On predictive inference for intractable models via approximate Bayesian computation
- PMID: 36785730
- PMCID: PMC9911513
- DOI: 10.1007/s11222-022-10163-6
On predictive inference for intractable models via approximate Bayesian computation
Abstract
Approximate Bayesian computation (ABC) is commonly used for parameter estimation and model comparison for intractable simulator-based statistical models whose likelihood function cannot be evaluated. In this paper we instead investigate the feasibility of ABC as a generic approximate method for predictive inference, in particular, for computing the posterior predictive distribution of future observations or missing data of interest. We consider three complementary ABC approaches for this goal, each based on different assumptions regarding which predictive density of the intractable model can be sampled from. The case where only simulation from the joint density of the observed and future data given the model parameters can be used for inference is given particular attention and it is shown that the ideal summary statistic in this setting is minimal predictive sufficient instead of merely minimal sufficient (in the ordinary sense). An ABC prediction approach that takes advantage of a certain latent variable representation is also investigated. We additionally show how common ABC sampling algorithms can be used in the predictive settings considered. Our main results are first illustrated by using simple time-series models that facilitate analytical treatment, and later by using two common intractable dynamic models.
Supplementary information: The online version contains supplementary material available at 10.1007/s11222-022-10163-6.
Keywords: Approximate Bayesian computation; Intractable dynamic models; Posterior predictive distribution; Predictive sufficiency; Selection of summary statistics.
© The Author(s) 2023.
Figures
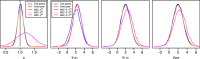
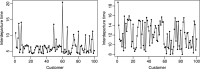
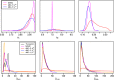
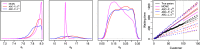
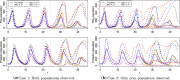
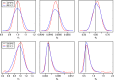
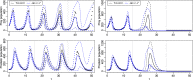
Similar articles
-
Piecewise Approximate Bayesian Computation: fast inference for discretely observed Markov models using a factorised posterior distribution.Stat Comput. 2015;25(2):289-301. doi: 10.1007/s11222-013-9432-2. Epub 2013 Nov 29. Stat Comput. 2015. PMID: 26097293 Free PMC article.
-
Parameter Estimation of Platelets Deposition: Approximate Bayesian Computation With High Performance Computing.Front Physiol. 2018 Aug 20;9:1128. doi: 10.3389/fphys.2018.01128. eCollection 2018. Front Physiol. 2018. PMID: 30177886 Free PMC article.
-
AABC: approximate approximate Bayesian computation for inference in population-genetic models.Theor Popul Biol. 2015 Feb;99:31-42. doi: 10.1016/j.tpb.2014.09.002. Epub 2014 Sep 26. Theor Popul Biol. 2015. PMID: 25261426 Free PMC article.
-
On the use of kernel approximate Bayesian computation to infer population history.Genes Genet Syst. 2015;90(3):153-62. doi: 10.1266/ggs.90.153. Genes Genet Syst. 2015. PMID: 26510570 Review.
-
A practical guide to pseudo-marginal methods for computational inference in systems biology.J Theor Biol. 2020 Jul 7;496:110255. doi: 10.1016/j.jtbi.2020.110255. Epub 2020 Mar 26. J Theor Biol. 2020. PMID: 32223995 Review.
References
-
- Andrieu C, Doucet A, Holenstein R. Particle Markov chain Monte Carlo methods. J. R. Stat. Soc. Ser. B. 2010;72(3):269–342.
-
- Barber S, Voss J, Webster M. The rate of convergence for approximate Bayesian computation. Electron. J. Stat. 2015;9(1):80–105.
-
- Beaumont MA, Cornuet J-M, Marin J-M, Robert CP. Adaptive approximate Bayesian computation. Biometrika. 2009;96(4):983–990.
-
- Bernardo JM, Smith AFM. Bayesian Theory. Hoboken: Wiley; 1994.
LinkOut - more resources
Full Text Sources