Dosing time optimization of antihypertensive medications by including the circadian rhythm in pharmacokinetic-pharmacodynamic models
- PMID: 36374862
- PMCID: PMC9704759
- DOI: 10.1371/journal.pcbi.1010711
Dosing time optimization of antihypertensive medications by including the circadian rhythm in pharmacokinetic-pharmacodynamic models
Abstract
Blood pressure (BP) follows a circadian variation, increasing during active hours, showing a small postprandial valley and a deeper decrease during sleep. Nighttime reduction of 10-20% relative to daytime BP is defined as a dipper pattern, and a reduction of less than 10%, as a non-dipper pattern. Despite this BP variability, hypertension's diagnostic criteria and therapeutic objectives are usually based on BP average values. Indeed, studies have shown that chrono-pharmacological optimization significantly reduces long-term cardiovascular risk if a BP dipper pattern is maintained. Changes in the effect of antihypertensive medications can be explained by circadian variations in their pharmacokinetics (PK) and pharmacodynamics (PD). Nevertheless, BP circadian variation has been scarcely included in PK-PD models of antihypertensive medications to date. In this work, we developed PK-PD models that include circadian rhythm to find the optimal dosing time (Ta) of first-line antihypertensive medications for dipper and non-dipper patterns. The parameters of the PK-PD models were estimated using global optimization, and models were selected according to the lowest corrected Akaike information criterion value. Simultaneously, sensitivity and identifiability analysis were performed to determine the relevance of the parameters and establish those that can be estimated. Subsequently, Ta parameters were optimized to maximize the effect on BP average, BP peaks, and sleep-time dip. As a result, all selected models included at least one circadian PK component, and circadian parameters had the highest sensitivity. Furthermore, Ta with which BP>130/80 mmHg and a dip of 10-20% are achieved were proposed when possible. We show that the optimal Ta depends on the therapeutic objective, the medication, and the BP profile. Therefore, our results suggest making chrono-pharmacological recommendations in a personalized way.
Copyright: © 2022 Cortés-Ríos et al. This is an open access article distributed under the terms of the Creative Commons Attribution License, which permits unrestricted use, distribution, and reproduction in any medium, provided the original author and source are credited.
Conflict of interest statement
The authors have declared that no competing interests exist.
Figures
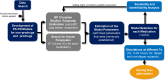
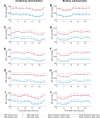
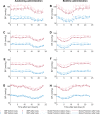
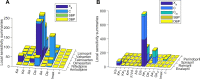
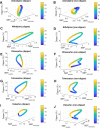
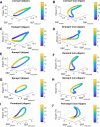
Similar articles
-
Ambulatory blood pressure monitoring in the prediction of cardiovascular events and effects of chronotherapy: rationale and design of the MAPEC study.Chronobiol Int. 2007;24(4):749-75. doi: 10.1080/07420520701535837. Chronobiol Int. 2007. PMID: 17701685 Clinical Trial.
-
Blunted sleep-time relative blood pressure decline increases cardiovascular risk independent of blood pressure level--the "normotensive non-dipper" paradox.Chronobiol Int. 2013 Mar;30(1-2):87-98. doi: 10.3109/07420528.2012.701127. Epub 2012 Oct 5. Chronobiol Int. 2013. PMID: 23039824 Clinical Trial.
-
Perspectives on the chronotherapy of hypertension based on the results of the MAPEC study.Chronobiol Int. 2010 Sep;27(8):1652-67. doi: 10.3109/07420528.2010.510788. Chronobiol Int. 2010. PMID: 20854140
-
Circadian variation of blood pressure: the basis for the chronotherapy of hypertension.Adv Drug Deliv Rev. 2007 Aug 31;59(9-10):904-22. doi: 10.1016/j.addr.2006.08.003. Epub 2007 Jun 27. Adv Drug Deliv Rev. 2007. PMID: 17659807 Review.
-
[Dipper, non-dipper pattern].Nihon Rinsho. 2014 Aug;72(8):1400-3. Nihon Rinsho. 2014. PMID: 25167742 Review. Japanese.
Cited by
-
Understanding the dosing-time-dependent antihypertensive effect of valsartan and aspirin through mathematical modeling.Front Endocrinol (Lausanne). 2023 Mar 8;14:1110459. doi: 10.3389/fendo.2023.1110459. eCollection 2023. Front Endocrinol (Lausanne). 2023. PMID: 36967780 Free PMC article.
References
-
- Salles GF, Reboldi G, Fagard RH, Cardoso CRL, Pierdomenico SD, Verdecchia P, et al.. Prognostic Effect of the Nocturnal Blood Pressure Fall in Hypertensive Patients: The Ambulatory Blood Pressure Collaboration in Patients With Hypertension (ABC-H) Meta-Analysis. Hypertens (Dallas, Tex 1979). 2016;67: 693–700. doi: 10.1161/HYPERTENSIONAHA.115.06981 - DOI - PubMed
Publication types
MeSH terms
Substances
Grants and funding
LinkOut - more resources
Full Text Sources
Medical
Research Materials