Community- and genome-based evidence for a shaping influence of redox potential on bacterial protein evolution
- PMID: 37289197
- PMCID: PMC10308962
- DOI: 10.1128/msystems.00014-23
Community- and genome-based evidence for a shaping influence of redox potential on bacterial protein evolution
Abstract
Despite deep interest in how environments shape microbial communities, whether redox conditions influence the sequence composition of genomes is not well known. We predicted that the carbon oxidation state (ZC) of protein sequences would be positively correlated with redox potential (Eh). To test this prediction, we used taxonomic classifications for 68 publicly available 16S rRNA gene sequence data sets to estimate the abundances of archaeal and bacterial genomes in river & seawater, lake & pond, geothermal, hyperalkaline, groundwater, sediment, and soil environments. Locally, ZC of community reference proteomes (i.e., all the protein sequences in each genome, weighted by taxonomic abundances but not by protein abundances) is positively correlated with Eh corrected to pH 7 (Eh7) for the majority of data sets for bacterial communities in each type of environment, and global-scale correlations are positive for bacterial communities in all environments. In contrast, archaeal communities show approximately equal frequencies of positive and negative correlations in individual data sets, and a positive pan-environmental correlation for archaea only emerges after limiting the analysis to samples with reported oxygen concentrations. These results provide empirical evidence that geochemistry modulates genome evolution and may have distinct effects on bacteria and archaea. IMPORTANCE The identification of environmental factors that influence the elemental composition of proteins has implications for understanding microbial evolution and biogeography. Millions of years of genome evolution may provide a route for protein sequences to attain incomplete equilibrium with their chemical environment. We developed new tests of this chemical adaptation hypothesis by analyzing trends of the carbon oxidation state of community reference proteomes for microbial communities in local- and global-scale redox gradients. The results provide evidence for widespread environmental shaping of the elemental composition of protein sequences at the community level and establish a rationale for using thermodynamic models as a window into geochemical effects on microbial community assembly and evolution.
Keywords: Eh–pH diagram; geochemistry; global analysis; oxidation state; protein evolution; redox potential; thermodynamics.
Conflict of interest statement
The authors declare no conflict of interest.
Figures
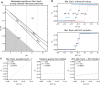
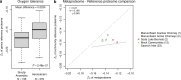
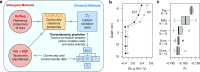
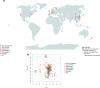
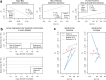
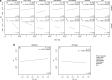
Similar articles
-
Archaeal and bacterial communities respond differently to environmental gradients in anoxic sediments of a California hypersaline lake, the Salton Sea.Appl Environ Microbiol. 2010 Feb;76(3):757-68. doi: 10.1128/AEM.02409-09. Epub 2009 Nov 30. Appl Environ Microbiol. 2010. PMID: 19948847 Free PMC article.
-
Chemical Links Between Redox Conditions and Estimated Community Proteomes from 16S rRNA and Reference Protein Sequences.Microb Ecol. 2023 May;85(4):1338-1355. doi: 10.1007/s00248-022-01988-9. Epub 2022 May 3. Microb Ecol. 2023. PMID: 35503575
-
Changes in northern Gulf of Mexico sediment bacterial and archaeal communities exposed to hypoxia.Geobiology. 2015 Sep;13(5):478-93. doi: 10.1111/gbi.12142. Epub 2015 May 5. Geobiology. 2015. PMID: 25939270
-
Functional microbial diversity explains groundwater chemistry in a pristine aquifer.BMC Microbiol. 2013 Jun 24;13:146. doi: 10.1186/1471-2180-13-146. BMC Microbiol. 2013. PMID: 23800252 Free PMC article.
-
Correlating microbial community profiles with geochemical data in highly stratified sediments from the Arctic Mid-Ocean Ridge.Proc Natl Acad Sci U S A. 2012 Oct 16;109(42):E2846-55. doi: 10.1073/pnas.1207574109. Epub 2012 Oct 1. Proc Natl Acad Sci U S A. 2012. PMID: 23027979 Free PMC article.
Cited by
-
chem16S: community-level chemical metrics for exploring genomic adaptation to environments.Bioinformatics. 2023 Sep 2;39(9):btad564. doi: 10.1093/bioinformatics/btad564. Bioinformatics. 2023. PMID: 37688565 Free PMC article.
-
Annotation-free prediction of microbial dioxygen utilization.mSystems. 2024 Oct 22;9(10):e0076324. doi: 10.1128/msystems.00763-24. Epub 2024 Sep 4. mSystems. 2024. PMID: 39230322 Free PMC article.
References
-
- Ren M, Feng X, Huang Y, Wang H, Hu Z, Clingenpeel S, Swan BK, Fonseca MM, Posada D, Stepanauskas R, Hollibaugh JT, Foster PG, Woyke T, Luo H. 2019. Phylogenomics suggests oxygen availability as a driving force in Thaumarchaeota evolution. ISME J 13:2150–2161. doi:10.1038/s41396-019-0418-8 - DOI - PMC - PubMed
-
- Algeo TJ, Li C. 2020. Redox classification and calibration of redox thresholds in sedimentary systems. Geochim Cosmochim Acta 287:8–26. doi:10.1016/j.gca.2020.01.055 - DOI
MeSH terms
Substances
Grants and funding
LinkOut - more resources
Full Text Sources