Network Biology Analyses and Dynamic Modeling of Gene Regulatory Networks under Drought Stress Reveal Major Transcriptional Regulators in Arabidopsis
- PMID: 37108512
- PMCID: PMC10139068
- DOI: 10.3390/ijms24087349
Network Biology Analyses and Dynamic Modeling of Gene Regulatory Networks under Drought Stress Reveal Major Transcriptional Regulators in Arabidopsis
Abstract
Drought is one of the most serious abiotic stressors in the environment, restricting agricultural production by reducing plant growth, development, and productivity. To investigate such a complex and multifaceted stressor and its effects on plants, a systems biology-based approach is necessitated, entailing the generation of co-expression networks, identification of high-priority transcription factors (TFs), dynamic mathematical modeling, and computational simulations. Here, we studied a high-resolution drought transcriptome of Arabidopsis. We identified distinct temporal transcriptional signatures and demonstrated the involvement of specific biological pathways. Generation of a large-scale co-expression network followed by network centrality analyses identified 117 TFs that possess critical properties of hubs, bottlenecks, and high clustering coefficient nodes. Dynamic transcriptional regulatory modeling of integrated TF targets and transcriptome datasets uncovered major transcriptional events during the course of drought stress. Mathematical transcriptional simulations allowed us to ascertain the activation status of major TFs, as well as the transcriptional intensity and amplitude of their target genes. Finally, we validated our predictions by providing experimental evidence of gene expression under drought stress for a set of four TFs and their major target genes using qRT-PCR. Taken together, we provided a systems-level perspective on the dynamic transcriptional regulation during drought stress in Arabidopsis and uncovered numerous novel TFs that could potentially be used in future genetic crop engineering programs.
Keywords: Arabidopsis; co-expression network; computational simulation; drought; network centrality; systems biology; transcriptional regulation; water deprivation.
Conflict of interest statement
The authors declare no conflict of interest.
Figures
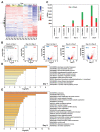
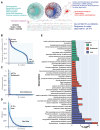
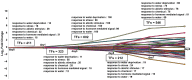
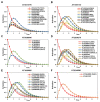
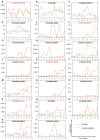
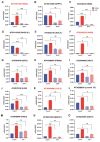
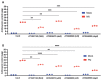
Similar articles
-
Abiotic stress induced miRNA-TF-gene regulatory network: A structural perspective.Genomics. 2020 Jan;112(1):412-422. doi: 10.1016/j.ygeno.2019.03.004. Epub 2019 Mar 12. Genomics. 2020. PMID: 30876925
-
Temporal transcriptional logic of dynamic regulatory networks underlying nitrogen signaling and use in plants.Proc Natl Acad Sci U S A. 2018 Jun 19;115(25):6494-6499. doi: 10.1073/pnas.1721487115. Epub 2018 May 16. Proc Natl Acad Sci U S A. 2018. PMID: 29769331 Free PMC article.
-
A transcriptional dynamic network during Arabidopsis thaliana pollen development.BMC Syst Biol. 2011;5 Suppl 3(Suppl 3):S8. doi: 10.1186/1752-0509-5-S3-S8. Epub 2011 Dec 23. BMC Syst Biol. 2011. PMID: 22784627 Free PMC article.
-
Insights into molecular links and transcription networks integrating drought stress and nitrogen signaling.New Phytol. 2024 Jan;241(2):560-566. doi: 10.1111/nph.19403. Epub 2023 Nov 16. New Phytol. 2024. PMID: 37974513 Review.
-
Transcriptome meta-analysis-based identification of hub transcription factors and RNA-binding proteins potentially orchestrating gene regulatory cascades and crosstalk in response to abiotic stresses in Arabidopsis thaliana.J Appl Genet. 2024 May;65(2):255-269. doi: 10.1007/s13353-024-00837-4. Epub 2024 Feb 10. J Appl Genet. 2024. PMID: 38337133 Review.
Cited by
-
Integrated Systems Biology Pipeline to Compare Co-Expression Networks in Plants and Elucidate Differential Regulators.Plants (Basel). 2023 Oct 19;12(20):3618. doi: 10.3390/plants12203618. Plants (Basel). 2023. PMID: 37896081 Free PMC article.
-
Profiling of Key Hub Genes Using a Two-State Weighted Gene Co-Expression Network of 'Jao Khao' Rice under Soil Salinity Stress Based on Time-Series Transcriptome Data.Int J Mol Sci. 2024 Oct 16;25(20):11086. doi: 10.3390/ijms252011086. Int J Mol Sci. 2024. PMID: 39456877 Free PMC article.
References
MeSH terms
Substances
Grants and funding
LinkOut - more resources
Full Text Sources
Miscellaneous