Integrated transcriptome study of the tumor microenvironment for treatment response prediction in male predominant hypopharyngeal carcinoma
- PMID: 36928331
- PMCID: PMC10020474
- DOI: 10.1038/s41467-023-37159-8
Integrated transcriptome study of the tumor microenvironment for treatment response prediction in male predominant hypopharyngeal carcinoma
Abstract
The efficacy of the first-line treatment for hypopharyngeal carcinoma (HPC), a predominantly male cancer, at advanced stage is only about 50% without reliable molecular indicators for its prognosis. In this study, HPC biopsy samples collected before and after the first-line treatment are classified into different groups according to treatment responses. We analyze the changes of HPC tumor microenvironment (TME) at the single-cell level in response to the treatment and identify three gene modules associated with advanced HPC prognosis. We estimate cell constitutions based on bulk RNA-seq of our HPC samples and build a binary classifier model based on non-malignant cell subtype abundance in TME, which can be used to accurately identify treatment-resistant advanced HPC patients in time and enlarge the possibility to preserve their laryngeal function. In summary, we provide a useful approach to identify gene modules and a classifier model as reliable indicators to predict treatment responses in HPC.
© 2023. The Author(s).
Conflict of interest statement
Two patents based on the study were submitted by Z.X., Y.Z., G.L., and M.T., which were entitled as “Featured gene sets based on scRNA-seq profiles of malignant tumor cells for the prognosis prediction of hypopharyngeal carcinoma” (application number, no 202310103758.7) and “A prediction method of the therapeutic efficacy for the combined treatment in advanced hypopharyngeal carcinoma based on the integration of single-cell and bulk transcriptome profiles” (application number, no 202310106947.X). The remaining authors declare no competing interests.
Figures
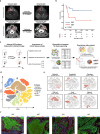
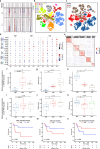
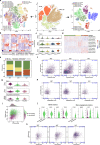
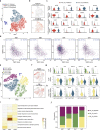
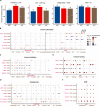
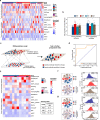
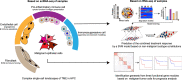
Similar articles
-
Upregulation of microRNA‑194‑5p inhibits hypopharyngeal carcinoma cell proliferation, migration and invasion by targeting SMURF1 via the mTOR signaling pathway.Int J Oncol. 2019 Apr;54(4):1245-1255. doi: 10.3892/ijo.2019.4711. Epub 2019 Feb 4. Int J Oncol. 2019. PMID: 30720112 Free PMC article.
-
Fibrinogen and Neutrophil-to-lymphocyte Ratio Predicts Survival in Patients with Advanced Hypopharyngeal Squamous Cell Carcinoma.Anticancer Res. 2018 Sep;38(9):5321-5330. doi: 10.21873/anticanres.12859. Anticancer Res. 2018. PMID: 30194184
-
APE1 facilitates PD-L1-mediated progression of laryngeal and hypopharyngeal squamous cell carcinoma.Int Immunopharmacol. 2021 Aug;97:107675. doi: 10.1016/j.intimp.2021.107675. Epub 2021 May 6. Int Immunopharmacol. 2021. PMID: 33964809
-
Lentivirus-Mediated Short Hairpin RNA for Follistatin Downregulation Suppresses Tumor Progression in Hypopharyngeal Carcinoma.Curr Med Sci. 2022 Aug;42(4):832-840. doi: 10.1007/s11596-022-2615-3. Epub 2022 Jul 21. Curr Med Sci. 2022. PMID: 35864413
-
Treatment of hypopharyngeal carcinoma with primary chemoradiotherapy: functional morbidity.Curr Opin Otolaryngol Head Neck Surg. 2012 Apr;20(2):89-96. doi: 10.1097/MOO.0b013e32834fa72c. Curr Opin Otolaryngol Head Neck Surg. 2012. PMID: 22249169 Review.
Cited by
-
New perspectives on biology, disease progression, and therapy response of head and neck cancer gained from single cell RNA sequencing and spatial transcriptomics.Oncol Res. 2023 Nov 15;32(1):1-17. doi: 10.32604/or.2023.044774. eCollection 2023. Oncol Res. 2023. PMID: 38188682 Free PMC article. Review.
-
Spatial transcriptomics in glioblastoma: is knowing the right zip code the key to the next therapeutic breakthrough?Front Oncol. 2023 Oct 17;13:1266397. doi: 10.3389/fonc.2023.1266397. eCollection 2023. Front Oncol. 2023. PMID: 37916170 Free PMC article. Review.
-
Influencing Factors of Survival in Hypopharyngeal Squamous Cell Cancer.J Clin Med. 2024 Jun 30;13(13):3853. doi: 10.3390/jcm13133853. J Clin Med. 2024. PMID: 38999419 Free PMC article.
-
Single-cell transcriptomes identify patient-tailored therapies for selective co-inhibition of cancer clones.Nat Commun. 2024 Oct 3;15(1):8579. doi: 10.1038/s41467-024-52980-5. Nat Commun. 2024. PMID: 39362905 Free PMC article.
References
-
- Bradley PJ. Epidemiology of hypopharyngeal cancer. Adv. Otorhinolaryngol. 2019;83:1–14. - PubMed
Publication types
MeSH terms
LinkOut - more resources
Full Text Sources