Statistical inference of the rates of cell proliferation and phenotypic switching in cancer
- PMID: 37087049
- PMCID: PMC10372878
- DOI: 10.1016/j.jtbi.2023.111497
Statistical inference of the rates of cell proliferation and phenotypic switching in cancer
Abstract
Recent evidence suggests that nongenetic (epigenetic) mechanisms play an important role at all stages of cancer evolution. In many cancers, these mechanisms have been observed to induce dynamic switching between two or more cell states, which commonly show differential responses to drug treatments. To understand how these cancers evolve over time, and how they respond to treatment, we need to understand the state-dependent rates of cell proliferation and phenotypic switching. In this work, we propose a rigorous statistical framework for estimating these parameters, using data from commonly performed cell line experiments, where phenotypes are sorted and expanded in culture. The framework explicitly models the stochastic dynamics of cell division, cell death and phenotypic switching, and it provides likelihood-based confidence intervals for the model parameters. The input data can be either the fraction of cells or the number of cells in each state at one or more time points. Through a combination of theoretical analysis and numerical simulations, we show that when cell fraction data is used, the rates of switching may be the only parameters that can be estimated accurately. On the other hand, using cell number data enables accurate estimation of the net division rate for each phenotype, and it can even enable estimation of the state-dependent rates of cell division and cell death. We conclude by applying our framework to a publicly available dataset.
Keywords: Cancer evolution; Epigenetics; Mathematical modeling; Maximum likelihood estimation; Parameter identifiability; Phenotypic switching.
Copyright © 2023 Elsevier Ltd. All rights reserved.
Conflict of interest statement
Declaration of Competing Interest The authors declare that they have no known competing financial interests or personal relationships that could have appeared to influence the work reported in this paper.
Figures
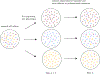
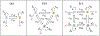
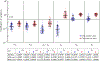
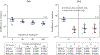
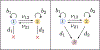
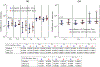

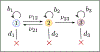
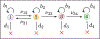
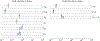
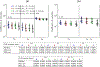
Update of
-
Statistical inference of the rates of cell proliferation and phenotypic switching in cancer.ArXiv [Preprint]. 2023 Jun 13:arXiv:2306.08096v1. ArXiv. 2023. Update in: J Theor Biol. 2023 Jul 7;568:111497. doi: 10.1016/j.jtbi.2023.111497 PMID: 37396613 Free PMC article. Updated. Preprint.
Similar articles
-
Statistical inference of the rates of cell proliferation and phenotypic switching in cancer.ArXiv [Preprint]. 2023 Jun 13:arXiv:2306.08096v1. ArXiv. 2023. Update in: J Theor Biol. 2023 Jul 7;568:111497. doi: 10.1016/j.jtbi.2023.111497 PMID: 37396613 Free PMC article. Updated. Preprint.
-
Reliable and efficient parameter estimation using approximate continuum limit descriptions of stochastic models.J Theor Biol. 2022 Sep 21;549:111201. doi: 10.1016/j.jtbi.2022.111201. Epub 2022 Jun 22. J Theor Biol. 2022. PMID: 35752285
-
Mutation, cell kinetics, and subpopulations at risk for colon cancer in the United States.Mutat Res. 1998 May 25;400(1-2):553-78. doi: 10.1016/s0027-5107(98)00067-0. Mutat Res. 1998. PMID: 9685710
-
Developing Statistical Methods to Improve Stepped-Wedge Cluster Randomized Trials [Internet].Washington (DC): Patient-Centered Outcomes Research Institute (PCORI); 2021 Aug. Washington (DC): Patient-Centered Outcomes Research Institute (PCORI); 2021 Aug. PMID: 38913814 Free Books & Documents. Review.
-
Dynamical hallmarks of cancer: Phenotypic switching in melanoma and epithelial-mesenchymal plasticity.Semin Cancer Biol. 2023 Nov;96:48-63. doi: 10.1016/j.semcancer.2023.09.007. Epub 2023 Oct 1. Semin Cancer Biol. 2023. PMID: 37788736 Review.
Cited by
-
Using birth-death processes to infer tumor subpopulation structure from live-cell imaging drug screening data.PLoS Comput Biol. 2024 Mar 6;20(3):e1011888. doi: 10.1371/journal.pcbi.1011888. eCollection 2024 Mar. PLoS Comput Biol. 2024. PMID: 38446830 Free PMC article.
References
-
- Brock Amy, Chang Hannah, and Huang Sui. Non-genetic heterogeneity - a mutation-independent driving force for the somatic evolution of tumours. Nature Reviews Genetics, 10(5):336, 2009. - PubMed
-
- Brown Robert, Curry Edward, Magnani Luca, Charlotte S Wilhelm-Benartzi, and Jane Borley. Poised epigenetic states and acquired drug resistance in cancer. Nature Reviews Cancer, 14(11):747, 2014. - PubMed
Publication types
MeSH terms
Grants and funding
LinkOut - more resources
Full Text Sources