This is a preprint.
MRT-ModSeq - Rapid detection of RNA modifications with MarathonRT
- PMID: 37292902
- PMCID: PMC10245971
- DOI: 10.1101/2023.05.25.542276
MRT-ModSeq - Rapid detection of RNA modifications with MarathonRT
Update in
-
MRT-ModSeq - Rapid Detection of RNA Modifications with MarathonRT.J Mol Biol. 2023 Nov 15;435(22):168299. doi: 10.1016/j.jmb.2023.168299. Epub 2023 Oct 4. J Mol Biol. 2023. PMID: 37802215
Abstract
Chemical modifications are essential regulatory elements that modulate the behavior and function of cellular RNAs. Despite recent advances in sequencing-based RNA modification mapping, methods combining accuracy and speed are still lacking. Here, we introduce MRT- ModSeq for rapid, simultaneous detection of multiple RNA modifications using MarathonRT. MRT-ModSeq employs distinct divalent cofactors to generate 2-D mutational profiles that are highly dependent on nucleotide identity and modification type. As a proof of concept, we use the MRT fingerprints of well-studied rRNAs to implement a general workflow for detecting RNA modifications. MRT-ModSeq rapidly detects positions of diverse modifications across a RNA transcript, enabling assignment of m1acp3Y, m1A, m3U, m7G and 2'-OMe locations through mutation-rate filtering and machine learning. m1A sites in sparsely modified targets, such as MALAT1 and PRUNE1 could also be detected. MRT-ModSeq can be trained on natural and synthetic transcripts to expedite detection of diverse RNA modification subtypes across targets of interest.
Conflict of interest statement
DECLARATION OF INTERESTS
The authors declare no competing interests.
Figures
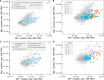
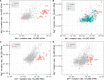
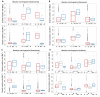
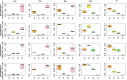
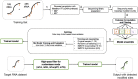
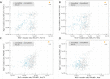
Similar articles
-
MRT-ModSeq - Rapid Detection of RNA Modifications with MarathonRT.J Mol Biol. 2023 Nov 15;435(22):168299. doi: 10.1016/j.jmb.2023.168299. Epub 2023 Oct 4. J Mol Biol. 2023. PMID: 37802215
-
Detection of internal N7-methylguanosine (m7G) RNA modifications by mutational profiling sequencing.Nucleic Acids Res. 2019 Nov 18;47(20):e126. doi: 10.1093/nar/gkz736. Nucleic Acids Res. 2019. PMID: 31504776 Free PMC article.
-
Quantitative profiling N1-methyladenosine (m1A) RNA methylation from Oxford nanopore direct RNA sequencing data.Methods. 2024 Aug;228:30-37. doi: 10.1016/j.ymeth.2024.05.009. Epub 2024 May 18. Methods. 2024. PMID: 38768930
-
[Advances in mapping analysis of ribonucleic acid modifications through sequencing].Se Pu. 2024 Jul;42(7):632-645. doi: 10.3724/SP.J.1123.2023.12025. Se Pu. 2024. PMID: 38966972 Free PMC article. Review. Chinese.
-
M7G-related tumor immunity: novel insights of RNA modification and potential therapeutic targets.Int J Biol Sci. 2024 Jan 27;20(4):1238-1255. doi: 10.7150/ijbs.90382. eCollection 2024. Int J Biol Sci. 2024. PMID: 38385078 Free PMC article. Review.
References
-
- Begik O., Lucas M.C., Pryszcz L.P., Ramirez J.M., Medina R., Milenkovic I., Cruciani S., Liu H., Vieira H.G.S., Sas-Chen A., et al. (2021). Quantitative profiling of pseudouridylation dynamics in native RNAs with nanopore sequencing. Nat Biotechnol 39, 1278–1291. 10.1038/s41587-021-00915-6. - DOI - PubMed
Publication types
Grants and funding
LinkOut - more resources
Full Text Sources
Molecular Biology Databases
Research Materials