Effectiveness of Semi-Supervised Active Learning in Automated Wound Image Segmentation
- PMID: 36614147
- PMCID: PMC9821322
- DOI: 10.3390/ijms24010706
Effectiveness of Semi-Supervised Active Learning in Automated Wound Image Segmentation
Abstract
Appropriate wound management shortens the healing times and reduces the management costs, benefiting the patient in physical terms and potentially reducing the healthcare system's economic burden. Among the instrumental measurement methods, the image analysis of a wound area is becoming one of the cornerstones of chronic ulcer management. Our study aim is to develop a solid AI method based on a convolutional neural network to segment the wounds efficiently to make the work of the physician more efficient, and subsequently, to lay the foundations for the further development of more in-depth analyses of ulcer characteristics. In this work, we introduce a fully automated model for identifying and segmenting wound areas which can completely automatize the clinical wound severity assessment starting from images acquired from smartphones. This method is based on an active semi-supervised learning training of a convolutional neural network model. In our work, we tested the robustness of our method against a wide range of natural images acquired in different light conditions and image expositions. We collected the images using an ad hoc developed app and saved them in a database which we then used for AI training. We then tested different CNN architectures to develop a balanced model, which we finally validated with a public dataset. We used a dataset of images acquired during clinical practice and built an annotated wound image dataset consisting of 1564 ulcer images from 474 patients. Only a small part of this large amount of data was manually annotated by experts (ground truth). A multi-step, active, semi-supervised training procedure was applied to improve the segmentation performances of the model. The developed training strategy mimics a continuous learning approach and provides a viable alternative for further medical applications. We tested the efficiency of our model against other public datasets, proving its robustness. The efficiency of the transfer learning showed that after less than 50 epochs, the model achieved a stable DSC that was greater than 0.95. The proposed active semi-supervised learning strategy could allow us to obtain an efficient segmentation method, thereby facilitating the work of the clinician by reducing their working times to achieve the measurements. Finally, the robustness of our pipeline confirms its possible usage in clinical practice as a reliable decision support system for clinicians.
Keywords: computer-aided diagnosis; deep learning; image analysis; image segmentation; wound healing.
Conflict of interest statement
The authors declare no conflict of interest.
Figures
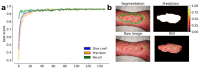
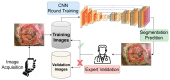
Similar articles
-
Semi-supervised abdominal multi-organ segmentation by object-redrawing.Med Phys. 2024 Nov;51(11):8334-8347. doi: 10.1002/mp.17364. Epub 2024 Aug 21. Med Phys. 2024. PMID: 39167059
-
Learning fuzzy clustering for SPECT/CT segmentation via convolutional neural networks.Med Phys. 2021 Jul;48(7):3860-3877. doi: 10.1002/mp.14903. Epub 2021 May 28. Med Phys. 2021. PMID: 33905560 Free PMC article.
-
Semi-supervised learning for automatic segmentation of the knee from MRI with convolutional neural networks.Comput Methods Programs Biomed. 2020 Jun;189:105328. doi: 10.1016/j.cmpb.2020.105328. Epub 2020 Jan 11. Comput Methods Programs Biomed. 2020. PMID: 31958580
-
Weakly Supervised Learning using Attention gates for colon cancer histopathological image segmentation.Artif Intell Med. 2022 Nov;133:102407. doi: 10.1016/j.artmed.2022.102407. Epub 2022 Sep 24. Artif Intell Med. 2022. PMID: 36328667 Review.
-
Not-so-supervised: A survey of semi-supervised, multi-instance, and transfer learning in medical image analysis.Med Image Anal. 2019 May;54:280-296. doi: 10.1016/j.media.2019.03.009. Epub 2019 Mar 29. Med Image Anal. 2019. PMID: 30959445 Review.
Cited by
-
Automated Wound Image Segmentation: Transfer Learning from Human to Pet via Active Semi-Supervised Learning.Animals (Basel). 2023 Mar 7;13(6):956. doi: 10.3390/ani13060956. Animals (Basel). 2023. PMID: 36978498 Free PMC article.
-
Laser in the Treatment of Atonic Wounds.Biomedicines. 2023 Jun 24;11(7):1815. doi: 10.3390/biomedicines11071815. Biomedicines. 2023. PMID: 37509454 Free PMC article.
-
Non-invasive techniques for wound assessment: A comprehensive review.Int Wound J. 2024 Nov;21(11):e70109. doi: 10.1111/iwj.70109. Int Wound J. 2024. PMID: 39567223 Free PMC article. Review.
-
Automated Prediction of Photographic Wound Assessment Tool in Chronic Wound Images.J Med Syst. 2024 Jan 16;48(1):14. doi: 10.1007/s10916-023-02029-9. J Med Syst. 2024. PMID: 38227131 Free PMC article.
-
ETU-Net: efficient Transformer and convolutional U-style connected attention segmentation network applied to endoscopic image of epistaxis.Front Med (Lausanne). 2023 Aug 9;10:1198054. doi: 10.3389/fmed.2023.1198054. eCollection 2023. Front Med (Lausanne). 2023. PMID: 37636575 Free PMC article.
References
-
- Gethin G. The importance of continuous wound measuring. Wounds UK. 2006;2:60–68.
MeSH terms
Grants and funding
LinkOut - more resources
Full Text Sources