A risk prediction model based on machine learning for early cognitive impairment in hypertension: Development and validation study
- PMID: 36969637
- PMCID: PMC10034177
- DOI: 10.3389/fpubh.2023.1143019
A risk prediction model based on machine learning for early cognitive impairment in hypertension: Development and validation study
Abstract
Background: Clinical practice guidelines recommend early identification of cognitive impairment in individuals with hypertension with the help of risk prediction tools based on risk factors.
Objective: The aim of this study was to develop a superior machine learning model based on easily collected variables to predict the risk of early cognitive impairment in hypertensive individuals, which could be used to optimize early cognitive impairment risk assessment strategies.
Methods: For this cross-sectional study, 733 patients with hypertension (aged 30-85, 48.98% male) enrolled in multi-center hospitals in China were divided into a training group (70%) and a validation group (30%). After least absolute shrinkage and selection operator (LASSO) regression analysis with 5-fold cross-validation determined the modeling variables, three machine learning classifiers, logistic regression (LR), XGBoost (XGB), and gaussian naive bayes (GNB), were developed. The area under the ROC curve (AUC), accuracy, sensitivity, specificity, and F1 score were used to evaluate the model performance. Shape Additive explanation (SHAP) analysis was performed to rank feature importance. Further decision curve analysis (DCA) assessed the clinical performance of the established model and visualized it by nomogram.
Results: Hip circumference, age, education levels, and physical activity were considered significant predictors of early cognitive impairment in hypertension. The AUC (0.88), F1 score (0.59), accuracy (0.81), sensitivity (0.84), and specificity (0.80) of the XGB model were superior to LR and GNB classifiers.
Conclusion: The XGB model based on hip circumference, age, educational level, and physical activity has superior predictive performance and it shows promise in predicting the risk of cognitive impairment in hypertensive clinical settings.
Keywords: cognitive impairment; hypertension; machine learning; prediction model; risk factors.
Copyright © 2023 Zhong, Yu, Jiang, Chen, Wang, Teng and Jiao.
Conflict of interest statement
The authors declare that the research was conducted in the absence of any commercial or financial relationships that could be construed as a potential conflict of interest.
Figures
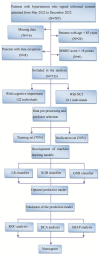
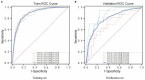
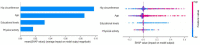
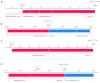
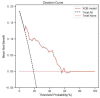
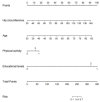
Similar articles
-
A Risk Prediction Model for Physical Restraints Among Older Chinese Adults in Long-term Care Facilities: Machine Learning Study.J Med Internet Res. 2023 Apr 6;25:e43815. doi: 10.2196/43815. J Med Internet Res. 2023. PMID: 37023416 Free PMC article.
-
Development and validation of the eMCI-CHD tool: A multivariable prediction model for the risk of mild cognitive impairment in patients with coronary heart disease.J Evid Based Med. 2024 Sep;17(3):535-549. doi: 10.1111/jebm.12632. Epub 2024 Aug 6. J Evid Based Med. 2024. PMID: 39107928
-
Prediction and validation of mild cognitive impairment in occupational dust exposure population based on machine learning.Ecotoxicol Environ Saf. 2024 Oct 15;285:117111. doi: 10.1016/j.ecoenv.2024.117111. Epub 2024 Sep 26. Ecotoxicol Environ Saf. 2024. PMID: 39332198
-
Evaluate prognostic accuracy of SOFA component score for mortality among adults with sepsis by machine learning method.BMC Infect Dis. 2023 Feb 6;23(1):76. doi: 10.1186/s12879-023-08045-x. BMC Infect Dis. 2023. PMID: 36747139 Free PMC article. Clinical Trial.
-
Prediction of subjective cognitive decline after corpus callosum infarction by an interpretable machine learning-derived early warning strategy.Front Neurol. 2023 Jun 9;14:1123607. doi: 10.3389/fneur.2023.1123607. eCollection 2023. Front Neurol. 2023. PMID: 37416313 Free PMC article.
Cited by
-
Development and Validation of the Communities Geriatric Mild Cognitive Impairment Risk Calculator (CGMCI-Risk).Healthcare (Basel). 2024 Oct 10;12(20):2015. doi: 10.3390/healthcare12202015. Healthcare (Basel). 2024. PMID: 39451430 Free PMC article.
-
Application of machine learning in measurement of ageing and geriatric diseases: a systematic review.BMC Geriatr. 2023 Dec 12;23(1):841. doi: 10.1186/s12877-023-04477-x. BMC Geriatr. 2023. PMID: 38087195 Free PMC article.
-
Association between intensity of physical activity and cognitive function in hypertensive patients: a case-control study.Sci Rep. 2024 May 2;14(1):10106. doi: 10.1038/s41598-024-59457-x. Sci Rep. 2024. PMID: 38697999 Free PMC article.
-
Predictive Value of the Total Bilirubin and CA50 Screened Based on Machine Learning for Recurrence of Bladder Cancer Patients.Cancer Manag Res. 2024 May 31;16:537-546. doi: 10.2147/CMAR.S457269. eCollection 2024. Cancer Manag Res. 2024. PMID: 38835478 Free PMC article.
-
Increased extracellular water/body mass is associated with functional impairment in hemodialysis patients.Ren Fail. 2023;45(2):2271066. doi: 10.1080/0886022X.2023.2271066. Epub 2023 Nov 21. Ren Fail. 2023. PMID: 38532722 Free PMC article.
References
Publication types
MeSH terms
Grants and funding
LinkOut - more resources
Full Text Sources
Medical