Multimodal AI for prediction of distant metastasis in carcinoma patients
- PMID: 37228671
- PMCID: PMC10203594
- DOI: 10.3389/fbinf.2023.1131021
Multimodal AI for prediction of distant metastasis in carcinoma patients
Abstract
Metastasis of cancer is directly related to death in almost all cases, however a lot is yet to be understood about this process. Despite advancements in the available radiological investigation techniques, not all cases of Distant Metastasis (DM) are diagnosed at initial clinical presentation. Also, there are currently no standard biomarkers of metastasis. Early, accurate diagnosis of DM is however crucial for clinical decision making, and planning of appropriate management strategies. Previous works have achieved little success in attempts to predict DM from either clinical, genomic, radiology, or histopathology data. In this work we attempt a multimodal approach to predict the presence of DM in cancer patients by combining gene expression data, clinical data and histopathology images. We tested a novel combination of Random Forest (RF) algorithm with an optimization technique for gene selection, and investigated if gene expression pattern in the primary tissues of three cancer types (Bladder Carcinoma, Pancreatic Adenocarcinoma, and Head and Neck Squamous Carcinoma) with DM are similar or different. Gene expression biomarkers of DM identified by our proposed method outperformed Differentially Expressed Genes (DEGs) identified by the DESeq2 software package in the task of predicting presence or absence of DM. Genes involved in DM tend to be more cancer type specific rather than general across all cancers. Our results also indicate that multimodal data is more predictive of metastasis than either of the three unimodal data tested, and genomic data provides the highest contribution by a wide margin. The results re-emphasize the importance for availability of sufficient image data when a weakly supervised training technique is used. Code is made available at: https://github.com/rit-cui-lab/Multimodal-AI-for-Prediction-of-Distant-Metastasis-in-Carcinoma-Patients.
Keywords: cancer; deep learning; gene expression; histopathology; machine learning; metastasis; multimodal.
Copyright © 2023 Olatunji and Cui.
Conflict of interest statement
The authors declare that the research was conducted in the absence of any commercial or financial relationships that could be construed as a potential conflict of interest.
Figures
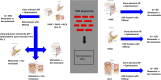
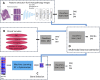
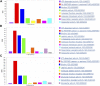
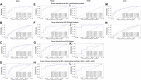
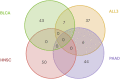
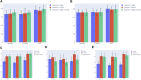
Similar articles
-
Erratum: Eyestalk Ablation to Increase Ovarian Maturation in Mud Crabs.J Vis Exp. 2023 May 26;(195). doi: 10.3791/6561. J Vis Exp. 2023. PMID: 37235796
-
Defining the optimum strategy for identifying adults and children with coeliac disease: systematic review and economic modelling.Health Technol Assess. 2022 Oct;26(44):1-310. doi: 10.3310/ZUCE8371. Health Technol Assess. 2022. PMID: 36321689 Free PMC article.
-
Comparison of Two Modern Survival Prediction Tools, SORG-MLA and METSSS, in Patients With Symptomatic Long-bone Metastases Who Underwent Local Treatment With Surgery Followed by Radiotherapy and With Radiotherapy Alone.Clin Orthop Relat Res. 2024 Dec 1;482(12):2193-2208. doi: 10.1097/CORR.0000000000003185. Epub 2024 Jul 23. Clin Orthop Relat Res. 2024. PMID: 39051924
-
Depressing time: Waiting, melancholia, and the psychoanalytic practice of care.In: Kirtsoglou E, Simpson B, editors. The Time of Anthropology: Studies of Contemporary Chronopolitics. Abingdon: Routledge; 2020. Chapter 5. In: Kirtsoglou E, Simpson B, editors. The Time of Anthropology: Studies of Contemporary Chronopolitics. Abingdon: Routledge; 2020. Chapter 5. PMID: 36137063 Free Books & Documents. Review.
-
Acute Intermittent Porphyria.2005 Sep 27 [updated 2024 Feb 8]. In: Adam MP, Feldman J, Mirzaa GM, Pagon RA, Wallace SE, Amemiya A, editors. GeneReviews® [Internet]. Seattle (WA): University of Washington, Seattle; 1993–2025. 2005 Sep 27 [updated 2024 Feb 8]. In: Adam MP, Feldman J, Mirzaa GM, Pagon RA, Wallace SE, Amemiya A, editors. GeneReviews® [Internet]. Seattle (WA): University of Washington, Seattle; 1993–2025. PMID: 20301372 Free Books & Documents. Review.
Cited by
-
Applications of Multimodal Artificial Intelligence in Non-Hodgkin Lymphoma B Cells.Biomedicines. 2024 Aug 5;12(8):1753. doi: 10.3390/biomedicines12081753. Biomedicines. 2024. PMID: 39200217 Free PMC article. Review.
-
Deep neural networks integrating genomics and histopathological images for predicting stages and survival time-to-event in colon cancer.PLoS One. 2024 Sep 3;19(9):e0305268. doi: 10.1371/journal.pone.0305268. eCollection 2024. PLoS One. 2024. PMID: 39226289 Free PMC article.
References
-
- Bednarek R., Selmi A., Wojkowska D., Karolczak K., Popielarski M., Stasiak M., et al. (2020). Functional inhibition of F11 receptor (F11R/junctional adhesion molecule-A/JAM-A) activity by a F11R-derived peptide in breast cancer and its microenvironment. Breast Cancer Res. Treat. 179 (2), 325–335. 10.1007/s10549-019-05471-x - DOI - PMC - PubMed
-
- Bitter E. E., Morris R. M., Mortimer T., Barlow K., Schekall A., Townsend M. H., et al. (2022). “The potential effects of thymidine kinase 1 on breast cancer invasion,” in Proceedings of the American association for cancer research annual meeting 2022 (Philadelphia (PA): AACR; Cancer Res; ).
Grants and funding
LinkOut - more resources
Full Text Sources
Miscellaneous