Plasmodium falciparum infection prevalence among children aged 6-59 months from independent DHS and HIV surveys: Nigeria, 2018
- PMID: 36737630
- PMCID: PMC9898257
- DOI: 10.1038/s41598-023-28257-0
Plasmodium falciparum infection prevalence among children aged 6-59 months from independent DHS and HIV surveys: Nigeria, 2018
Abstract
Prevalence estimates are critical for malaria programming efforts but generating these from non-malaria surveys is not standard practice. Malaria prevalence estimates for 6-59-month-old Nigerian children were compared between two national household surveys performed simultaneously in 2018: a Demographic and Health Survey (DHS) and the Nigeria HIV/AIDS Indicator and Impact Survey (NAIIS). DHS tested via microscopy (n = 8298) and HRP2-based rapid diagnostic test (RDT, n = 11,351), and NAIIS collected dried blood spots (DBS) which were later tested for histidine-rich protein 2 (HRP2) antigen (n = 8029). National Plasmodium falciparum prevalence was 22.6% (95% CI 21.2- 24.1%) via microscopy and 36.2% (34.6- 37.8%) via RDT according to DHS, and HRP2 antigenemia was 38.3% (36.7-39.9%) by NAIIS DBS. Between the two surveys, significant rank-order correlation occurred for state-level malaria prevalence for RDT (Rho = 0.80, p < 0.001) and microscopy (Rho = 0.75, p < 0.001) versus HRP2. RDT versus HRP2 positivity showed 24 states (64.9%) with overlapping 95% confidence intervals from the two independent surveys. P. falciparum prevalence estimates among 6-59-month-olds in Nigeria were highly concordant from two simultaneous, independently conducted household surveys, regardless of malaria test utilized. This provides evidence for the value of post-hoc laboratory HRP2 detection to leverage non-malaria surveys with similar sampling designs to obtain accurate P. falciparum estimates.
© 2023. This is a U.S. Government work and not under copyright protection in the US; foreign copyright protection may apply.
Conflict of interest statement
The authors declare no competing interests.
Figures
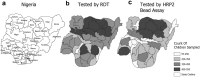
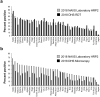
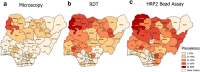
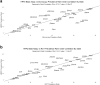
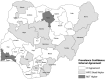
Similar articles
-
Malaria surveys using rapid diagnostic tests and validation of results using post hoc quantification of Plasmodium falciparum histidine-rich protein 2.Malar J. 2017 Nov 7;16(1):451. doi: 10.1186/s12936-017-2101-8. Malar J. 2017. PMID: 29115966 Free PMC article.
-
Improving the Specificity of Plasmodium falciparum Malaria Diagnosis in High-Transmission Settings with a Two-Step Rapid Diagnostic Test and Microscopy Algorithm.J Clin Microbiol. 2017 May;55(5):1540-1549. doi: 10.1128/JCM.00130-17. Epub 2017 Mar 8. J Clin Microbiol. 2017. PMID: 28275077 Free PMC article.
-
Investigation of Plasmodium falciparum pfhrp2 and pfhrp3 gene deletions and performance of a rapid diagnostic test for identifying asymptomatic malaria infection in northern Ethiopia, 2015.Malar J. 2022 Mar 4;21(1):70. doi: 10.1186/s12936-022-04097-7. Malar J. 2022. PMID: 35246151 Free PMC article.
-
Interpreting rapid diagnostic test (RDT) for Plasmodium falciparum.BMC Res Notes. 2018 Dec 4;11(1):850. doi: 10.1186/s13104-018-3967-4. BMC Res Notes. 2018. PMID: 30509313 Free PMC article. Review.
-
Screening strategies and laboratory assays to support Plasmodium falciparum histidine-rich protein deletion surveillance: where we are and what is needed.Malar J. 2022 Jun 24;21(1):201. doi: 10.1186/s12936-022-04226-2. Malar J. 2022. PMID: 35751070 Free PMC article. Review.
Cited by
-
Malaria prevalence and associated population and ecological risk factors among women and children under 5 years in Rwanda.Heliyon. 2024 Jul 14;10(14):e34574. doi: 10.1016/j.heliyon.2024.e34574. eCollection 2024 Jul 30. Heliyon. 2024. PMID: 39130444 Free PMC article.
-
GIS-based spatiotemporal mapping of malaria prevalence and exploration of environmental inequalities.Parasitol Res. 2024 Jul 6;123(7):262. doi: 10.1007/s00436-024-08276-0. Parasitol Res. 2024. PMID: 38970660 Free PMC article.
References
-
- Diboulo E, et al. Bayesian variable selection in modelling geographical heterogeneity in malaria transmission from sparse data: An application to Nouna Health and Demographic Surveillance System (HDSS) data, Burkina Faso. Parasit. Vectors. 2015;8:118. doi: 10.1186/s13071-015-0679-7. - DOI - PMC - PubMed
Publication types
MeSH terms
Substances
LinkOut - more resources
Full Text Sources
Medical