Accuracy of a machine learning method based on structural and locational information from AlphaFold2 for predicting the pathogenicity of TARDBP and FUS gene variants in ALS
- PMID: 37208601
- PMCID: PMC10197232
- DOI: 10.1186/s12859-023-05338-5
Accuracy of a machine learning method based on structural and locational information from AlphaFold2 for predicting the pathogenicity of TARDBP and FUS gene variants in ALS
Abstract
Background: In the sporadic form of amyotrophic lateral sclerosis (ALS), the pathogenicity of rare variants in the causative genes characterizing the familial form remains largely unknown. To predict the pathogenicity of such variants, in silico analysis is commonly used. In some ALS causative genes, the pathogenic variants are concentrated in specific regions, and the resulting alterations in protein structure are thought to significantly affect pathogenicity. However, existing methods have not taken this issue into account. To address this, we have developed a technique termed MOVA (method for evaluating the pathogenicity of missense variants using AlphaFold2), which applies positional information for structural variants predicted by AlphaFold2. Here we examined the utility of MOVA for analysis of several causative genes of ALS.
Methods: We analyzed variants of 12 ALS-related genes (TARDBP, FUS, SETX, TBK1, OPTN, SOD1, VCP, SQSTM1, ANG, UBQLN2, DCTN1, and CCNF) and classified them as pathogenic or neutral. For each gene, the features of the variants, consisting of their positions in the 3D structure predicted by AlphaFold2, pLDDT score, and BLOSUM62 were trained into a random forest and evaluated by the stratified fivefold cross validation method. We compared how accurately MOVA predicted mutant pathogenicity with other in silico prediction methods and evaluated the prediction accuracy at TARDBP and FUS hotspots. We also examined which of the MOVA features had the greatest impact on pathogenicity discrimination.
Results: MOVA yielded useful results (AUC ≥ 0.70) for TARDBP, FUS, SOD1, VCP, and UBQLN2 of 12 ALS causative genes. In addition, when comparing the prediction accuracy with other in silico prediction methods, MOVA obtained the best results among those compared for TARDBP, VCP, UBQLN2, and CCNF. MOVA demonstrated superior predictive accuracy for the pathogenicity of mutations at hotspots of TARDBP and FUS. Moreover, higher accuracy was achieved by combining MOVA with REVEL or CADD. Among the features of MOVA, the x, y, and z coordinates performed the best and were highly correlated with MOVA.
Conclusions: MOVA is useful for predicting the virulence of rare variants in which they are concentrated at specific structural sites, and for use in combination with other prediction methods.
Keywords: AlphaFold2; Amyotrophic lateral sclerosis; MOVA; Missense variant; Prediction tool.
© 2023. The Author(s).
Conflict of interest statement
There are no associations with companies or organizations that would constitute a conflict of interest requiring disclosure in relation to this study.
Figures
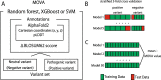
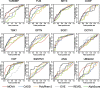
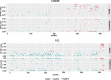
Similar articles
-
Screening of SOD1, FUS and TARDBP genes in patients with amyotrophic lateral sclerosis in central-southern China.Sci Rep. 2016 Sep 8;6:32478. doi: 10.1038/srep32478. Sci Rep. 2016. PMID: 27604643 Free PMC article.
-
SOD1, ANG, TARDBP and FUS mutations in amyotrophic lateral sclerosis: a United States clinical testing lab experience.Amyotroph Lateral Scler. 2012 Feb;13(2):217-22. doi: 10.3109/17482968.2011.643899. Amyotroph Lateral Scler. 2012. PMID: 22292843
-
Mutation analysis of SOD1, C9orf72, TARDBP and FUS genes in ethnically-diverse Malaysian patients with amyotrophic lateral sclerosis (ALS).Neurobiol Aging. 2021 Dec;108:200-206. doi: 10.1016/j.neurobiolaging.2021.07.008. Epub 2021 Jul 21. Neurobiol Aging. 2021. PMID: 34404558
-
The Occurrence of FUS Mutations in Pediatric Amyotrophic Lateral Sclerosis: A Case Report and Review of the Literature.J Child Neurol. 2020 Jul;35(8):556-562. doi: 10.1177/0883073820915099. Epub 2020 Apr 13. J Child Neurol. 2020. PMID: 32281455 Review.
-
Studies of Genetic and Proteomic Risk Factors of Amyotrophic Lateral Sclerosis Inspire Biomarker Development and Gene Therapy.Cells. 2023 Jul 27;12(15):1948. doi: 10.3390/cells12151948. Cells. 2023. PMID: 37566027 Free PMC article. Review.
Cited by
-
Challenges and limitations in computational prediction of protein misfolding in neurodegenerative diseases.Front Comput Neurosci. 2024 Jan 5;17:1323182. doi: 10.3389/fncom.2023.1323182. eCollection 2023. Front Comput Neurosci. 2024. PMID: 38250244 Free PMC article. No abstract available.
References
-
- Breiman L. Random forests. Mach Learn. 2001;45:5–32. doi: 10.1023/A:1010933404324. - DOI
MeSH terms
Substances
Grants and funding
LinkOut - more resources
Full Text Sources
Medical
Miscellaneous