DRPreter: Interpretable Anticancer Drug Response Prediction Using Knowledge-Guided Graph Neural Networks and Transformer
- PMID: 36430395
- PMCID: PMC9699175
- DOI: 10.3390/ijms232213919
DRPreter: Interpretable Anticancer Drug Response Prediction Using Knowledge-Guided Graph Neural Networks and Transformer
Abstract
Some of the recent studies on drug sensitivity prediction have applied graph neural networks to leverage prior knowledge on the drug structure or gene network, and other studies have focused on the interpretability of the model to delineate the mechanism governing the drug response. However, it is crucial to make a prediction model that is both knowledge-guided and interpretable, so that the prediction accuracy is improved and practical use of the model can be enhanced. We propose an interpretable model called DRPreter (drug response predictor and interpreter) that predicts the anticancer drug response. DRPreter learns cell line and drug information with graph neural networks; the cell-line graph is further divided into multiple subgraphs with domain knowledge on biological pathways. A type-aware transformer in DRPreter helps detect relationships between pathways and a drug, highlighting important pathways that are involved in the drug response. Extensive experiments on the GDSC (Genomics of Drug Sensitivity and Cancer) dataset demonstrate that the proposed method outperforms state-of-the-art graph-based models for drug response prediction. In addition, DRPreter detected putative key genes and pathways for specific drug-cell-line pairs with supporting evidence in the literature, implying that our model can help interpret the mechanism of action of the drug.
Keywords: Explainable AI; artificial intelligence; cancer; drug discovery; drug sensitivity; graph neural networks; human health; pharmacogenomics; precision medicine; transcriptomics.
Conflict of interest statement
The authors declare no conflict of interest.
Figures
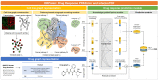
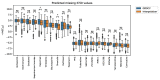
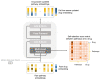
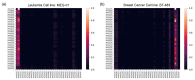
Similar articles
-
GPDRP: a multimodal framework for drug response prediction with graph transformer.BMC Bioinformatics. 2023 Dec 17;24(1):484. doi: 10.1186/s12859-023-05618-0. BMC Bioinformatics. 2023. PMID: 38105227 Free PMC article.
-
Prediction of anticancer drug sensitivity using an interpretable model guided by deep learning.BMC Bioinformatics. 2024 May 9;25(1):182. doi: 10.1186/s12859-024-05669-x. BMC Bioinformatics. 2024. PMID: 38724920 Free PMC article.
-
Toward Explainable Anticancer Compound Sensitivity Prediction via Multimodal Attention-Based Convolutional Encoders.Mol Pharm. 2019 Dec 2;16(12):4797-4806. doi: 10.1021/acs.molpharmaceut.9b00520. Epub 2019 Oct 31. Mol Pharm. 2019. PMID: 31618586
-
Graph Artificial Intelligence in Medicine.Annu Rev Biomed Data Sci. 2024 Aug;7(1):345-368. doi: 10.1146/annurev-biodatasci-110723-024625. Epub 2024 Jul 24. Annu Rev Biomed Data Sci. 2024. PMID: 38749465 Free PMC article. Review.
-
Comprehensive evaluation of deep and graph learning on drug-drug interactions prediction.Brief Bioinform. 2023 Jul 20;24(4):bbad235. doi: 10.1093/bib/bbad235. Brief Bioinform. 2023. PMID: 37401373 Review.
Cited by
-
TransCDR: a deep learning model for enhancing the generalizability of drug activity prediction through transfer learning and multimodal data fusion.BMC Biol. 2024 Oct 9;22(1):227. doi: 10.1186/s12915-024-02023-8. BMC Biol. 2024. PMID: 39385185 Free PMC article.
-
Transfer learning of condition-specific perturbation in gene interactions improves drug response prediction.Bioinformatics. 2024 Jun 28;40(Suppl 1):i130-i139. doi: 10.1093/bioinformatics/btae249. Bioinformatics. 2024. PMID: 38940127 Free PMC article.
-
Attention is all you need: utilizing attention in AI-enabled drug discovery.Brief Bioinform. 2023 Nov 22;25(1):bbad467. doi: 10.1093/bib/bbad467. Brief Bioinform. 2023. PMID: 38189543 Free PMC article. Review.
-
Graph Neural Networks in Cancer and Oncology Research: Emerging and Future Trends.Cancers (Basel). 2023 Dec 15;15(24):5858. doi: 10.3390/cancers15245858. Cancers (Basel). 2023. PMID: 38136405 Free PMC article. Review.
-
A granularity-level information fusion strategy on hypergraph transformer for predicting synergistic effects of anticancer drugs.Brief Bioinform. 2023 Nov 22;25(1):bbad522. doi: 10.1093/bib/bbad522. Brief Bioinform. 2023. PMID: 38243692 Free PMC article.
References
-
- Singh D.B. The impact of pharmacogenomics in personalized medicine. Curr. Appl. Pharm. Biotechnol. 2019;171:369–394. - PubMed
MeSH terms
Substances
Grants and funding
- NRF-2022M3E5F3085677/Bio Medical Technology Development Program of the National Research Foundation (NRF) funded by the Ministry of Science ICT
- No. DY0002259501/Ministry of food and Drug Safety
- NO.2021-0-01343, Artificial Intelligence Graduate School Program (Seoul National University)/Institute of Information communications Technology Planning Evaluation (IITP) grant funded by the Korea government(MSIT)
- No. NRF-2020R1G1A1003558/National Research Foundation of Korea(NRF) grant funded by the Korea government(MSIT)
- NRF2014M3C9A3063541/Collaborative Genome Program for Fostering New Post Genome Industry of the National Research Foundation (NRF) funded by the Ministry of Science and ICT (MSIT)
LinkOut - more resources
Full Text Sources
Other Literature Sources