This is a preprint.
Mathematical modeling suggests cytotoxic T lymphocytes control growth of B16 tumor cells in collagin-fibrin gels by cytolytic and non-lytic mechanisms
- PMID: 37034693
- PMCID: PMC10081166
- DOI: 10.1101/2023.03.28.534600
Mathematical modeling suggests cytotoxic T lymphocytes control growth of B16 tumor cells in collagin-fibrin gels by cytolytic and non-lytic mechanisms
Update in
-
Cytotoxic T Lymphocytes Control Growth of B16 Tumor Cells in Collagen-Fibrin Gels by Cytolytic and Non-Lytic Mechanisms.Viruses. 2023 Jun 27;15(7):1454. doi: 10.3390/v15071454. Viruses. 2023. PMID: 37515143 Free PMC article.
Abstract
Cytotoxic T lymphocytes (CTLs) are important in controlling some viral infections, and therapies involving transfer of large numbers of cancer-specific CTLs have been successfully used to treat several types of cancers in humans. While molecular mechanisms of how CTLs kill their targets are relatively well understood we still lack solid quantitative understanding of the kinetics and efficiency at which CTLs kill their targets in different conditions. Collagen-fibrin gel-based assays provide a tissue-like environment for the migration of CTLs, making them an attractive system to study the cytotoxicity in vitro. Budhu et al. [1] systematically varied the number of peptide (SIINFEKL)- pulsed B16 melanoma cells and SIINFEKL-specific CTLs (OT-1) and measured remaining targets at different times after target and CTL co-inoculation into collagen-fibrin gels. The authors proposed that their data were consistent with a simple model in which tumors grow exponentially and are killed by CTLs at a per capita rate proportional to the CTL density in the gel. By fitting several alternative mathematical models to these data we found that this simple "exponential-growth-mass-action-killing" model does not precisely fit the data. However, determining the best fit model proved difficult because the best performing model was dependent on the specific dataset chosen for the analysis. When considering all data that include biologically realistic CTL concentrations ( E ≤ 10 7 cell/ml) the model in which tumors grow exponentially and CTLs suppress tumor's growth non-lytically and kill tumors according to the mass-action law (SiGMA model) fitted the data with best quality. Results of power analysis suggested that longer experiments (∼ 3 - 4 days) with 4 measurements of B16 tumor cell concentrations for a range of CTL concentrations would best allow to discriminate between alternative models. Taken together, our results suggest that interactions between tumors and CTLs in collagen-fibrin gels are more complex than a simple exponential-growth- mass-action killing model and provide support for the hypothesis that CTLs impact on tumors may go beyond direct cytotoxicity.
Figures
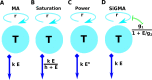
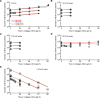
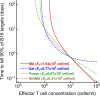
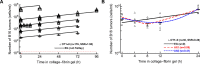
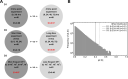
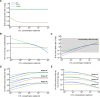
Similar articles
-
Cytotoxic T Lymphocytes Control Growth of B16 Tumor Cells in Collagen-Fibrin Gels by Cytolytic and Non-Lytic Mechanisms.Viruses. 2023 Jun 27;15(7):1454. doi: 10.3390/v15071454. Viruses. 2023. PMID: 37515143 Free PMC article.
-
Adoptive transfer of natural killer cells promotes the anti-tumor efficacy of T cells.Clin Immunol. 2017 Apr;177:76-86. doi: 10.1016/j.clim.2016.06.013. Epub 2016 Jul 1. Clin Immunol. 2017. PMID: 27377534 Free PMC article.
-
Tracking in vivo migration and distribution of antigen-specific cytotoxic T lymphocytes by 5,6-carboxyfluorescein diacetate succinimidyl ester staining during cancer immunotherapy.Chin Med J (Engl). 2013 Aug;126(16):3019-25. Chin Med J (Engl). 2013. PMID: 23981604
-
Molecular mechanisms and biological significance of CTL avidity.Curr HIV Res. 2003 Jul;1(3):287-94. doi: 10.2174/1570162033485230. Curr HIV Res. 2003. PMID: 15046253 Review.
-
What do mathematical models tell us about killing rates during HIV-1 infection?Immunol Lett. 2015 Nov;168(1):1-6. doi: 10.1016/j.imlet.2015.07.009. Epub 2015 Aug 13. Immunol Lett. 2015. PMID: 26279491 Review.
References
-
- Budhu S., Loike J. D., Pandolfi A., Han S., Catalano G., Constantinescu A., Clynes R. & Silverstein S. C. 2010. CD8+ T cell concentration determines their efficiency in killing cognate antigen-expressing syngeneic mammalian cells in vitro and in mouse tissues. J Exp Med, 207(1), 223–35. - PMC - PubMed
-
- Halle S., Keyser K. A., Stahl F. R., Busche A., Marquardt A., Zheng X., Galla M., Heissmeyer V., Heller K. et al. 2016. In vivo killing capacity of cytotoxic T cells is limited and involves dynamic interactions and T cell cooperativity. Immunity, 44(2), 233–245. doi: 10.1016/j.immuni.2016.01.010. - DOI - PMC - PubMed
Publication types
Grants and funding
LinkOut - more resources
Full Text Sources