Diagnostic accuracy of automated ACR BI-RADS breast density classification using deep convolutional neural networks
- PMID: 36856841
- PMCID: PMC10289992
- DOI: 10.1007/s00330-023-09474-7
Diagnostic accuracy of automated ACR BI-RADS breast density classification using deep convolutional neural networks
Abstract
Objectives: High breast density is a well-known risk factor for breast cancer. This study aimed to develop and adapt two (MLO, CC) deep convolutional neural networks (DCNN) for automatic breast density classification on synthetic 2D tomosynthesis reconstructions.
Methods: In total, 4605 synthetic 2D images (1665 patients, age: 57 ± 37 years) were labeled according to the ACR (American College of Radiology) density (A-D). Two DCNNs with 11 convolutional layers and 3 fully connected layers each, were trained with 70% of the data, whereas 20% was used for validation. The remaining 10% were used as a separate test dataset with 460 images (380 patients). All mammograms in the test dataset were read blinded by two radiologists (reader 1 with two and reader 2 with 11 years of dedicated mammographic experience in breast imaging), and the consensus was formed as the reference standard. The inter- and intra-reader reliabilities were assessed by calculating Cohen's kappa coefficients, and diagnostic accuracy measures of automated classification were evaluated.
Results: The two models for MLO and CC projections had a mean sensitivity of 80.4% (95%-CI 72.2-86.9), a specificity of 89.3% (95%-CI 85.4-92.3), and an accuracy of 89.6% (95%-CI 88.1-90.9) in the differentiation between ACR A/B and ACR C/D. DCNN versus human and inter-reader agreement were both "substantial" (Cohen's kappa: 0.61 versus 0.63).
Conclusion: The DCNN allows accurate, standardized, and observer-independent classification of breast density based on the ACR BI-RADS system.
Key points: • A DCNN performs on par with human experts in breast density assessment for synthetic 2D tomosynthesis reconstructions. • The proposed technique may be useful for accurate, standardized, and observer-independent breast density evaluation of tomosynthesis.
Keywords: Breast density; Breast neoplasms; Deep learning; Mammography; Risk factors.
© 2023. The Author(s).
Conflict of interest statement
The authors of this manuscript declare no relationships with any companies whose products or services may be related to the subject matter of the article.
Figures
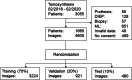
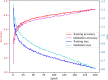
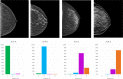
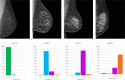
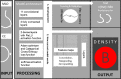
Similar articles
-
Determination of mammographic breast density using a deep convolutional neural network.Br J Radiol. 2019 Jan;92(1093):20180691. doi: 10.1259/bjr.20180691. Epub 2018 Oct 1. Br J Radiol. 2019. PMID: 30209957 Free PMC article.
-
Automatic classification of ultrasound breast lesions using a deep convolutional neural network mimicking human decision-making.Eur Radiol. 2019 Oct;29(10):5458-5468. doi: 10.1007/s00330-019-06118-7. Epub 2019 Mar 29. Eur Radiol. 2019. PMID: 30927100
-
Automated pectoral muscle identification on MLO-view mammograms: Comparison of deep neural network to conventional computer vision.Med Phys. 2019 May;46(5):2103-2114. doi: 10.1002/mp.13451. Epub 2019 Mar 12. Med Phys. 2019. PMID: 30771257 Free PMC article.
-
Understanding Clinical Mammographic Breast Density Assessment: a Deep Learning Perspective.J Digit Imaging. 2018 Aug;31(4):387-392. doi: 10.1007/s10278-017-0022-2. J Digit Imaging. 2018. PMID: 28932980 Free PMC article.
-
Supplemental Screening for Breast Cancer in Women With Dense Breasts: A Systematic Review for the U.S. Preventive Service Task Force [Internet].Rockville (MD): Agency for Healthcare Research and Quality (US); 2016 Jan. Report No.: 14-05201-EF-3. Rockville (MD): Agency for Healthcare Research and Quality (US); 2016 Jan. Report No.: 14-05201-EF-3. PMID: 26866210 Free Books & Documents. Review.
Cited by
-
Inter- and intra-observer variability of qualitative visual breast-composition assessment in mammography among Japanese physicians: a first multi-institutional observer performance study in Japan.Breast Cancer. 2024 Jul;31(4):671-683. doi: 10.1007/s12282-024-01580-8. Epub 2024 Apr 15. Breast Cancer. 2024. PMID: 38619787
-
Artificial Intelligence Applications in Breast Imaging: Current Status and Future Directions.Diagnostics (Basel). 2023 Jun 13;13(12):2041. doi: 10.3390/diagnostics13122041. Diagnostics (Basel). 2023. PMID: 37370936 Free PMC article. Review.
References
MeSH terms
LinkOut - more resources
Full Text Sources
Medical