Bioinformatics and systems biology approaches to identify molecular targeting mechanism influenced by COVID-19 on heart failure
- PMID: 36420258
- PMCID: PMC9678344
- DOI: 10.3389/fimmu.2022.1052850
Bioinformatics and systems biology approaches to identify molecular targeting mechanism influenced by COVID-19 on heart failure
Abstract
Coronavirus disease 2019 (COVID-19) caused by the severe acute respiratory syndrome coronavirus 2 (SARS-CoV-2) has emerged as a contemporary hazard to people. It has been known that COVID-19 can both induce heart failure (HF) and raise the risk of patient mortality. However, the mechanism underlying the association between COVID-19 and HF remains unclear. The common molecular pathways between COVID-19 and HF were identified using bioinformatic and systems biology techniques. Transcriptome analysis was performed to identify differentially expressed genes (DEGs). To identify gene ontology terms and Kyoto Encyclopedia of Genes and Genomes pathways, common DEGs were used for enrichment analysis. The results showed that COVID-19 and HF have several common immune mechanisms, including differentiation of T helper (Th) 1, Th 2, Th 17 cells; activation of lymphocytes; and binding of major histocompatibility complex class I and II protein complexes. Furthermore, a protein-protein interaction network was constructed to identify hub genes, and immune cell infiltration analysis was performed. Six hub genes (FCGR3A, CD69, IFNG, CCR7, CCL5, and CCL4) were closely associated with COVID-19 and HF. These targets were associated with immune cells (central memory CD8 T cells, T follicular helper cells, regulatory T cells, myeloid-derived suppressor cells, plasmacytoid dendritic cells, macrophages, eosinophils, and neutrophils). Additionally, transcription factors, microRNAs, drugs, and chemicals that are closely associated with COVID-19 and HF were identified through the interaction network.
Keywords: COVID-19; bioinformatics analysis; heart failure; immunology; systems biology approaches.
Copyright © 2022 Yang, Liu, Gong, Li and Liu.
Conflict of interest statement
The authors declare that the research was conducted in the absence of any commercial or financial relationships that could be construed as a potential conflict of interest.
Figures
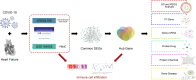
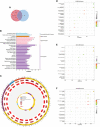
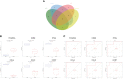
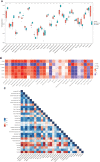
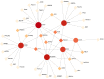
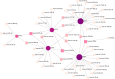
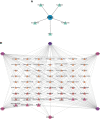
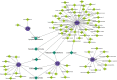
Similar articles
-
Investigation of the relationship between COVID-19 and pancreatic cancer using bioinformatics and systems biology approaches.Medicine (Baltimore). 2024 Aug 2;103(31):e39057. doi: 10.1097/MD.0000000000039057. Medicine (Baltimore). 2024. PMID: 39093763 Free PMC article.
-
Uncovering the genetic links of SARS-CoV-2 infections on heart failure co-morbidity by a systems biology approach.ESC Heart Fail. 2022 Oct;9(5):2937-2954. doi: 10.1002/ehf2.14003. Epub 2022 Jun 21. ESC Heart Fail. 2022. PMID: 35727093 Free PMC article.
-
Identifying immune cell infiltration and diagnostic biomarkers in heart failure and osteoarthritis by bioinformatics analysis.Medicine (Baltimore). 2023 Jun 30;102(26):e34166. doi: 10.1097/MD.0000000000034166. Medicine (Baltimore). 2023. PMID: 37390254 Free PMC article.
-
STAT4 and COL1A2 are potential diagnostic biomarkers and therapeutic targets for heart failure comorbided with depression.Brain Res Bull. 2022 Jun 15;184:68-75. doi: 10.1016/j.brainresbull.2022.03.014. Epub 2022 Mar 31. Brain Res Bull. 2022. PMID: 35367598 Review.
-
Bioinformatics and systems biology approaches to identify the effects of COVID-19 on neurodegenerative diseases: A review.Medicine (Baltimore). 2022 Dec 9;101(49):e32100. doi: 10.1097/MD.0000000000032100. Medicine (Baltimore). 2022. PMID: 36626425 Free PMC article. Review.
Cited by
-
Immunogenicity and reactogenicity of inactivated SARS-CoV-2 vaccines in healthy adults.Front Immunol. 2023 Jul 25;14:1152899. doi: 10.3389/fimmu.2023.1152899. eCollection 2023. Front Immunol. 2023. PMID: 37559719 Free PMC article.
References
-
- Vasbinder A, Meloche C, Azam TU, Anderson E, Catalan T, Shadid H, et al. . Relationship between preexisting cardiovascular disease and death and cardiovascular outcomes in critically ill patients with COVID-19. Circ Cardiovasc Qual Outcomes (2022) 15(10):e008942. doi: 10.1161/CIRCOUTCOMES.122.008942 - DOI - PMC - PubMed
Publication types
MeSH terms
LinkOut - more resources
Full Text Sources
Medical
Research Materials
Miscellaneous