Validation of a stereological method for estimating particle size and density from 2D projections with high accuracy
- PMID: 36930689
- PMCID: PMC10022809
- DOI: 10.1371/journal.pone.0277148
Validation of a stereological method for estimating particle size and density from 2D projections with high accuracy
Abstract
Stereological methods for estimating the 3D particle size and density from 2D projections are essential to many research fields. These methods are, however, prone to errors arising from undetected particle profiles due to sectioning and limited resolution, known as 'lost caps'. A potential solution developed by Keiding, Jensen, and Ranek in 1972, which we refer to as the Keiding model, accounts for lost caps by quantifying the smallest detectable profile in terms of its limiting 'cap angle' (ϕ), a size-independent measure of a particle's distance from the section surface. However, this simple solution has not been widely adopted nor tested. Rather, model-independent design-based stereological methods, which do not explicitly account for lost caps, have come to the fore. Here, we provide the first experimental validation of the Keiding model by comparing the size and density of particles estimated from 2D projections with direct measurement from 3D EM reconstructions of the same tissue. We applied the Keiding model to estimate the size and density of somata, nuclei and vesicles in the cerebellum of mice and rats, where high packing density can be problematic for design-based methods. Our analysis reveals a Gaussian distribution for ϕ rather than a single value. Nevertheless, curve fits of the Keiding model to the 2D diameter distribution accurately estimate the mean ϕ and 3D diameter distribution. While systematic testing using simulations revealed an upper limit to determining ϕ, our analysis shows that estimated ϕ can be used to determine the 3D particle density from the 2D density under a wide range of conditions, and this method is potentially more accurate than minimum-size-based lost-cap corrections and disector methods. Our results show the Keiding model provides an efficient means of accurately estimating the size and density of particles from 2D projections even under conditions of a high density.
Copyright: © 2023 Rothman et al. This is an open access article distributed under the terms of the Creative Commons Attribution License, which permits unrestricted use, distribution, and reproduction in any medium, provided the original author and source are credited.
Conflict of interest statement
The authors have declared that no competing interests exist.
Figures
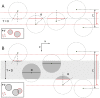
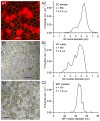
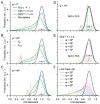
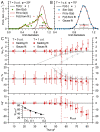
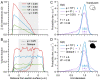
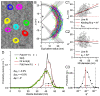
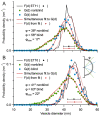
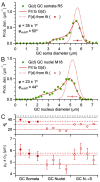
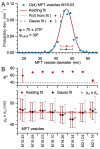
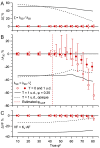
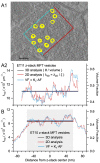
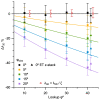
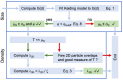
Similar articles
-
Estimation of the numerical density of synapses in rat neocortex. Comparison of the 'disector' with an 'unfolding' method.J Neurosci Methods. 1988 Apr;23(3):195-205. doi: 10.1016/0165-0270(88)90003-9. J Neurosci Methods. 1988. PMID: 3367656
-
Particle number can be estimated using a disector of unknown thickness: the selector.J Microsc. 1987 Feb;145(Pt 2):121-42. J Microsc. 1987. PMID: 3553604
-
A brief update on physical and optical disector applications and sectioning-staining methods in neuroscience.J Chem Neuroanat. 2018 Nov;93:16-29. doi: 10.1016/j.jchemneu.2018.02.009. Epub 2018 Feb 26. J Chem Neuroanat. 2018. PMID: 29496551 Review.
-
Development and application of an aerosol screening model for size-resolved urban aerosols.Res Rep Health Eff Inst. 2014 Jun;(179):3-79. Res Rep Health Eff Inst. 2014. PMID: 25145039
-
Applications of various stereological tools for estimation of biological tissues.Anat Histol Embryol. 2023 Mar;52(2):127-134. doi: 10.1111/ahe.12896. Epub 2022 Dec 23. Anat Histol Embryol. 2023. PMID: 36562319 Review.
References
Publication types
MeSH terms
Grants and funding
LinkOut - more resources
Full Text Sources
Miscellaneous