mar 20;32(4):703-716. doi: 10.32604/or.2023.030988. eCollection 2024. Oncol Res. 2024. PMID: 38560571 Free PMC article.
-
Molecular Characterization of Cuproptosis-related lncRNAs: Defining Molecular Subtypes and a Prognostic Signature of Ovarian Cancer.Biol Trace Elem Res. 2024 Apr;202(4):1428-1445. doi: 10.1007/s12011-023-03780-3. Epub 2023 Aug 2. Biol Trace Elem Res. 2024. PMID: 37528285
References
MeSH terms
Substances
LinkOut - more resources
Full Text Sources
Medical