Deep transfer learning of cancer drug responses by integrating bulk and single-cell RNA-seq data
- PMID: 36310235
- PMCID: PMC9618578
- DOI: 10.1038/s41467-022-34277-7
Deep transfer learning of cancer drug responses by integrating bulk and single-cell RNA-seq data
Abstract
Drug screening data from massive bulk gene expression databases can be analyzed to determine the optimal clinical application of cancer drugs. The growing amount of single-cell RNA sequencing (scRNA-seq) data also provides insights into improving therapeutic effectiveness by helping to study the heterogeneity of drug responses for cancer cell subpopulations. Developing computational approaches to predict and interpret cancer drug response in single-cell data collected from clinical samples can be very useful. We propose scDEAL, a deep transfer learning framework for cancer drug response prediction at the single-cell level by integrating large-scale bulk cell-line data. The highlight in scDEAL involves harmonizing drug-related bulk RNA-seq data with scRNA-seq data and transferring the model trained on bulk RNA-seq data to predict drug responses in scRNA-seq. Another feature of scDEAL is the integrated gradient feature interpretation to infer the signature genes of drug resistance mechanisms. We benchmark scDEAL on six scRNA-seq datasets and demonstrate its model interpretability via three case studies focusing on drug response label prediction, gene signature identification, and pseudotime analysis. We believe that scDEAL could help study cell reprogramming, drug selection, and repurposing for improving therapeutic efficacy.
© 2022. The Author(s).
Conflict of interest statement
The authors declare no competing interests.
Figures
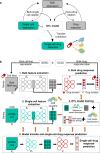
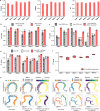
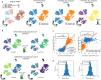
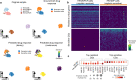
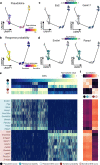
Similar articles
-
Exploring the advances of single-cell RNA sequencing in thyroid cancer: a narrative review.Med Oncol. 2023 Dec 21;41(1):27. doi: 10.1007/s12032-023-02260-x. Med Oncol. 2023. PMID: 38129369 Free PMC article. Review.
-
Improving bulk RNA-seq classification by transferring gene signature from single cells in acute myeloid leukemia.Brief Bioinform. 2022 Mar 10;23(2):bbac002. doi: 10.1093/bib/bbac002. Brief Bioinform. 2022. PMID: 35136933
-
scDR: Predicting Drug Response at Single-Cell Resolution.Genes (Basel). 2023 Jan 19;14(2):268. doi: 10.3390/genes14020268. Genes (Basel). 2023. PMID: 36833194 Free PMC article.
-
MuSiC2: cell-type deconvolution for multi-condition bulk RNA-seq data.Brief Bioinform. 2022 Nov 19;23(6):bbac430. doi: 10.1093/bib/bbac430. Brief Bioinform. 2022. PMID: 36208175 Free PMC article.
-
Single-cell RNA sequencing in breast cancer: Understanding tumor heterogeneity and paving roads to individualized therapy.Cancer Commun (Lond). 2020 Aug;40(8):329-344. doi: 10.1002/cac2.12078. Epub 2020 Jul 12. Cancer Commun (Lond). 2020. PMID: 32654419 Free PMC article. Review.
Cited by
-
Exploring the advances of single-cell RNA sequencing in thyroid cancer: a narrative review.Med Oncol. 2023 Dec 21;41(1):27. doi: 10.1007/s12032-023-02260-x. Med Oncol. 2023. PMID: 38129369 Free PMC article. Review.
-
DCRELM: dual correlation reduction network-based extreme learning machine for single-cell RNA-seq data clustering.Sci Rep. 2024 Jun 12;14(1):13541. doi: 10.1038/s41598-024-64217-y. Sci Rep. 2024. PMID: 38866896 Free PMC article.
-
Cell reprogramming design by transfer learning of functional transcriptional networks.Proc Natl Acad Sci U S A. 2024 Mar 12;121(11):e2312942121. doi: 10.1073/pnas.2312942121. Epub 2024 Mar 4. Proc Natl Acad Sci U S A. 2024. PMID: 38437548 Free PMC article.
-
AlphaML: A clear, legible, explainable, transparent, and elucidative binary classification platform for tabular data.Patterns (N Y). 2023 Dec 13;5(1):100897. doi: 10.1016/j.patter.2023.100897. eCollection 2024 Jan 12. Patterns (N Y). 2023. PMID: 38264719 Free PMC article.
-
SpaRx: Elucidate single-cell spatial heterogeneity of drug responses for personalized treatment.bioRxiv [Preprint]. 2023 Aug 6:2023.08.03.551911. doi: 10.1101/2023.08.03.551911. bioRxiv. 2023. Update in: Brief Bioinform. 2023 Sep 22;24(6):bbad338. doi: 10.1093/bib/bbad338 PMID: 37577665 Free PMC article. Updated. Preprint.
References
Publication types
MeSH terms
Substances
Grants and funding
LinkOut - more resources
Full Text Sources
Other Literature Sources