Jasmine and Iris: population-scale structural variant comparison and analysis
- PMID: 36658279
- PMCID: PMC10006329
- DOI: 10.1038/s41592-022-01753-3
Jasmine and Iris: population-scale structural variant comparison and analysis
Abstract
The availability of long reads is revolutionizing studies of structural variants (SVs). However, because SVs vary across individuals and are discovered through imprecise read technologies and methods, they can be difficult to compare. Addressing this, we present Jasmine and Iris ( https://github.com/mkirsche/Jasmine/ ), for fast and accurate SV refinement, comparison and population analysis. Using an SV proximity graph, Jasmine outperforms six widely used comparison methods, including reducing the rate of Mendelian discordance in trio datasets by more than fivefold, and reveals a set of high-confidence de novo SVs confirmed by multiple technologies. We also present a unified callset of 122,813 SVs and 82,379 indels from 31 samples of diverse ancestry sequenced with long reads. We genotype these variants in 1,317 samples from the 1000 Genomes Project and the Genotype-Tissue Expression project with DNA and RNA-sequencing data and assess their widespread impact on gene expression, including within medically relevant genes.
© 2023. The Author(s), under exclusive licence to Springer Nature America, Inc.
Conflict of interest statement
Competing Interests
S.A. has become an employee at Oxford Nanopore. R.S. has become an employee at Illumina.
Figures
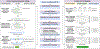
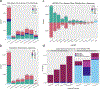
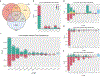
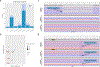
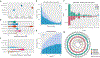
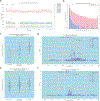
Similar articles
-
SVJedi: genotyping structural variations with long reads.Bioinformatics. 2020 Nov 1;36(17):4568-4575. doi: 10.1093/bioinformatics/btaa527. Bioinformatics. 2020. PMID: 32437523
-
GGTyper: genotyping complex structural variants using short-read sequencing data.Bioinformatics. 2024 Sep 1;40(Suppl 2):ii11-ii19. doi: 10.1093/bioinformatics/btae391. Bioinformatics. 2024. PMID: 39230689 Free PMC article.
-
Comparison and benchmark of structural variants detected from long read and long-read assembly.Brief Bioinform. 2023 Jul 20;24(4):bbad188. doi: 10.1093/bib/bbad188. Brief Bioinform. 2023. PMID: 37200087
-
Haplotyping-Assisted Diploid Assembly and Variant Detection with Linked Reads.Methods Mol Biol. 2023;2590:161-182. doi: 10.1007/978-1-0716-2819-5_11. Methods Mol Biol. 2023. PMID: 36335499 Review.
-
A Practical Guide for Structural Variation Detection in the Human Genome.Curr Protoc Hum Genet. 2020 Sep;107(1):e103. doi: 10.1002/cphg.103. Curr Protoc Hum Genet. 2020. PMID: 32813322 Free PMC article. Review.
Cited by
-
Cytochrome P450 CitCYP97B modulates carotenoid accumulation diversity by hydroxylating β-cryptoxanthin in Citrus.Plant Commun. 2024 Jun 10;5(6):100847. doi: 10.1016/j.xplc.2024.100847. Epub 2024 Feb 19. Plant Commun. 2024. PMID: 38379285 Free PMC article.
-
Truvari: refined structural variant comparison preserves allelic diversity.Genome Biol. 2022 Dec 27;23(1):271. doi: 10.1186/s13059-022-02840-6. Genome Biol. 2022. PMID: 36575487 Free PMC article.
-
A graph clustering algorithm for detection and genotyping of structural variants from long reads.Gigascience. 2024 Jan 2;13:giad112. doi: 10.1093/gigascience/giad112. Gigascience. 2024. PMID: 38206589 Free PMC article.
-
The landscape of genomic structural variation in Indigenous Australians.Nature. 2023 Dec;624(7992):602-610. doi: 10.1038/s41586-023-06842-7. Epub 2023 Dec 13. Nature. 2023. PMID: 38093003 Free PMC article.
-
Graph construction method impacts variation representation and analyses in a bovine super-pangenome.Genome Biol. 2023 May 22;24(1):124. doi: 10.1186/s13059-023-02969-y. Genome Biol. 2023. PMID: 37217946 Free PMC article.
References
Publication types
MeSH terms
Grants and funding
LinkOut - more resources
Full Text Sources