A Machine Learning Model to Estimate Toxicokinetic Half-Lives of Per- and Polyfluoro-Alkyl Substances (PFAS) in Multiple Species
- PMID: 36850973
- PMCID: PMC9962572
- DOI: 10.3390/toxics11020098
A Machine Learning Model to Estimate Toxicokinetic Half-Lives of Per- and Polyfluoro-Alkyl Substances (PFAS) in Multiple Species
Abstract
Per- and polyfluoroalkyl substances (PFAS) are a diverse group of man-made chemicals that are commonly found in body tissues. The toxicokinetics of most PFAS are currently uncharacterized, but long half-lives (t½) have been observed in some cases. Knowledge of chemical-specific t½ is necessary for exposure reconstruction and extrapolation from toxicological studies. We used an ensemble machine learning method, random forest, to model the existing in vivo measured t½ across four species (human, monkey, rat, mouse) and eleven PFAS. Mechanistically motivated descriptors were examined, including two types of surrogates for renal transporters: (1) physiological descriptors, including kidney geometry, for renal transporter expression and (2) structural similarity of defluorinated PFAS to endogenous chemicals for transporter affinity. We developed a classification model for t½ (Bin 1: <12 h; Bin 2: <1 week; Bin 3: <2 months; Bin 4: >2 months). The model had an accuracy of 86.1% in contrast to 32.2% for a y-randomized null model. A total of 3890 compounds were within domain of the model, and t½ was predicted using the bin medians: 4.9 h, 2.2 days, 33 days, and 3.3 years. For human t½, 56% of PFAS were classified in Bin 4, 7% were classified in Bin 3, and 37% were classified in Bin 2. This model synthesizes the limited available data to allow tentative extrapolation and prioritization.
Keywords: PFAS; half-life; machine learning model; perfluoro-alkyl substances; toxicokinetics.
Conflict of interest statement
The authors declare no conflict of interest.
Figures
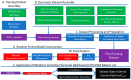
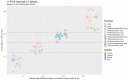
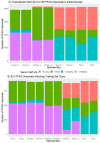
Similar articles
-
A Scoping Assessment of Implemented Toxicokinetic Models of Per- and Polyfluoro-Alkyl Substances, with a Focus on One-Compartment Models.Toxics. 2023 Feb 9;11(2):163. doi: 10.3390/toxics11020163. Toxics. 2023. PMID: 36851038 Free PMC article. Review.
-
A State-of-the-Science Review of Interactions of Per- and Polyfluoroalkyl Substances (PFAS) with Renal Transporters in Health and Disease: Implications for Population Variability in PFAS Toxicokinetics.Environ Health Perspect. 2023 Jul;131(7):76002. doi: 10.1289/EHP11885. Epub 2023 Jul 7. Environ Health Perspect. 2023. PMID: 37418334 Free PMC article. Review.
-
Modeling the transplacental transfer of small molecules using machine learning: a case study on per- and polyfluorinated substances (PFAS).J Expo Sci Environ Epidemiol. 2022 Nov;32(6):808-819. doi: 10.1038/s41370-022-00481-2. Epub 2022 Oct 7. J Expo Sci Environ Epidemiol. 2022. PMID: 36207486 Free PMC article.
-
Estimation of per- and polyfluoroalkyl substances (PFAS) half-lives in human studies: a systematic review and meta-analysis.Environ Res. 2024 Feb 1;242:117743. doi: 10.1016/j.envres.2023.117743. Epub 2023 Nov 25. Environ Res. 2024. PMID: 38008199 Review.
-
Towards process-based modelling and parameterisation of bioaccumulation in humans across PFAS congeners.Chemosphere. 2024 Jul;359:142253. doi: 10.1016/j.chemosphere.2024.142253. Epub 2024 May 5. Chemosphere. 2024. PMID: 38714250
Cited by
-
PFAS levels in paired drinking water and serum samples collected from an exposed community in Central North Carolina.Sci Total Environ. 2023 Oct 15;895:165091. doi: 10.1016/j.scitotenv.2023.165091. Epub 2023 Jun 23. Sci Total Environ. 2023. PMID: 37355130 Free PMC article.
-
A multi-tiered hierarchical Bayesian approach to derive toxic equivalency factors for dioxin-like compounds.Regul Toxicol Pharmacol. 2023 Sep;143:105464. doi: 10.1016/j.yrtph.2023.105464. Epub 2023 Jul 27. Regul Toxicol Pharmacol. 2023. PMID: 37516304 Free PMC article.
-
A Scoping Assessment of Implemented Toxicokinetic Models of Per- and Polyfluoro-Alkyl Substances, with a Focus on One-Compartment Models.Toxics. 2023 Feb 9;11(2):163. doi: 10.3390/toxics11020163. Toxics. 2023. PMID: 36851038 Free PMC article. Review.
-
Factors associated with per- and polyfluoroalkyl substances (PFAS) serum concentrations in residents of New Hanover County, North Carolina: The GenX exposure study.Environ Res. 2023 Nov 15;237(Pt 2):117020. doi: 10.1016/j.envres.2023.117020. Epub 2023 Sep 4. Environ Res. 2023. PMID: 37673120 Free PMC article.
-
Risk-based prioritization of PFAS using phenotypic and transcriptomic data from human induced pluripotent stem cell-derived hepatocytes and cardiomyocytes.ALTEX. 2024;41(3):363-381. doi: 10.14573/altex.2311031. Epub 2024 Feb 22. ALTEX. 2024. PMID: 38429992 Free PMC article.
References
-
- DeWitt J.C. Toxicological Effects of Perfluoroalkyl and Polyfluoroalkyl Substances. Springer; Berlin/Heidelberg, Germany: 2015.
-
- Buck R.C., Murphy P.M., Pabon M. Polyfluorinated Chemicals and Transformation Products. Springer; Berlin/Heidelberg, Germany: 2012. Chemistry, properties, and uses of commercial fluorinated surfactants; pp. 1–24.
-
- Rao N.S., Baker B.E. Organofluorine Chemistry. Springer; Berlin/Heidelberg, Germany: 1994. Textile finishes and fluorosurfactants; pp. 321–338.
-
- Rayne S., Forest K., Friesen K.J. Congener-specific numbering systems for the environmentally relevant C4 through C8 perfluorinated homologue groups of alkyl sulfonates, carboxylates, telomer alcohols, olefins, and acids, and their derivatives. J. Environ. Sci. Health Part A. 2008;43:1391–1401. doi: 10.1080/10934520802232030. - DOI - PubMed
Grants and funding
LinkOut - more resources
Full Text Sources